Statistical Selection of Congruent Subspaces for Mining Attributed Graphs.
ICDM(2013)
摘要
Current mining algorithms for attributed graphs exploit dependencies between attribute information and edge structure, referred to as homophily. However, techniques fail if this assumption does not hold for the full attribute space. In multivariate spaces, some attributes have high dependency with the graph structure while others do not show any dependency. Hence, it is important to select congruent subspaces (i.e., subsets of the node attributes) showing dependencies with the graph structure. In this work, we propose a method for the statistical selection of such congruent subspaces. More specifically, we define a measure which assesses the degree of congruence between a set of attributes and the entire graph. We use it as the core of a statistical test, which congruent subspaces must pass. To illustrate its applicability to common graph mining tasks and in order to evaluate our selection scheme, we apply it to community outlier detection. Our selection of congruent subspaces enhances outlier detection by measuring outlier ness scores in selected subspaces only. Experiments on attributed graphs show that our approach outperforms traditional full space approaches and gives way to better outlier detection.
更多查看译文
关键词
data mining,statistical testing,graph theory
AI 理解论文
溯源树
样例
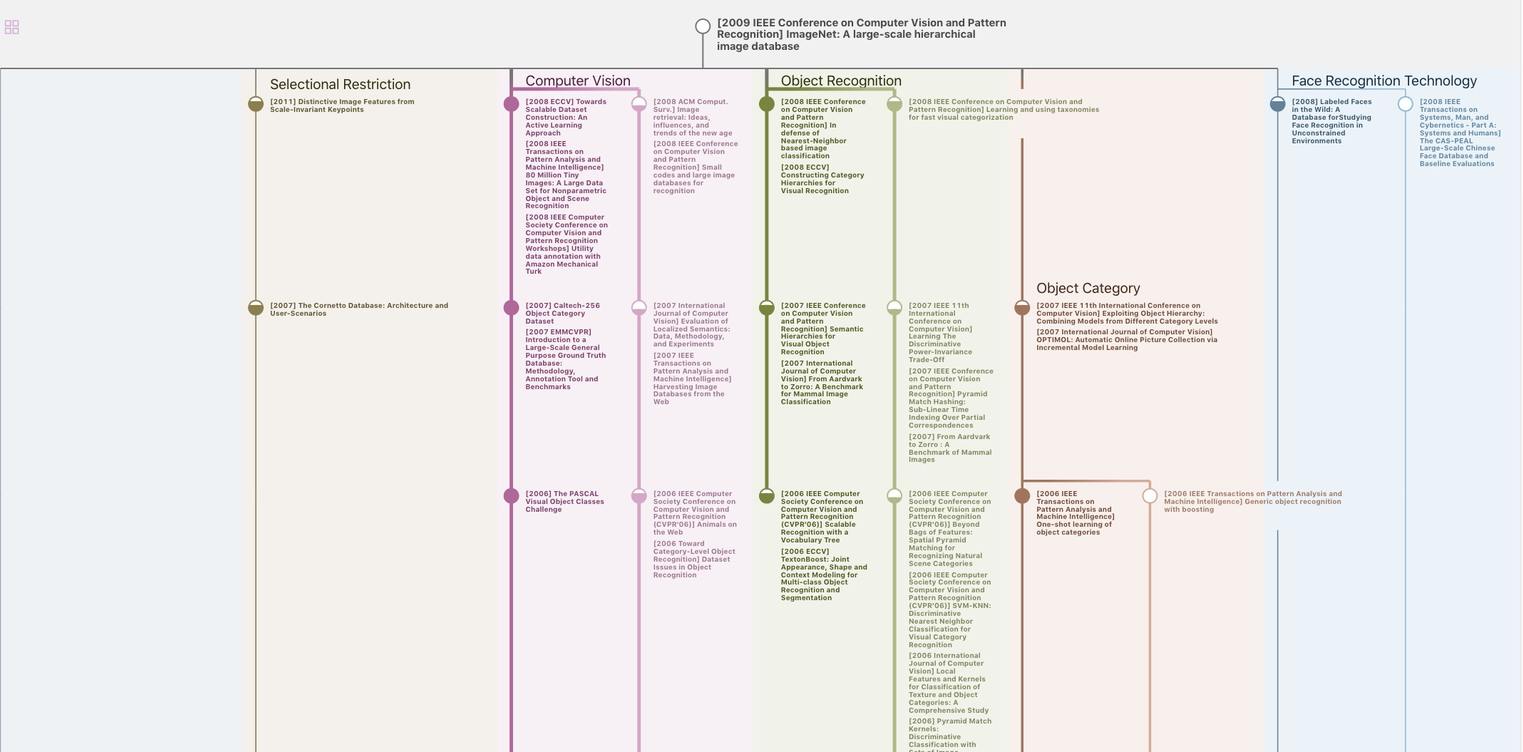
生成溯源树,研究论文发展脉络
Chat Paper
正在生成论文摘要