Revenue optimization with relevance constraint in sponsored search.
KDD(2009)
摘要
ABSTRACTDisplaying sponsored ads alongside the search results is a key monetization strategy for search engine companies. Since users are more likely to click ads that are relevant to their query, it is crucial for search engine to deliver the right ads for the query and the order in which they are displayed. There are several works investigating on how to learn a ranking function to maximize the number of ad clicks. However, this ranking optimization problem is different from algorithmic search results ranking in that the ranking scheme must take received revenue into account in order to make more profit for the search engines. In this paper, we address a new optimization problem and aim to answer the question: how to construct a ranking model that can deliver high quality ads to the user as well as maximize search engine revenue? We introduce a novel tradeoff method from machine learning perspective, and through this method we have the privilege of choosing a tradeoff parameter to achieve highest relevance ranking or highest revenue ranking or the tradeoff between them. The algorithms are built upon the click-through log data with real ad clicks and impressions. The extensively experimental results verify that the proposed algorithm has the property that the search engine could choose a proper parameter to achieve high revenue(income) without losing to much relevance.
更多查看译文
AI 理解论文
溯源树
样例
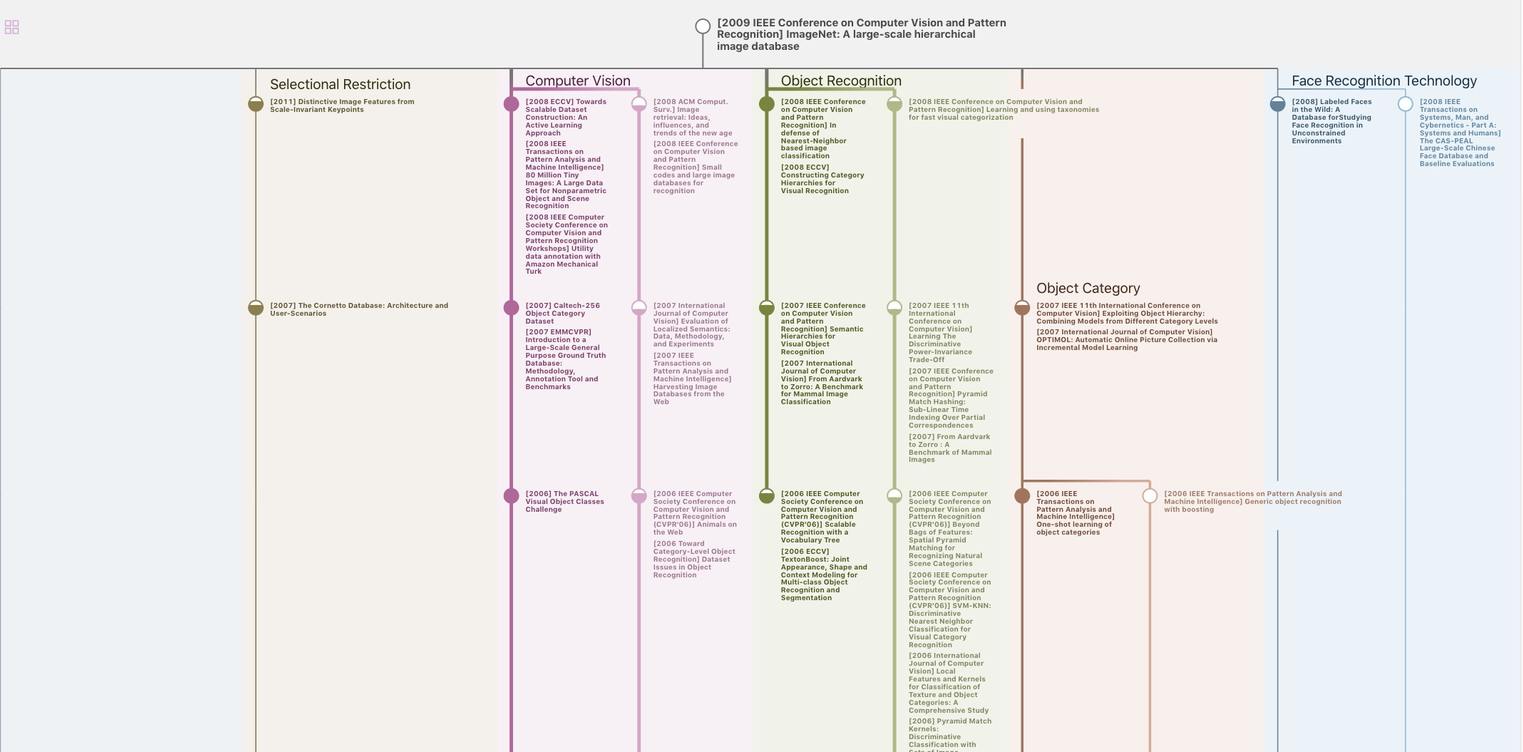
生成溯源树,研究论文发展脉络
Chat Paper
正在生成论文摘要