Cross-Lingual Self-training to Learn Multilingual Representation for Low-Resource Speech Recognition
CIRCUITS SYSTEMS AND SIGNAL PROCESSING(2022)
Abstract
Representation learning or pre-training has shown promising performance for low-resource speech recognition which suffers from the data shortage. Recently, self-supervised methods have achieved surprising performance for speech pre-training by effectively utilizing large amount of un-annotated data. In this paper, we propose a new pre-training framework, Cross-Lingual Self-Training (XLST), to further improve the effectiveness for multilingual representation learning. Specifically, XLST first trains a phoneme classification model with a small amount of annotated data of a non-target language and then uses it to produce initial targets for training another model on multilingual un-annotated data, i.e., maximizing frame-level similarity between the output embeddings of two models. Furthermore, we employ the moving average and multi-view data augmentation mechanisms to better generalize the learned representations. Experimental results on downstream speech recognition tasks for 5 low-resource languages demonstrate the effectiveness of XLST. Specifically, leveraging additional 100 h of annotated English data for pre-training, the proposed XLST achieves a relative 24.8% PER reduction over the state-of-the-art self-supervised methods.
MoreTranslated text
Key words
Multilingual representation learning, Cross-lingual self-training, Low-resource speech recognition, Speech pre-training
AI Read Science
Must-Reading Tree
Example
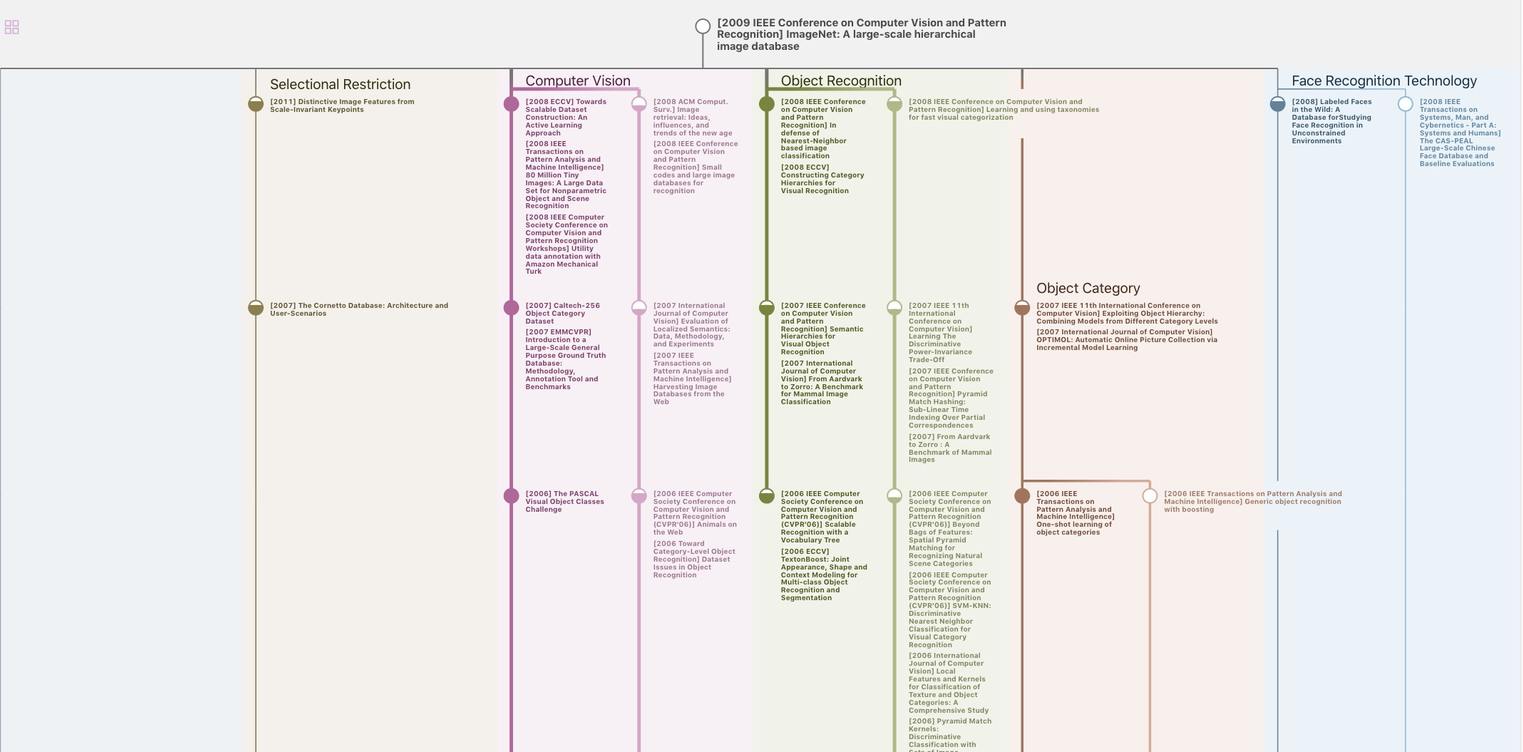
Generate MRT to find the research sequence of this paper
Chat Paper
Summary is being generated by the instructions you defined