Real-time and low power embedded ℓ1-optimization solver design
FPT(2013)
摘要
Basis pursuit denoising (BPDN) is an optimization method used in cutting edge computer vision and compressive sensing research. Although hosting a BPDN solver on an embedded platform is desirable because analysis can be performed in real-time, existing solvers are generally unsuitable for embedded implementation due to either poor run-time performance or high memory usage. To address the aforementioned issues, this paper proposes an embedded-friendly solver which demonstrates superior run-time performance, high recovery accuracy and competitive memory usage compared to existing solvers. For a problem with 5000 variables and 500 constraints, the solver occupies a small memory footprint of 29 kB and takes 0.14 seconds to complete on the Xilinx Zynq Z-7020 system-on-chip. The same problem takes 0.19 seconds on the Intel Core i7-2620M, which runs at 4 times the clock frequency and 114 times the power budget of the Z-7020. Without sacrificing runtime performance, the solver has been highly optimized for power constrained embedded applications. By far this is the first embedded solver capable of handling large scale problems with several thousand variables.
更多查看译文
关键词
embedded platform,basis pursuit denoising,cutting edge computer vision,BPDN solver,circuit optimisation,low-power electronics,image denoising,ℓ1-optimization,Basis pursuit denoising,system-on-chip,Xilinx Zynq Z-7020 system-on-chip,Embedded implementation,storage capacity 14 Kbit,power constrained embedded applications,compressed sensing,time 0.14 s,compressive sensing research,computer vision,low power embedded ℓ1-optimization solver design,time 0.19 s,embedded systems,Intel Core i7-2620M,power budget,real-time systems,LASSO optimization,Xilinx Zynq Z-7020
AI 理解论文
溯源树
样例
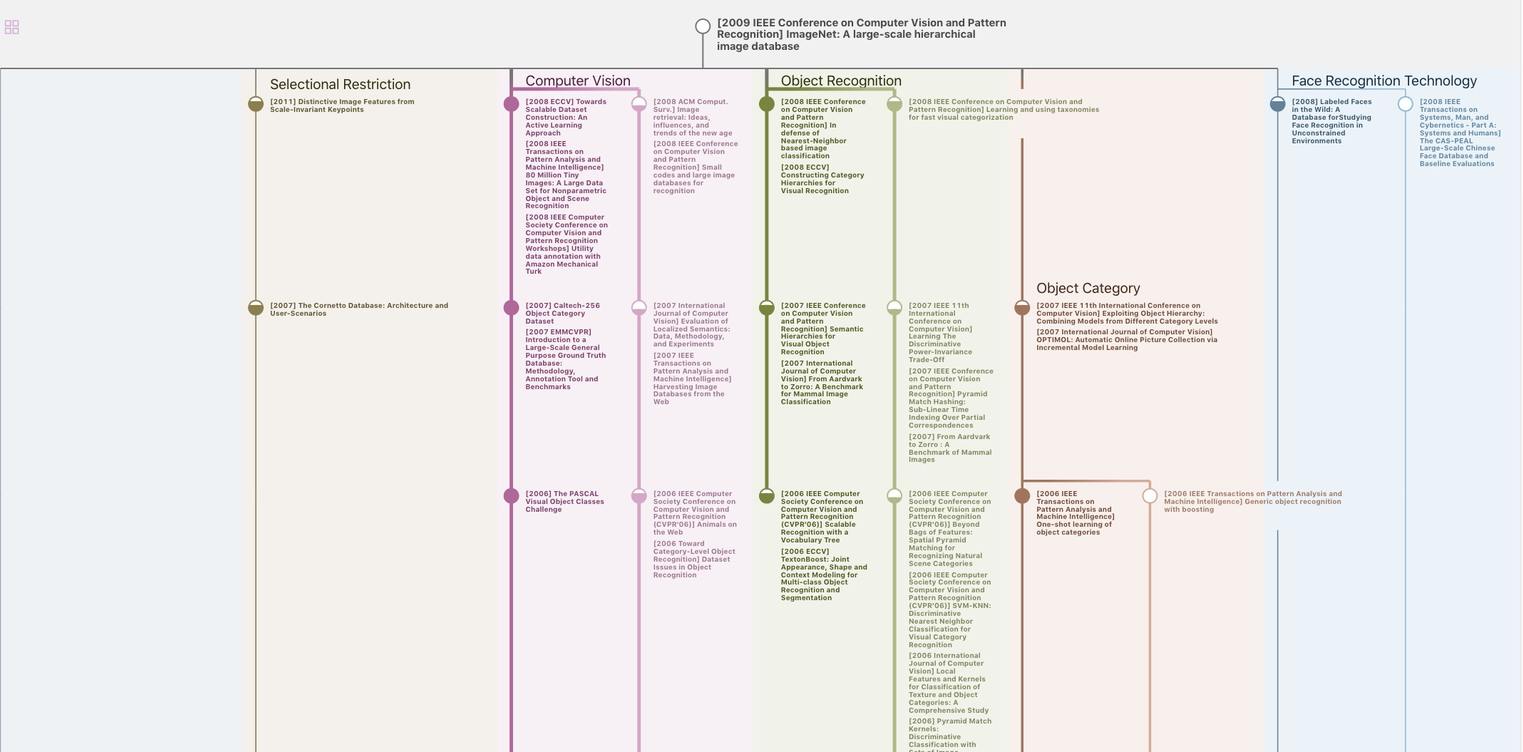
生成溯源树,研究论文发展脉络
Chat Paper
正在生成论文摘要