Neural Architecture Search via Multi-Hashing Embedding and Graph Tensor Networks for Multilingual Text Classification
IEEE TRANSACTIONS ON EMERGING TOPICS IN COMPUTATIONAL INTELLIGENCE(2024)
Abstract
Neural architecture search (NAS) has been demonstrated to be promising in deep learning for text classification. Most existing NAS algorithms, however, are proposed for single-language text classification. They may become ineffective when extended to multilingual text classification because the differences in the syntactic structure and contextual semantics of different languages increase the computational complexity of NAS search. To address the above issue, this article proposes a differential neural architecture search approach using multi-hashing embedding for multilingual text representation. A multi-hashing network capable of processing heterogeneous graph information is constructed so that cross-language syntactic and contextual semantic information can be effectively represented. In addition, a neural tensor network with multi-hashing embedding is adopted as a continuous encoder to estimate the probability for each candidate operation in the search space, and reparameterization-based gradient search is employed to efficiently search for network architectures for multilingual text representation. Our experimental results on two multilingual text classification datasets demonstrate that the proposed approach outperforms the state-of-the-art NAS methods for text classification in terms of both classification accuracy and computational efficiency.
MoreTranslated text
Key words
Gradient-based method,multi-hashing embedding,multilingual text classification,neural architecture search,neural tensor network
AI Read Science
Must-Reading Tree
Example
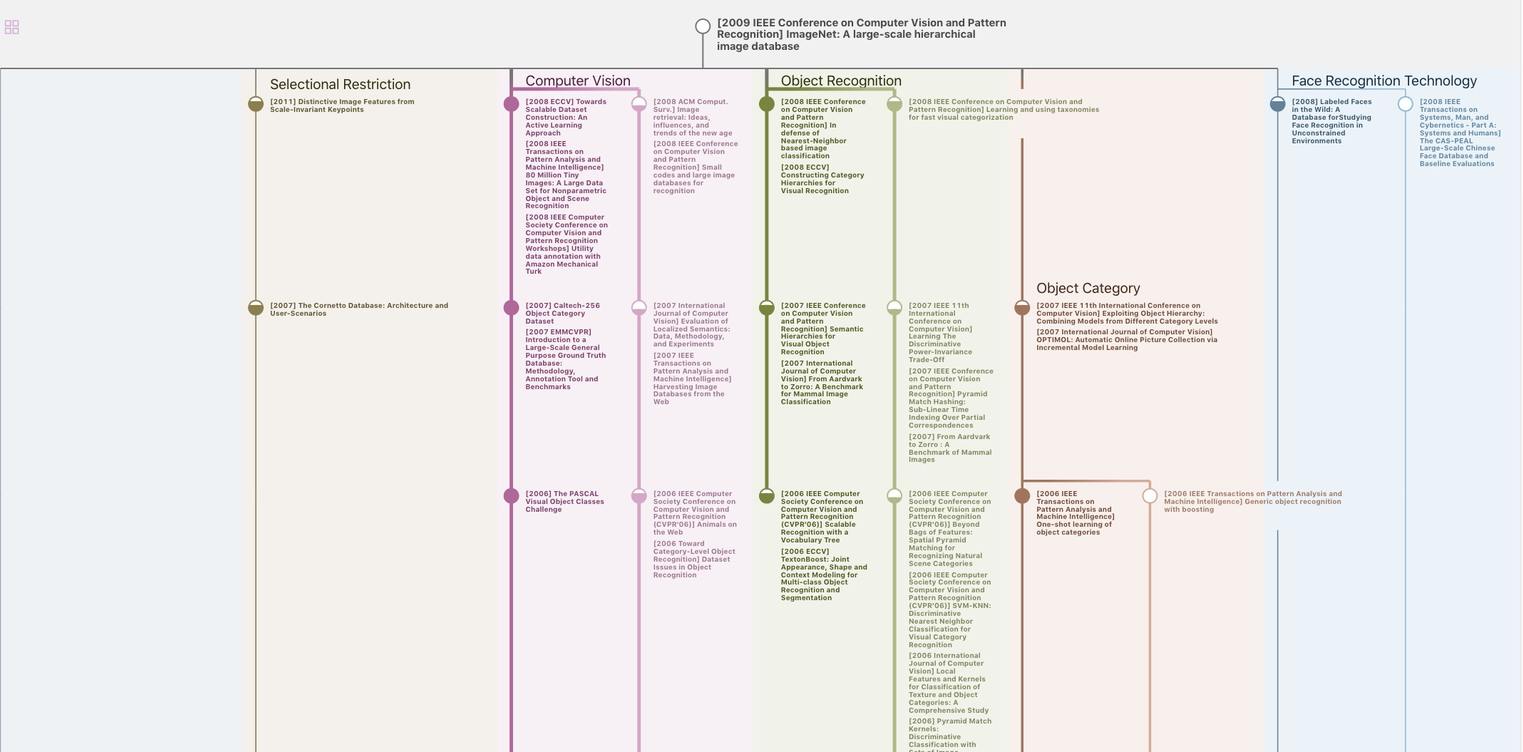
Generate MRT to find the research sequence of this paper
Chat Paper
Summary is being generated by the instructions you defined