Kernel Trick Embedded Gaussian Mixture Model
ALGORITHMIC LEARNING THEORY, PROCEEDINGS(2003)
摘要
In this paper, we present a kernel trick embedded Gaussian Mixture Model (GMM), called kernel GMM. The basic idea is to embed kernel trick into EM algorithm and deduce a parameter estimation algorithm for GMM in feature space. Kernel GMM could be viewed as a Bayesian Kernel Method. Compared with most classical kernel methods, the proposed method can solve problems in probabilistic framework. Moreover, it can tackle nonlinear problems better than the traditional GMM. To avoid great computational cost problem existing in most kernel methods upon large scale data set, we also employ a Monte Carlo sampling technique to speed up kernel GMM so that it is more practical and efficient. Experimental results on synthetic and real-world data set demonstrate that the proposed approach has satisfing performance.
更多查看译文
关键词
monte carlo sampling,feature space,parameter estimation,gaussian mixture model,kernel method,em algorithm
AI 理解论文
溯源树
样例
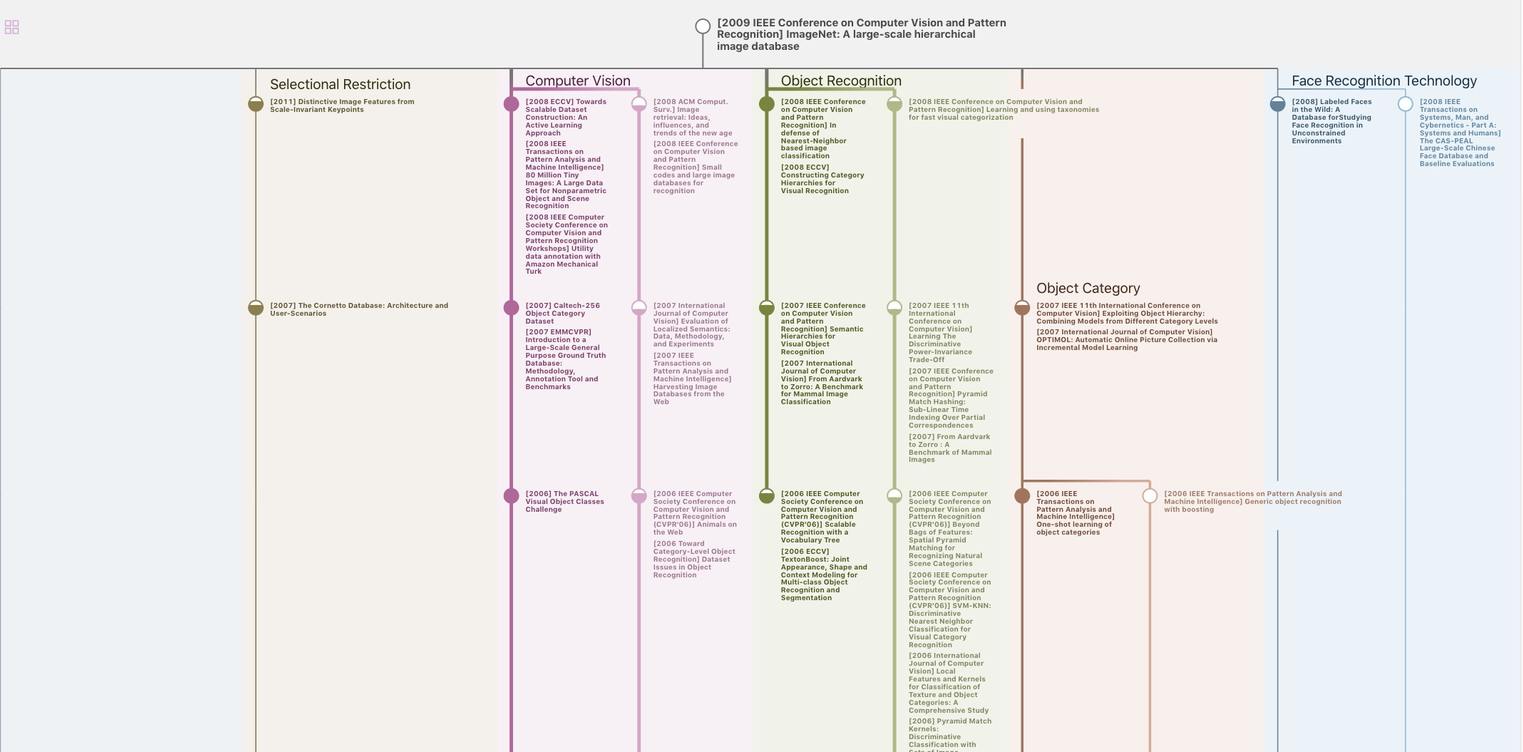
生成溯源树,研究论文发展脉络
Chat Paper
正在生成论文摘要