On the Performance of Online Learning Methods for Detecting Malicious Executables
Machine Learning in Cyber Trust(2009)
Abstract
We present results from an empirical study of seven online-learning methods on the task of detecting previously unseen malicious
executables. Malicious software has disrupted computer and network operation and has compromised or destroyed sensitive information.
Methods of machine learning, which build predictive models that generalize training data, have proven useful for detecting
previously unseen malware. In previous studies, batch methods detected malicious and benign executables with high true-positive
and true-negative rates, but doing so required significant time and space, which may limit applicability. Online methods of
learning can update models quickly with only a single example, but potential trade-offs in performance are not well-understood
for this task. Accuracy of the best performing online methods was 93#x0025;, which was 3-4% lower than that of batch methods.
For applications that require immediate updates of models, this may be an acceptable trade-off. Our study characterizes these
tradeoffs, thereby giving researchers and practitioners insights into the performance of online methods of machine learning
on the task of detecting malicious executables.
MoreTranslated text
Key words
machine learning,prediction model,empirical study
AI Read Science
Must-Reading Tree
Example
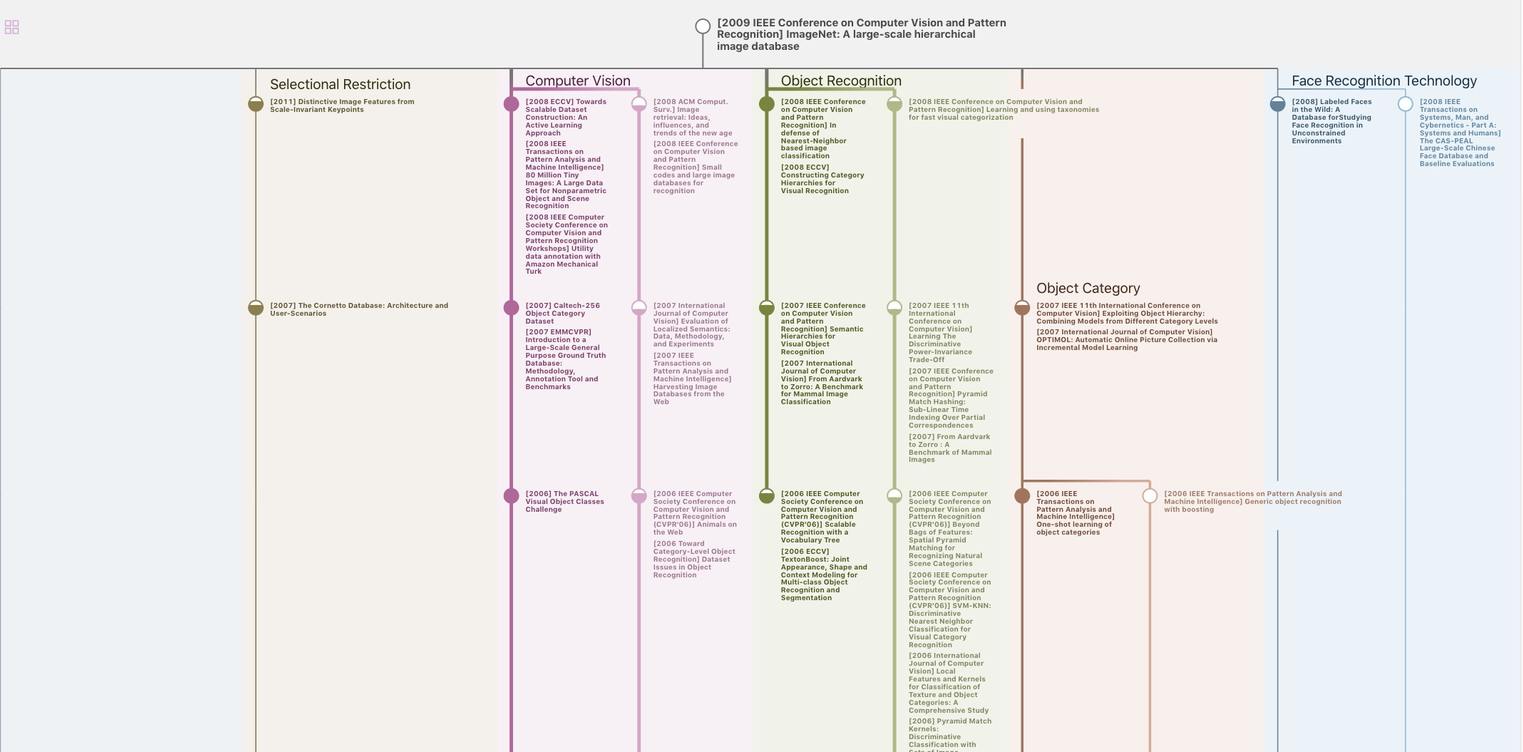
Generate MRT to find the research sequence of this paper
Chat Paper
Summary is being generated by the instructions you defined