Classification of non-coding RNA using graph representations of secondary structure.
PACIFIC SYMPOSIUM ON BIOCOMPUTING 2005(2005)
摘要
Some genes produce transcripts that function directly in regulatory, catalytic, or structural roles in the cell. These non-coding RNAs are prevalent in all living organisms, and methods that aid the understanding of their functional roles are essential. RNA secondary structure, the pattern of base-pairing, contains the critical information for determining the three dimensional structure and function of the molecule. In this work we examine whether the basic geometric and topological properties of secondary structure are sufficient to distinguish between RNA families in a learning framework. First, we develop a labeled dual graph representation of RNA secondary structure by adding biologically meaningful labels to the dual graphs proposed by Gan et al [1]. Next, we define a similarity measure directly on the labeled dual graphs using the recently developed marginalized kernels [2]. Using this similarity measure, we were able to train Support Vector Machine classifiers to distinguish RNAs of known families from random RNAs with similar statistics. For 22 of the 25 families tested, the classifier achieved better than 70% accuracy, with much higher accuracy rates for some families. Training a set of classifiers to automatically assign family labels to RNAs using a one vs. all multi-class scheme also yielded encouraging results. From these initial learning experiments, we suggest that the labeled dual graph representation, together with kernel machine methods, has potential for use in automated analysis and classification of uncharacterized RNA molecules or efficient genome-wide screens for RNA molecules from existing families.
更多查看译文
关键词
kernel machine,secondary structure,genes,graph representation,statistics,accuracy,classification,support vector machine,rna,base pair,vectors,non coding rna,rna secondary structure
AI 理解论文
溯源树
样例
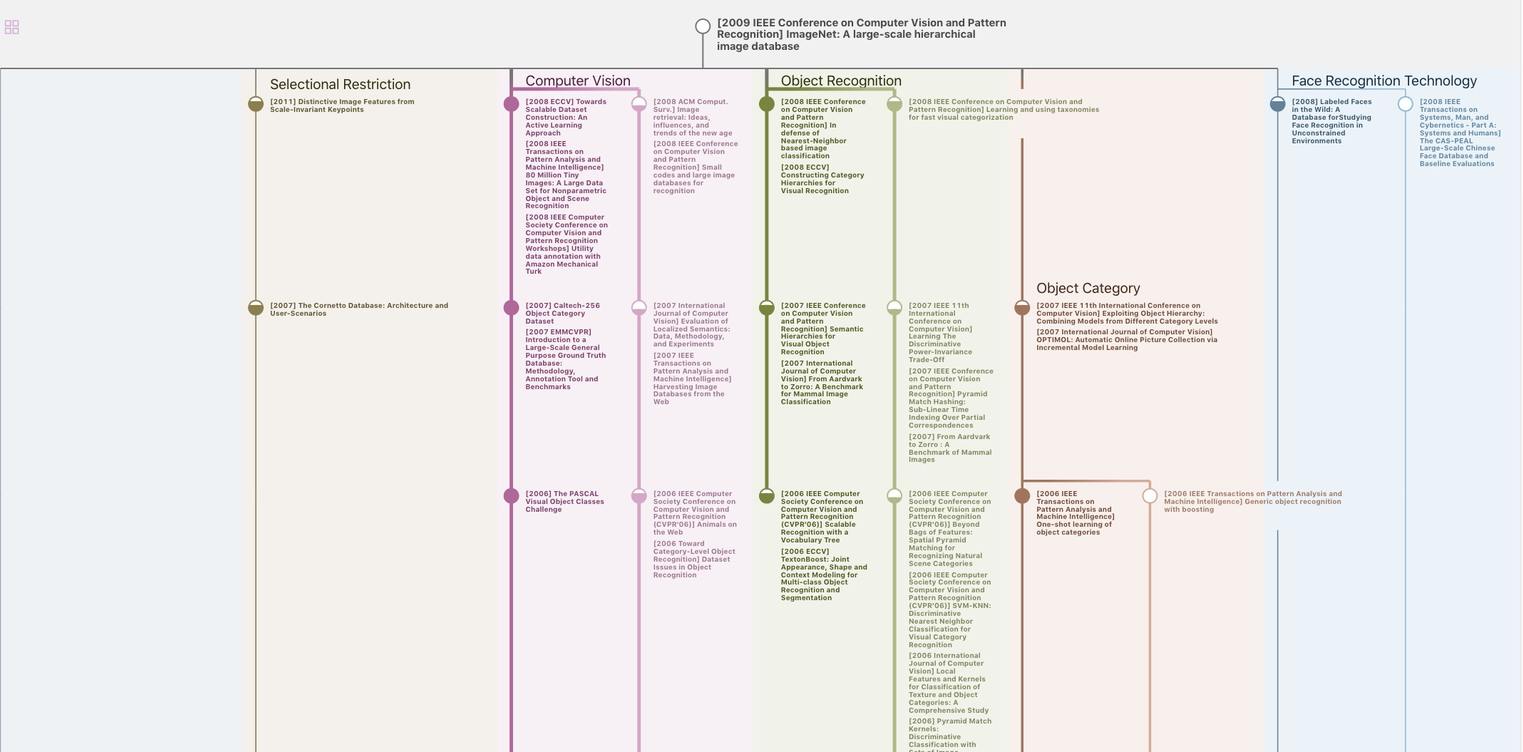
生成溯源树,研究论文发展脉络
Chat Paper
正在生成论文摘要