Optimal Feature Set and parameter Selection for Power Quality Data Mining.
APPLIED ARTIFICIAL INTELLIGENCE(2012)
摘要
The significance of detection and classification of power quality (PQ) events that disturb the voltage and/or current waveforms in electrical power distribution networks is well known. Consequently, in spite of a large number of research reports in this area, research on the selection of useful features from the existing feature set and the parameter selection for specific classifiers has thus far not been explored. The choice of a smoothing parameter for a probabilistic neural network classifier (PNN) in the training process, together with feature selection, will significantly impact the classification accuracy. In this work, a thorough analysis is carried out, using two wrapper-based optimization techniques-the genetic algorithm and simulated annealing-for identifying the ensemble of celebrated features obtained using discrete wavelet transform together with the smoothing parameter selection of the PNN classifier. As a result of these analyses, the proper smoothing parameter together with a more useful feature set from among a wider set of features for the PNN classifier is obtained with improved classification accuracy. Furthermore, the results show that the performance of simulated annealing is better than the genetic algorithm for feature selection and parameter optimization in Power Quality Data Mining.
更多查看译文
关键词
simulated annealing,data mining,genetic algorithm,discrete wavelet transform,probabilistic neural network,feature selection,electric power
AI 理解论文
溯源树
样例
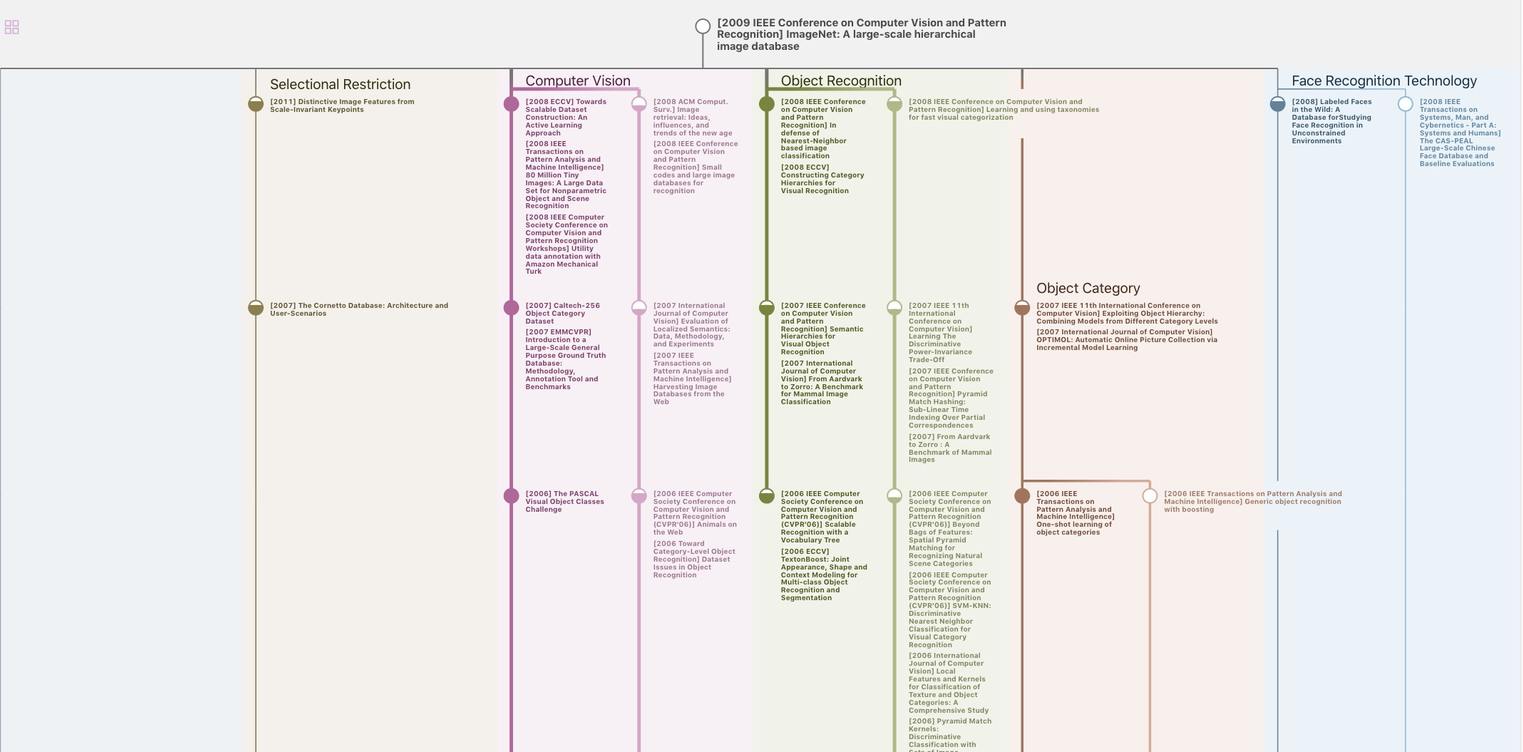
生成溯源树,研究论文发展脉络
Chat Paper
正在生成论文摘要