Predicting Response In Mobile Advertising With Hierarchical Importance-Aware Factorization Machine
WSDM(2014)
摘要
Mobile advertising has recently seen dramatic growth, fueled by the global proliferation of mobile phones and devices. The task of predicting ad response is thus crucial for maximizing business revenue. However, ad response data change dynamically over time, and are subject to cold-start situations in which limited history hinders reliable prediction. There is also a need for a robust regression estimation for high prediction accuracy, and good ranking to distinguish the impacts of different ads. To this end, we develop a Hierarchical Importance-aware Factorization Machine (HIFM), which provides an effective generic latent factor framework that incorporates importance weights and hierarchical learning. Comprehensive empirical studies on a real-world mobile advertising dataset show that HIFM outperforms the contemporary temporal latent factor models. The results also demonstrate the efficacy of the HIFM's importance-aware and hierarchical learning in improving the overall prediction and prediction in cold-start scenarios, respectively.
更多查看译文
关键词
Factorization machine,hierarchy,importance weight,mobile advertising,response prediction
AI 理解论文
溯源树
样例
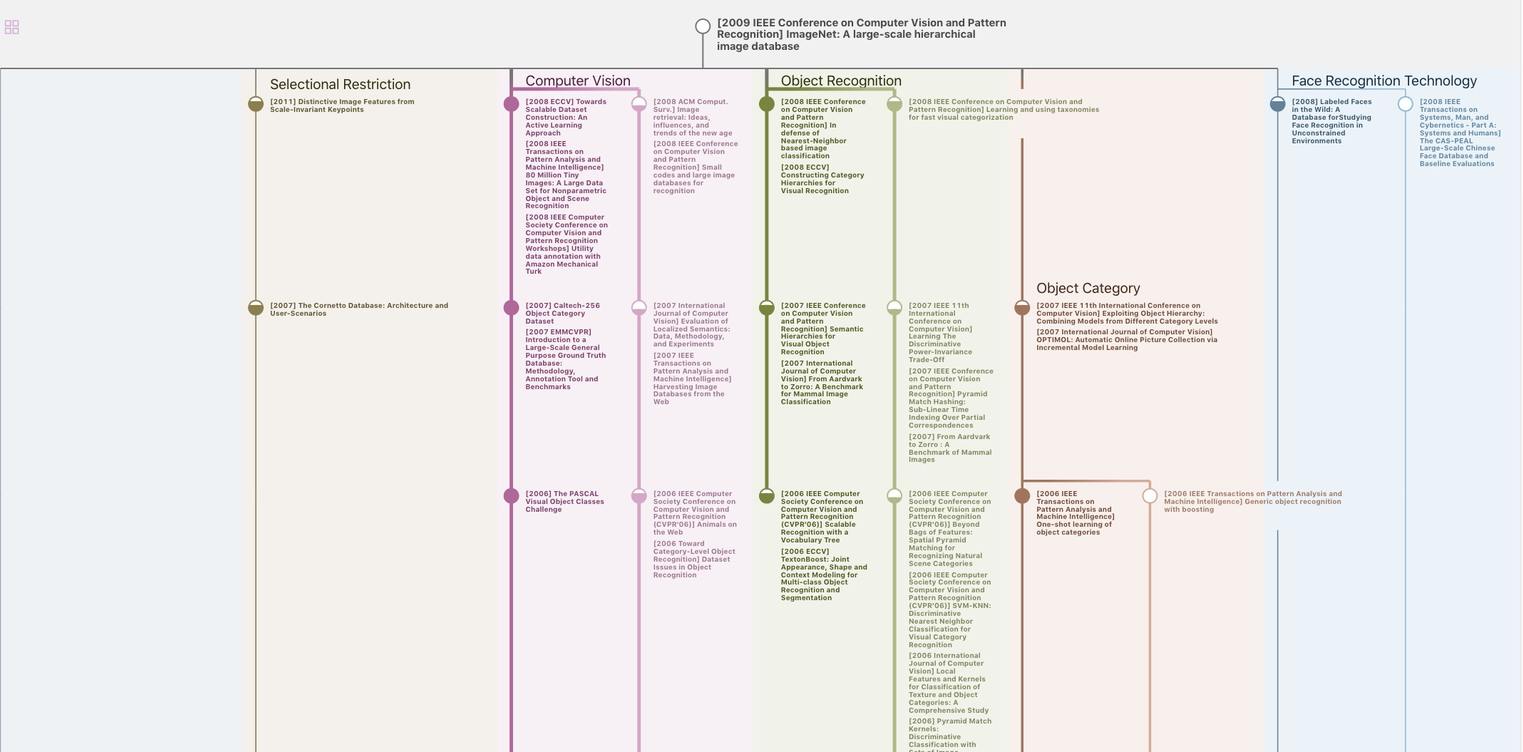
生成溯源树,研究论文发展脉络
Chat Paper
正在生成论文摘要