Partially Supervised Anomaly Detection Using Convex Hulls On A 2d Parameter Space
PARTIALLY SUPERVISED LEARNING, PSL 2013(2013)
摘要
Anomaly detection is the problem of identifying objects appearing to be inconsistent with the remainder of that set of data. Detecting such samples is useful on various applications such as fault detection, fraud detection and diagnostic systems. Partially supervised methods for anomaly detection are interesting because they only need data labeled as one of the classes (normal or abnormal). In this paper, we propose a partially supervised framework for anomaly detection based on convex hulls in a parameter space, assuming a given probability distribution. It can be considered a framework since it supports any model for the "normal" samples. We investigate an algorithm based on this framework, assuming the Gaussian distribution for the not anomalous ("normal") data, and compared the results with the One-class SVM and Naive Bayes classifiers, as well as two statistical anomaly detectors. The proposed method shows accuracy results that are comparable or better than the competing methods. Furthermore, this approach can handle any probability distribution or mixture of distributions, allowing the user to choose a parameter space that models adequately the problem of finding anomalies.
更多查看译文
关键词
Anomaly, Outlier, Semi-supervised learning
AI 理解论文
溯源树
样例
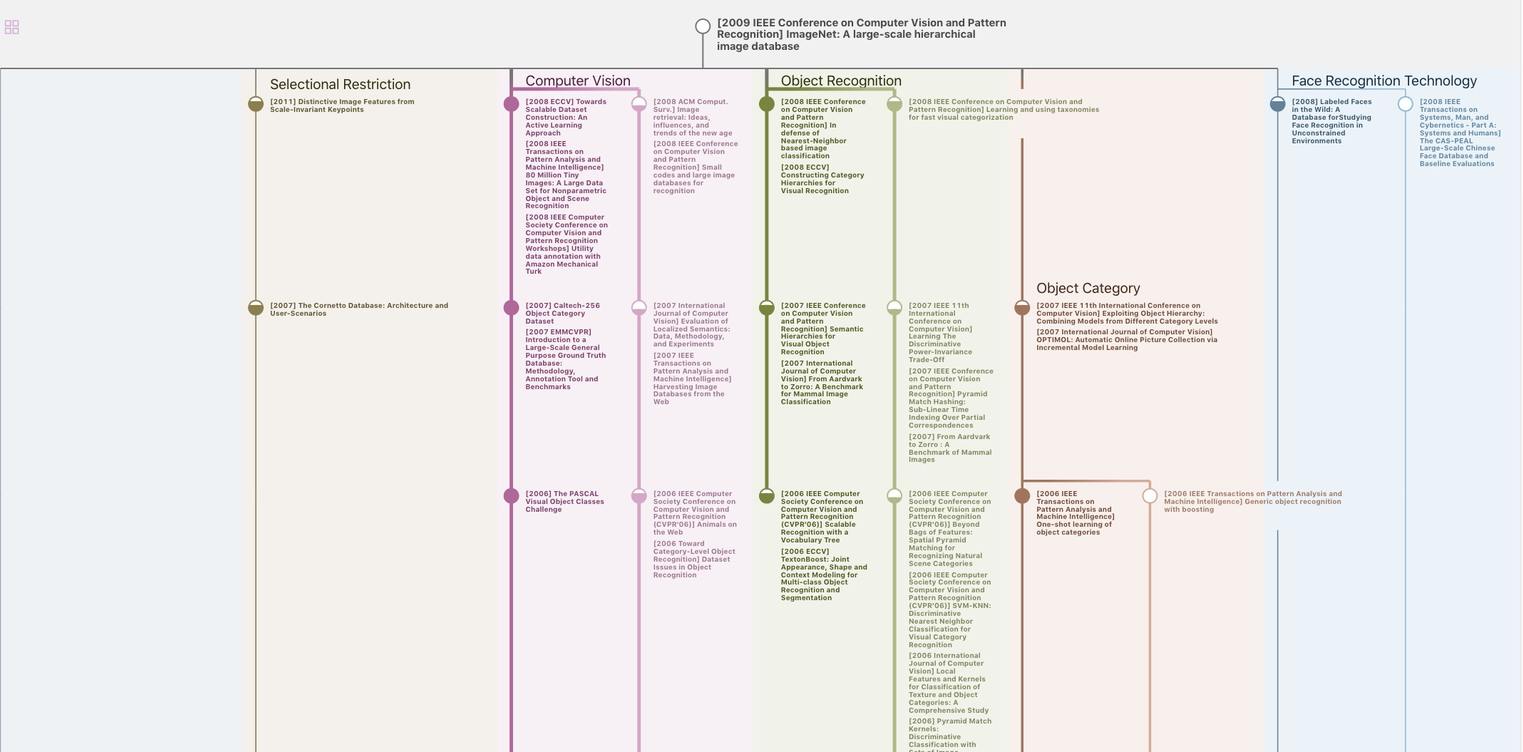
生成溯源树,研究论文发展脉络
Chat Paper
正在生成论文摘要