Disease surveillance using a hidden Markov model
BMC Medical Informatics and Decision Making(2009)
摘要
Background Routine surveillance of disease notification data can enable the early detection of localised disease outbreaks. Although hidden Markov models (HMMs) have been recognised as an appropriate method to model disease surveillance data, they have been rarely applied in public health practice. We aimed to develop and evaluate a simple flexible HMM for disease surveillance which is suitable for use with sparse small area count data and requires little baseline data. Methods A Bayesian HMM was designed to monitor routinely collected notifiable disease data that are aggregated by residential postcode. Semi-synthetic data were used to evaluate the algorithm and compare outbreak detection performance with the established Early Aberration Reporting System (EARS) algorithms and a negative binomial cusum. Results Algorithm performance varied according to the desired false alarm rate for surveillance. At false alarm rates around 0.05, the cusum-based algorithms provided the best overall outbreak detection performance, having similar sensitivity to the HMMs and a shorter average time to detection. At false alarm rates around 0.01, the HMM algorithms provided the best overall outbreak detection performance, having higher sensitivity than the cusum-based Methods and a generally shorter time to detection for larger outbreaks. Overall, the 14-day HMM had a significantly greater area under the receiver operator characteristic curve than the EARS C3 and 7-day negative binomial cusum algorithms. Conclusion Our findings suggest that the HMM provides an effective method for the surveillance of sparse small area notifiable disease data at low false alarm rates. Further investigations are required to evaluation algorithm performance across other diseases and surveillance contexts.
更多查看译文
关键词
False Alarm,Hide Markov Model,False Alarm Rate,Simulation Scenario,Postcode Area
AI 理解论文
溯源树
样例
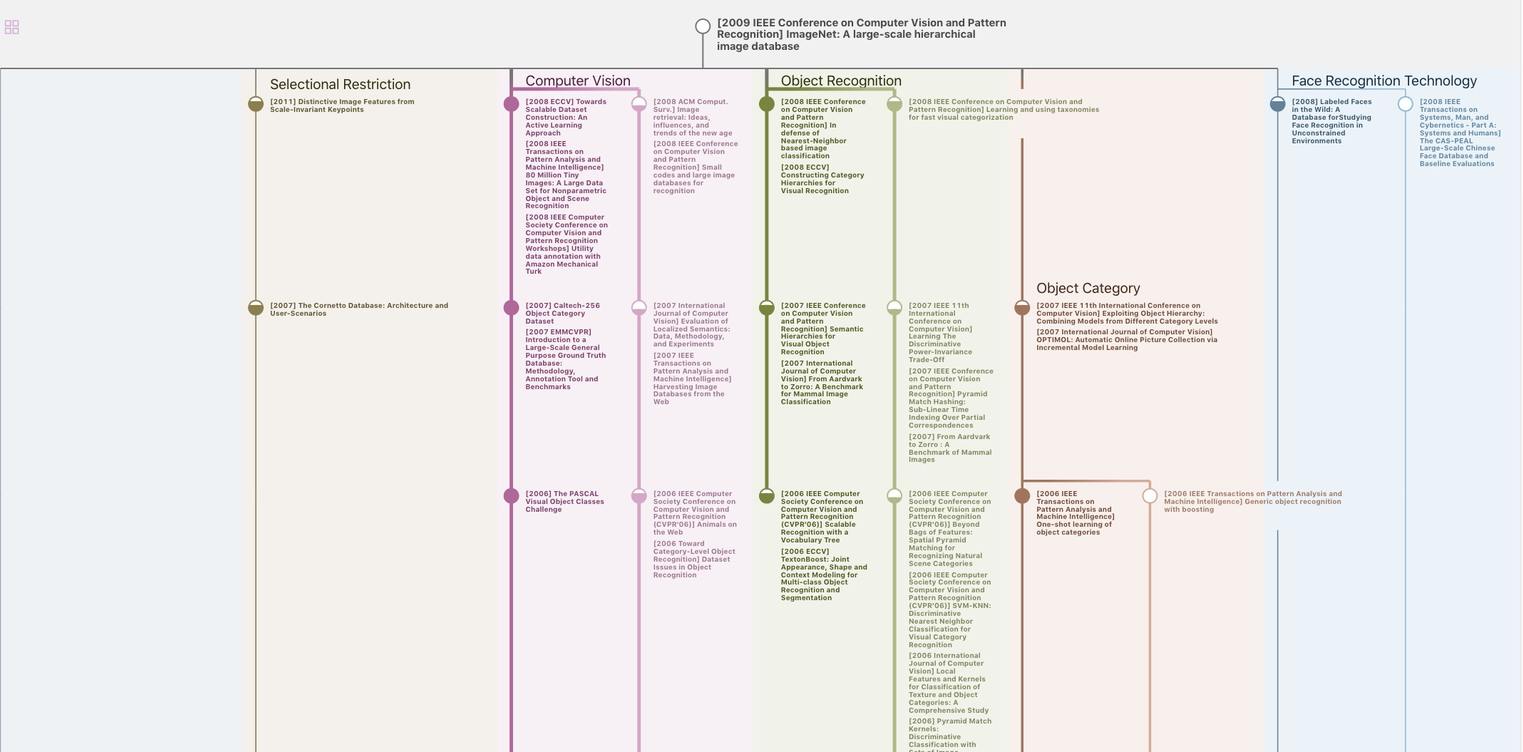
生成溯源树,研究论文发展脉络
Chat Paper
正在生成论文摘要