Primary user behavior estimation with adaptive length of the sample sequence
GLOBECOM(2012)
摘要
Modeling and estimating the primary user (PU) behavior is critical to implement dynamic spectrum access in cognitive radio networks. In this paper, we investigate the estimation accuracy of the PU behavior based on the Markov model. Maximum Likelihood (ML) estimation is employed to estimate the transition probabilities of the Markov model based on the sample sequence of PU idle/busy states. An approximate distribution of the ML estimator is derived to evaluate the estimation accuracy specified by the confidence level. To meet the requirement of estimation accuracy while reducing the unnecessary sensing time, we propose a learning algorithm which refines the estimation results iteratively. It dynamically estimates the required length of the sample sequence which is adaptive to the changing PU behavior. Numerical results show that the proposed estimation algorithm well tracks the variation of the PU behavior. Compared to the estimation method using fixed length of sample sequence, the proposed algorithm achieves the same requirement of the estimation accuracy with less number of samples.
更多查看译文
关键词
cognitive radio networks,adaptive length,markov model,primary user behavior estimation,maximum likelihood estimation,cognitive radio,dynamic spectrum access,sample sequence,markov processes
AI 理解论文
溯源树
样例
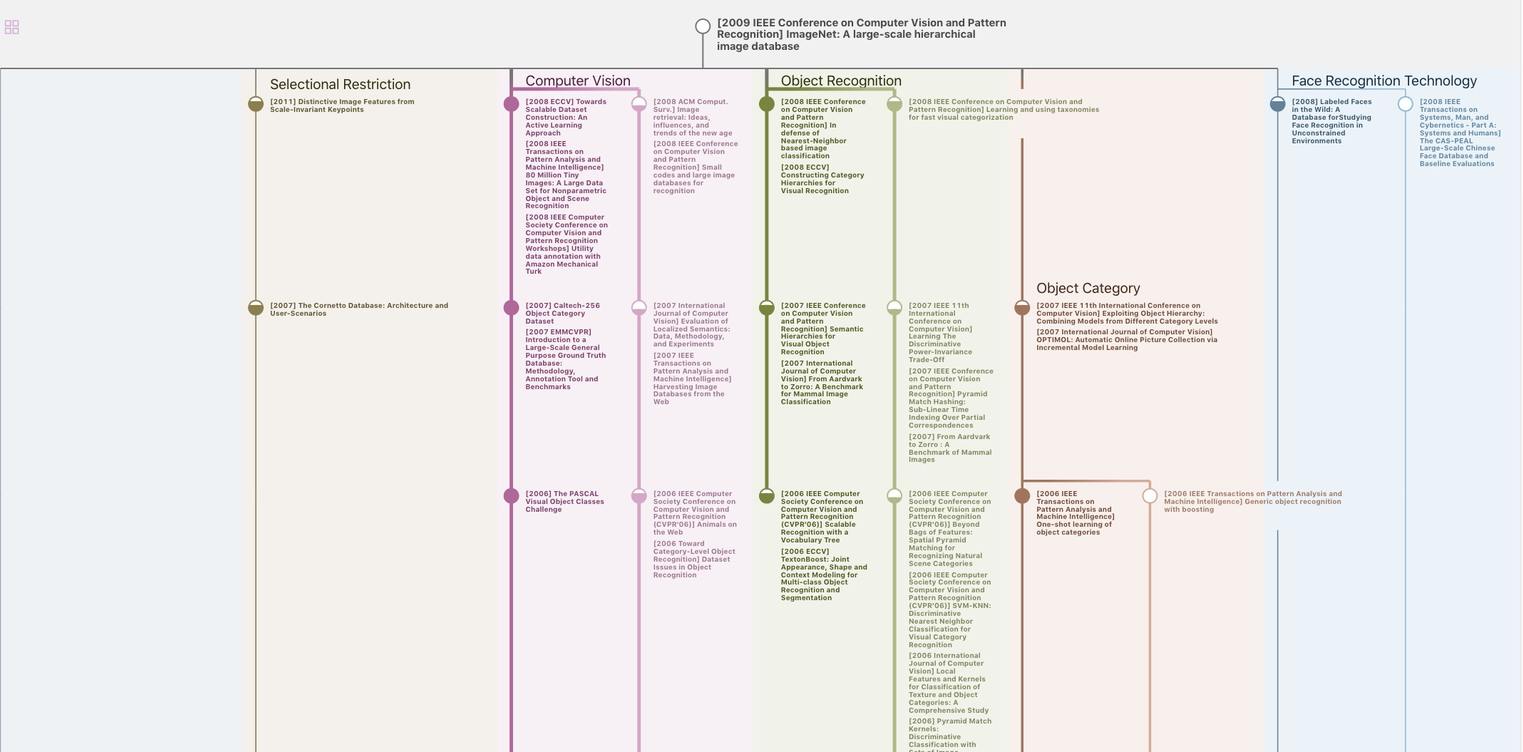
生成溯源树,研究论文发展脉络
Chat Paper
正在生成论文摘要