ACL-SAR: model agnostic adversarial contrastive learning for robust skeleton-based action recognition
The Visual Computer(2024)
Abstract
Human skeleton data have been widely explored in action recognition and the human–computer interface recently, thanks to off-the-shelf motion sensors and cameras. With the widespread usage of deep models on human skeleton data, their vulnerabilities under adversarial attacks have raised increasing security concerns. Although there are several works focusing on attack strategies, fewer efforts are put into defense against adversaries in skeleton-based action recognition, which is nontrivial. In addition, labels required in adversarial learning are another pain in adversarial training-based defense. This paper proposes a robust model agnostic adversarial contrastive learning framework for this task. First, we introduce an adversarial contrastive learning framework for skeleton-based action recognition (ACL-SAR). Second, the nature of cross-view skeleton data enables cross-view adversarial contrastive learning (CV-ACL-SAR) as a further improvement. Third, adversarial attack and defense strategies are investigated, including alternate instance-wise attacks and options in adversarial training. To validate the effectiveness of our method, we conducted extensive experiments on the NTU-RGB+D and HDM05 datasets. The results show that our defense strategies are not only robust to various adversarial attacks but can also maintain generalization.
MoreTranslated text
Key words
Skeleton-based action recognition,Adversarial training,Contrastive learning
AI Read Science
Must-Reading Tree
Example
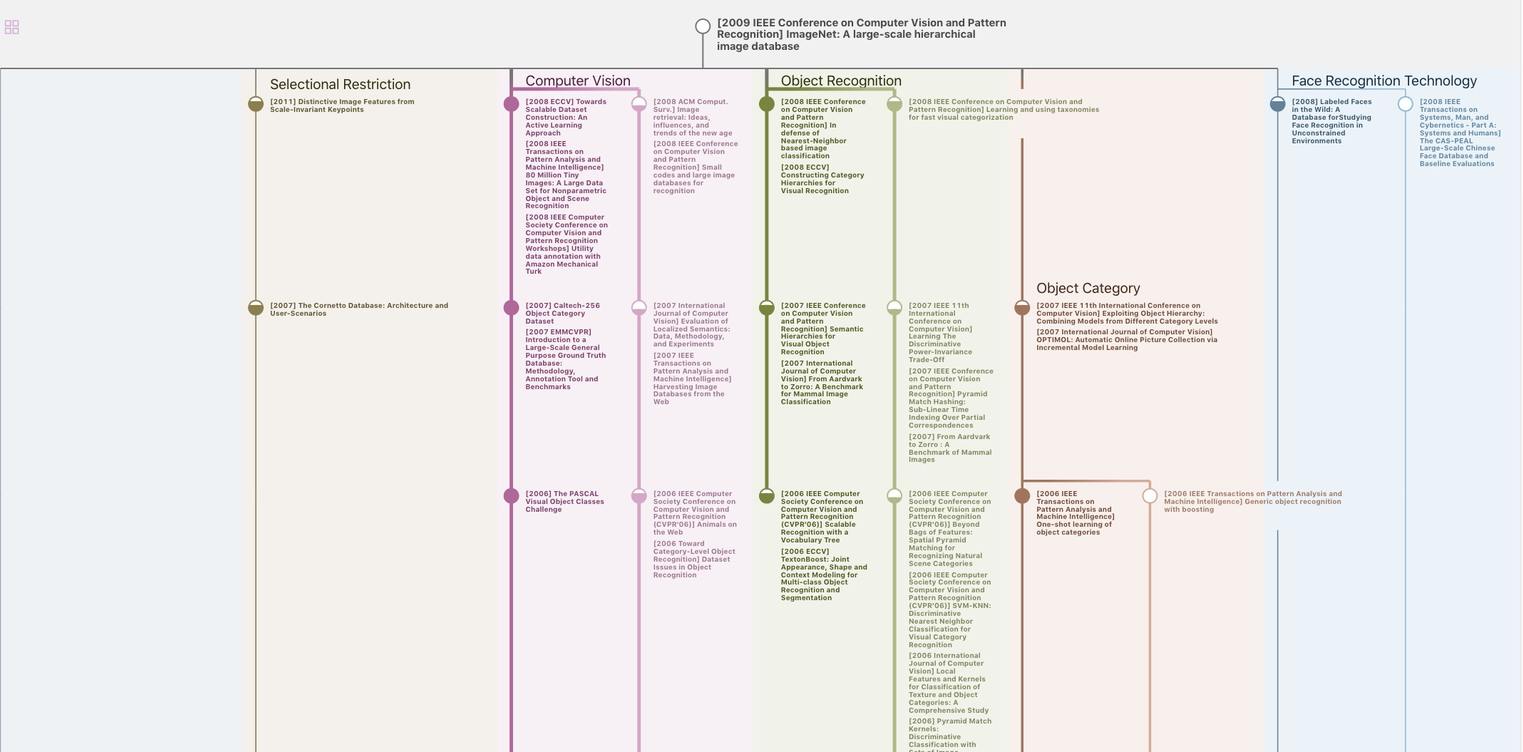
Generate MRT to find the research sequence of this paper
Chat Paper
Summary is being generated by the instructions you defined