On the Potential of Execution Traces for Batch Processing Workload Optimization in Public Clouds.
IEEE BigData(2021)
摘要
With the growing amount of data, data processing workloads and the management of their resource usage becomes increasingly important. Since managing a dedicated infrastructure is in many situations infeasible or uneconomical, users progressively execute their respective workloads in the cloud. As the configuration of workloads and resources is often challenging, various methods have been proposed that either quickly profile towards a good configuration or determine one based on data from previous runs. Still, performance data to train such methods is often lacking and must be costly collected. In this paper, we propose a collaborative approach for sharing anonymized workload execution traces among users, mining them for general patterns, and exploiting clusters of historical workloads for future optimizations. We evaluate our prototype implementation for mining workload execution graphs on a publicly available trace dataset and demonstrate the predictive value of workload clusters determined through traces only.
更多查看译文
关键词
Distributed Data Processing,Workload Optimization,Cloud Computing,Performance Modeling
AI 理解论文
溯源树
样例
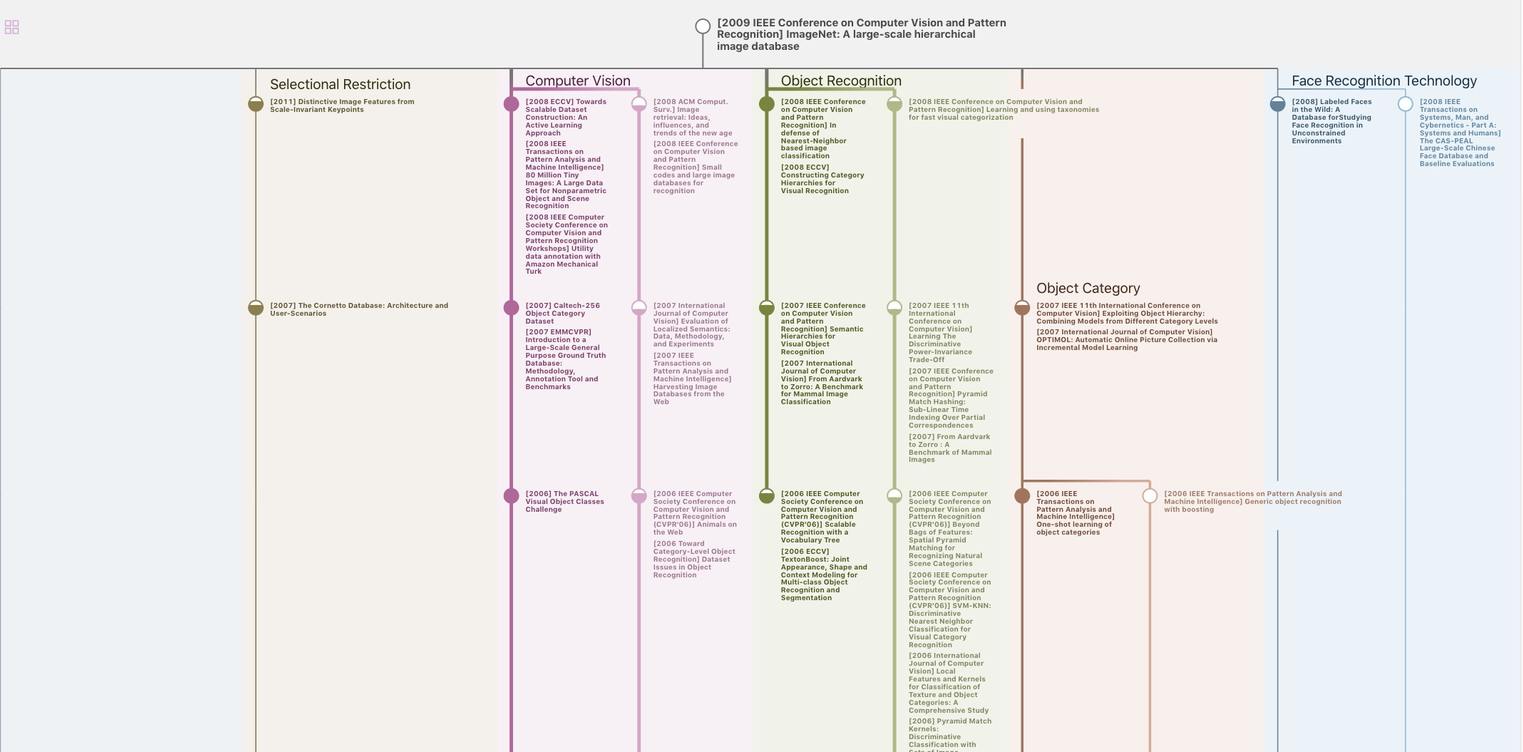
生成溯源树,研究论文发展脉络
Chat Paper
正在生成论文摘要