Error Estimation in Approximate Bayesian Belief Network Inference
UAI'95: Proceedings of the Eleventh conference on Uncertainty in artificial intelligence(2013)
摘要
We can perform inference in Bayesian belief networks by enumerating instantiations with high probability thus approximating the marginals. In this paper, we present a method for determining the fraction of instantiations that has to be considered such that the absolute error in the marginals does not exceed a predefined value. The method is based on extreme value theory. Essentially, the proposed method uses the reversed generalized Pareto distribution to model probabilities of instantiations below a given threshold. Based on this distribution, an estimate of the maximal absolute error if instantiations with probability smaller than u are disregarded can be made.
更多查看译文
关键词
enumerating instantiations,proposed method,absolute error,extreme value theory,high probability,maximal absolute error,model probability,predefined value,reversed generalized Pareto distribution,Bayesian belief network,approximate Bayesian belief network,error estimation
AI 理解论文
溯源树
样例
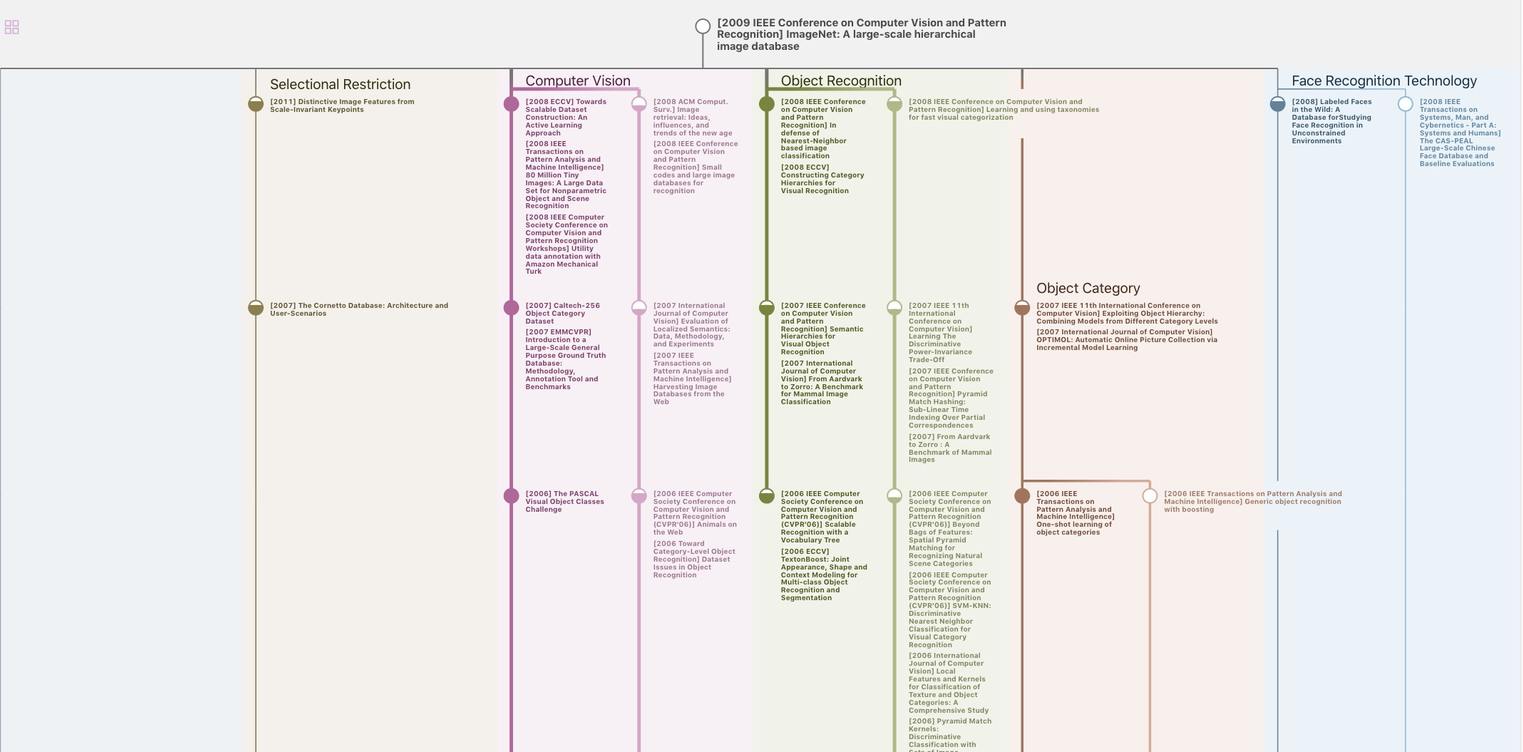
生成溯源树,研究论文发展脉络
Chat Paper
正在生成论文摘要