A martingale approach and time-consistent sampling-based algorithms for risk management in stochastic optimal control
CDC(2014)
摘要
In this paper, we consider a class of stochastic optimal control problems with risk constraints that are expressed as bounded probabilities of failure for particular initial states. We present here a martingale approach that diffuses a risk constraint into a martingale to construct time-consistent control policies. The martingale stands for the level of risk tolerance that is contingent on available information over time. By augmenting the system dynamics with the controlled martingale, the original risk-constrained problem is transformed into a stochastic target problem. We extend the incremental Markov Decision Process (iMDP) algorithm to approximate arbitrarily well an optimal feedback policy of the original problem by sampling in the augmented state space and computing proper boundary conditions for the reformulated problem. We show that the algorithm is both probabilistically sound and asymptotically optimal. The performance of the proposed algorithm is demonstrated on motion planning and control problems subject to bounded probability of collision in uncertain cluttered environments.
更多查看译文
关键词
imdp algorithm,bounded collision probability,motion planning,optimal control,incremental markov decision process,motion control,time-consistent control policy,stochastic systems,optimal feedback policy,risk tolerance,stochastic optimal control,risk management,risk constraints,markov processes,time-consistent sampling-based algorithm,martingale approach
AI 理解论文
溯源树
样例
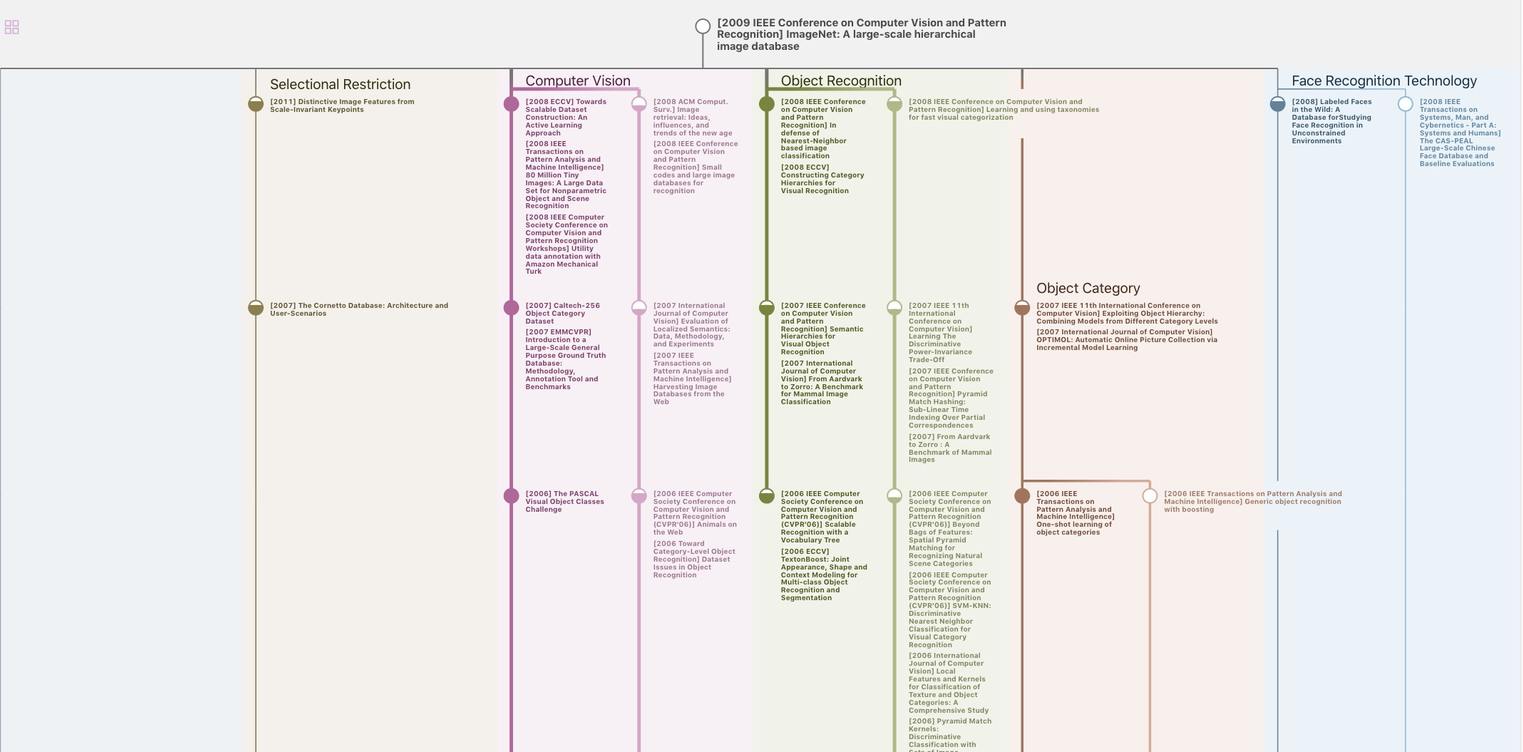
生成溯源树,研究论文发展脉络
Chat Paper
正在生成论文摘要