Collaborative Users' Brand Preference Mining across Multiple Domains from Implicit Feedbacks.
AAAI(2011)
摘要
Advanced e-applications require comprehensive knowledge about their users' preferences in order to provide accurate personalized services. In this paper, we propose to learn users' preferences to product brands from their implicit feedbacks such as their searching and browsing behaviors in user Web browsing log data. The user brand preference learning problem is challenge since (1) the users' implicit feedbacks are extremely sparse in various product domains; and (2) we can only observe positive feedbacks from users' behaviors. In this paper, we propose a latent factor model to collaboratively mine users' brand preferences across multiple domains simultaneously. By collective learning, the learning processes in all the domains are mutually enhanced and hence the problem of data scarcity in each single domain can be effectively addressed. On the other hand, we learn our model with an adaption of the Bayesian personalized ranking (BPR) optimization criterion which is a general learning framework for collaborative filtering from implicit feedbacks. Experiments with both synthetic and real world datasets show that our proposed model significantly outperforms the baselines. Copyright © 2011, Association for the Advancement of Artificial Intelligence (www.aaai.org). All rights reserved.
更多查看译文
AI 理解论文
溯源树
样例
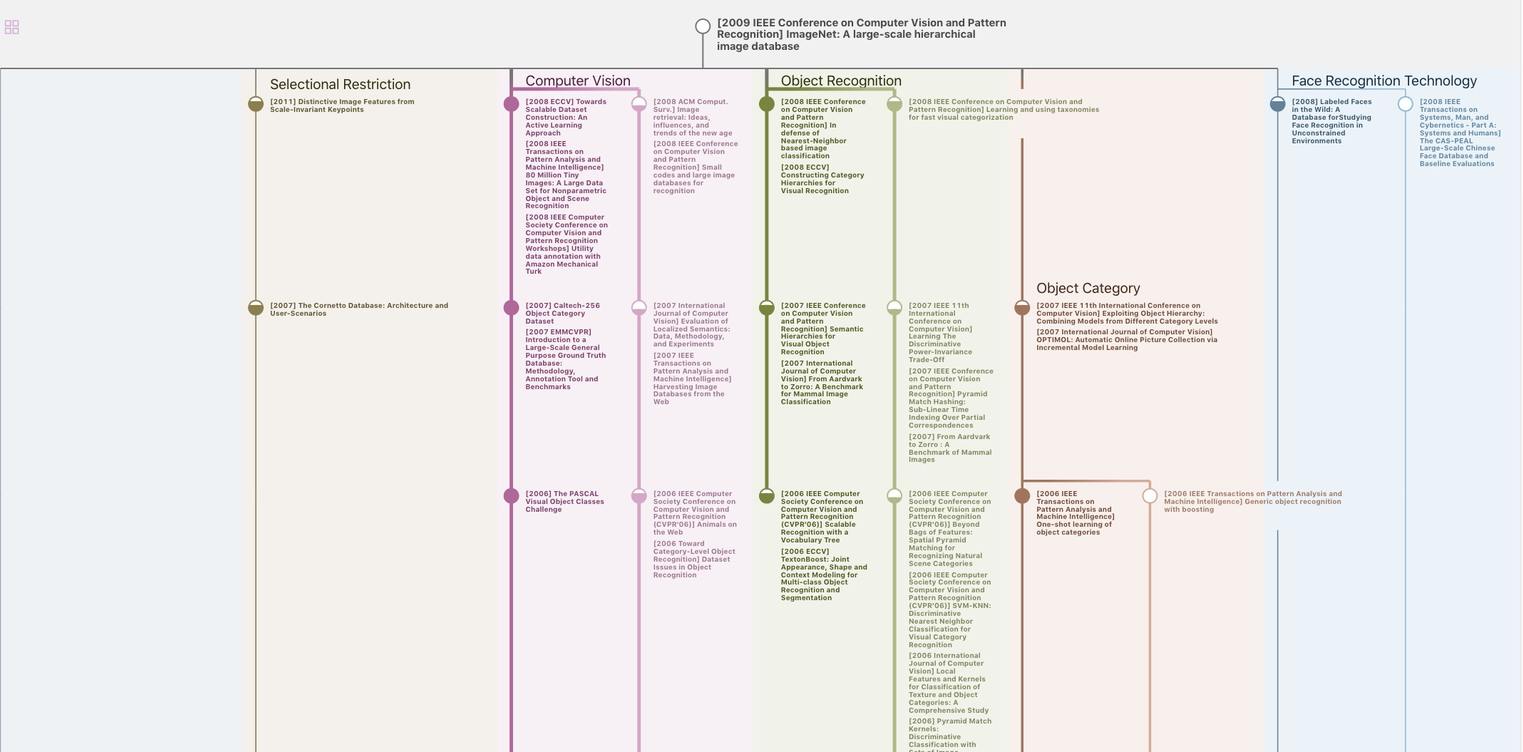
生成溯源树,研究论文发展脉络
Chat Paper
正在生成论文摘要