Document Difficulty Framework for Semi-automatic Text Classification
DaWaK(2013)
摘要
Text Classification systems are able to deal with large datasets, spending less time and human cost compared with manual classification. This is achieved, however, in expense of loss in quality. Semi-Automatic Text Classification SATC aims to achieve high quality with minimum human effort by ranking the documents according to their estimated certainty of being correctly classified. This paper introduces the Document Difficulty Framework DDF, a unification of different strategies to estimate the document certainty, and its application to SATC. DDF exploits the scores and thresholds computed by any given classifier. Different metrics are obtained by changing the parameters of the three levels the framework is lied upon: how to measure the confidence for each document-class evidence, which classes to observe class and how to aggregate this knowledge aggregation. Experiments show that DDF metrics consistently achieve high error reduction with large portions of the collection being automatically classified. Furthermore, DDF outperforms all the reported SATC methods in the literature.
更多查看译文
关键词
Human Expert, Evidence Level, Correct Class, Positive Label, Thresholding Strategy
AI 理解论文
溯源树
样例
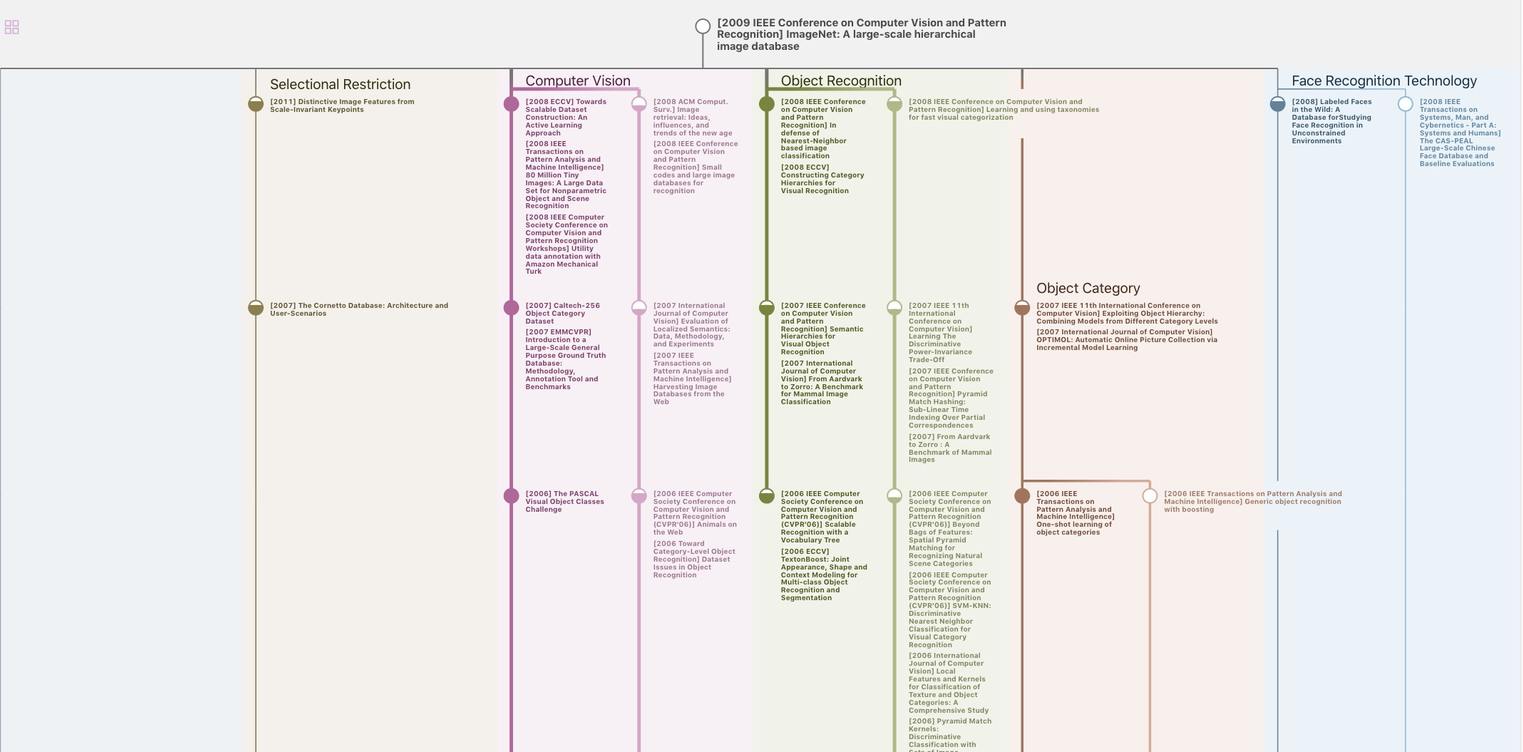
生成溯源树,研究论文发展脉络
Chat Paper
正在生成论文摘要