Transfer Learning In Mir: Sharing Learned Latent Representations For Music Audio Classification And Similarity.
ISMIR 2013(2013)
Abstract
This paper discusses the concept of transfer learning and its potential applications to MIR tasks such as music audio classification and similarity. In a traditional supervised machine learning setting, a system can only use labeled data from a single dataset to solve a given task. The labels associated with the dataset define the nature of the task to solve. A key advantage of transfer learning is in leveraging knowledge from related tasks to improve performance on a given target task. One way to transfer knowledge is to learn a shared latent representation across related tasks. This method has shown to be beneficial in many domains of machine learning, but has yet to be explored in MIR. Many MIR datasets for audio classification present a semantic overlap in their labels. Furthermore, these datasets often contain relatively few songs. Thus, there is a strong case for exploring methods to share knowledge between these datasets towards a more general and robust understanding of high level musical concepts such as genre and similarity. Our results show that shared representations can improve classification accuracy. We also show how transfer learning can improve performance for music similarity.
MoreTranslated text
Key words
music audio classification,transfer learning,mir,latent representations
AI Read Science
Must-Reading Tree
Example
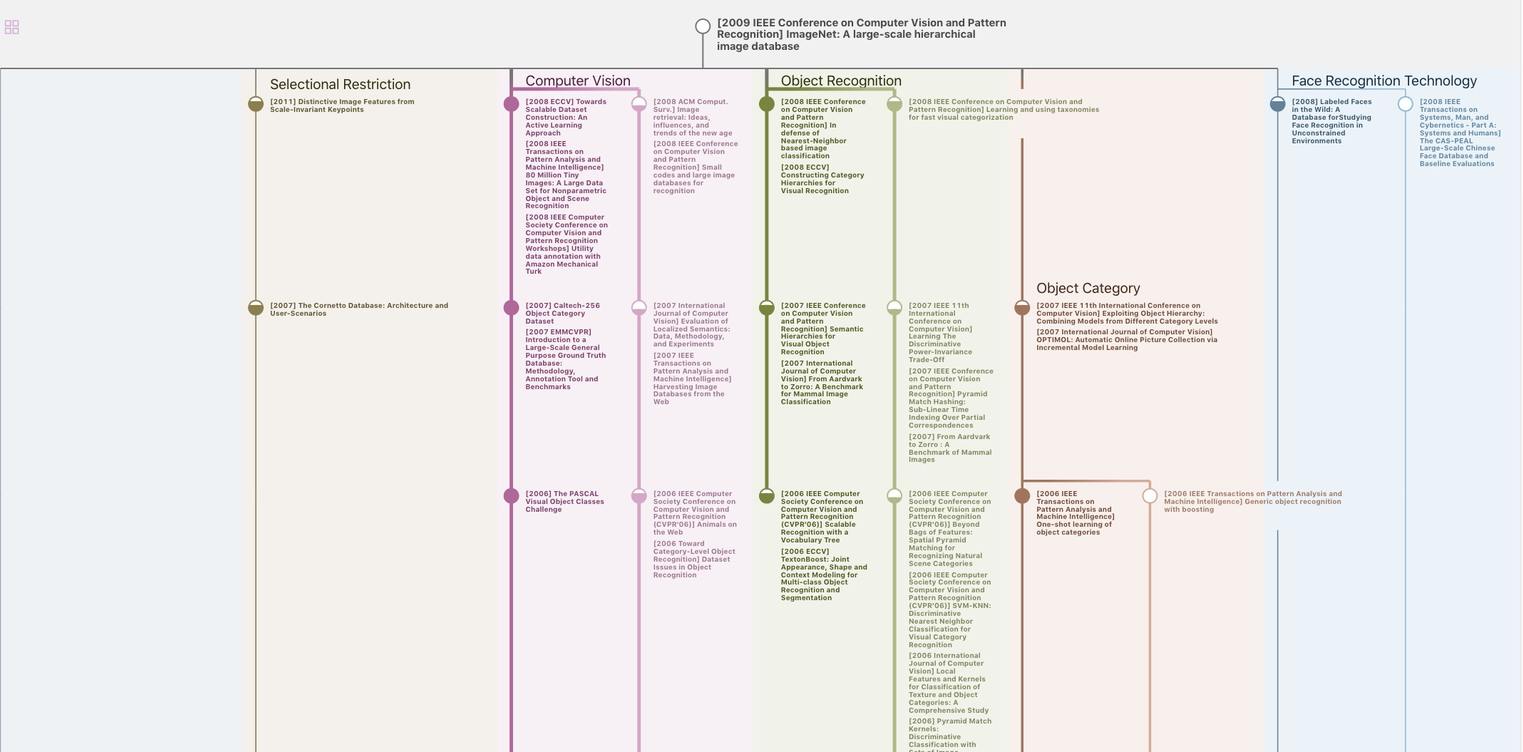
Generate MRT to find the research sequence of this paper
Chat Paper
Summary is being generated by the instructions you defined