A probabilistic and continuous model of protein conformational space for template-free modeling.
JOURNAL OF COMPUTATIONAL BIOLOGY(2010)
摘要
One of the major challenges with protein template-free modeling is an efficient sampling algorithm that can explore a huge conformation space quickly. The popular fragment assembly method constructs a conformation by stringing together short fragments extracted from the Protein Data Base (PDB). The discrete nature of this method may limit generated conformations to a subspace in which the native fold does not belong. Another worry is that a protein with really new fold may contain some fragments not in the PDB. This article presents a probabilistic model of protein conformational space to overcome the above two limitations. This probabilistic model employs directional statistics to model the distribution of backbone angles and 2(nd)-order Conditional Random Fields (CRFs) to describe sequence-angle relationship. Using this probabilistic model, we can sample protein conformations in a continuous space, as opposed to the widely used fragment assembly and lattice model methods that work in a discrete space. We show that when coupled with a simple energy function, this probabilistic method compares favorably with the fragment assembly method in the blind CASP8 evaluation, especially on alpha or small beta proteins. To our knowledge, this is the first probabilistic method that can search conformations in a continuous space and achieves favorable performance. Our method also generated three-dimensional (3D) models better than template-based methods for a couple of CASP8 hard targets. The method described in this article can also be applied to protein loop modeling, model refinement, and even RNA tertiary structure prediction.
更多查看译文
关键词
conditional random fields (CRFs),directional statistics,fragment assembly,lattice model,protein structure prediction,template-free modeling
AI 理解论文
溯源树
样例
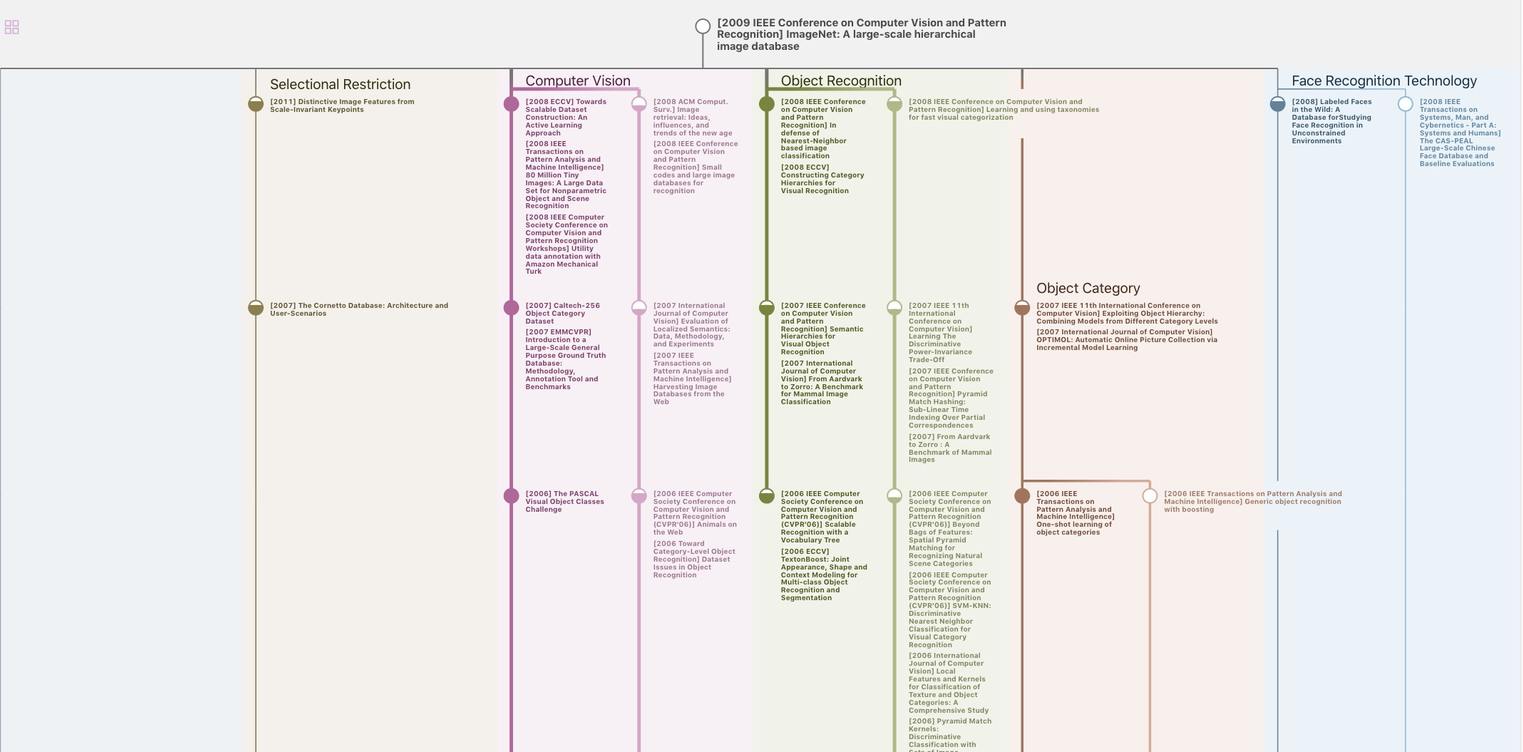
生成溯源树,研究论文发展脉络
Chat Paper
正在生成论文摘要