SU-FF-T-480: Minimum Multi-Plan IMRT Framework Knowledge Base for Predicting Organ-At-Risk Dose-Volume Levels and Complications
MEDICAL PHYSICS(2009)
摘要
Purpose: To determine the minimum number of treatment plans required to build accurate representations of the IMRT plan surface in order to predict organ‐at‐risk (OAR) dose‐volume (DV) levels and complications as a function of input DV constraint settings. Method and Materials: 125 and 256 plans respectively were generated as the full knowledge base for a representative head and neck case and prostate case by varying input DV constraints on OARs (parotids, cord, bladder, bowel and rectum). Knowledge subsets of various sizes were used to determine the minimum sizes needed to obtain accurate plan surfacemodels and accurate complication predictions via Machine Learning algorithms. Predictions of saliva flow rate were obtained via a sequential minimal optimization algorithm for training a support vector regression model. Binary classification via optimized decision trees was used to predict rectal bleeding. To build the plan surface for each of the two test cases, 100%, 50%, 25%, and 12.5% of the full knowledge base of plans were used (6.25% was also used for pelvis case). For each fractional value 50 random samples of the corresponding size were used as training sets. Results: The doses received at various volume levels can be modeled with less than 2% mean relative errors with 30 plans (95% confidence interval (C.I.) on largest error was [1.66%, 1.72%]). Saliva flow rate can be predicted with 1.91% mean relative error and a 95% C.I. [1.87%, 1.94%] with 30 plans. Grade 2 rectal bleeding complications can be predicted with mean correct prediction of 92.64% and a 95% C.I. [92.18%, 93.09%] using 64 plans. Conclusion: Our results indicate that only a small number of plans (30–64) are needed in order to model the plan surface and to obtain models that yield accurate prediction of OAR DV levels and complications as a function of DV constraints.
更多查看译文
关键词
knowledge base
AI 理解论文
溯源树
样例
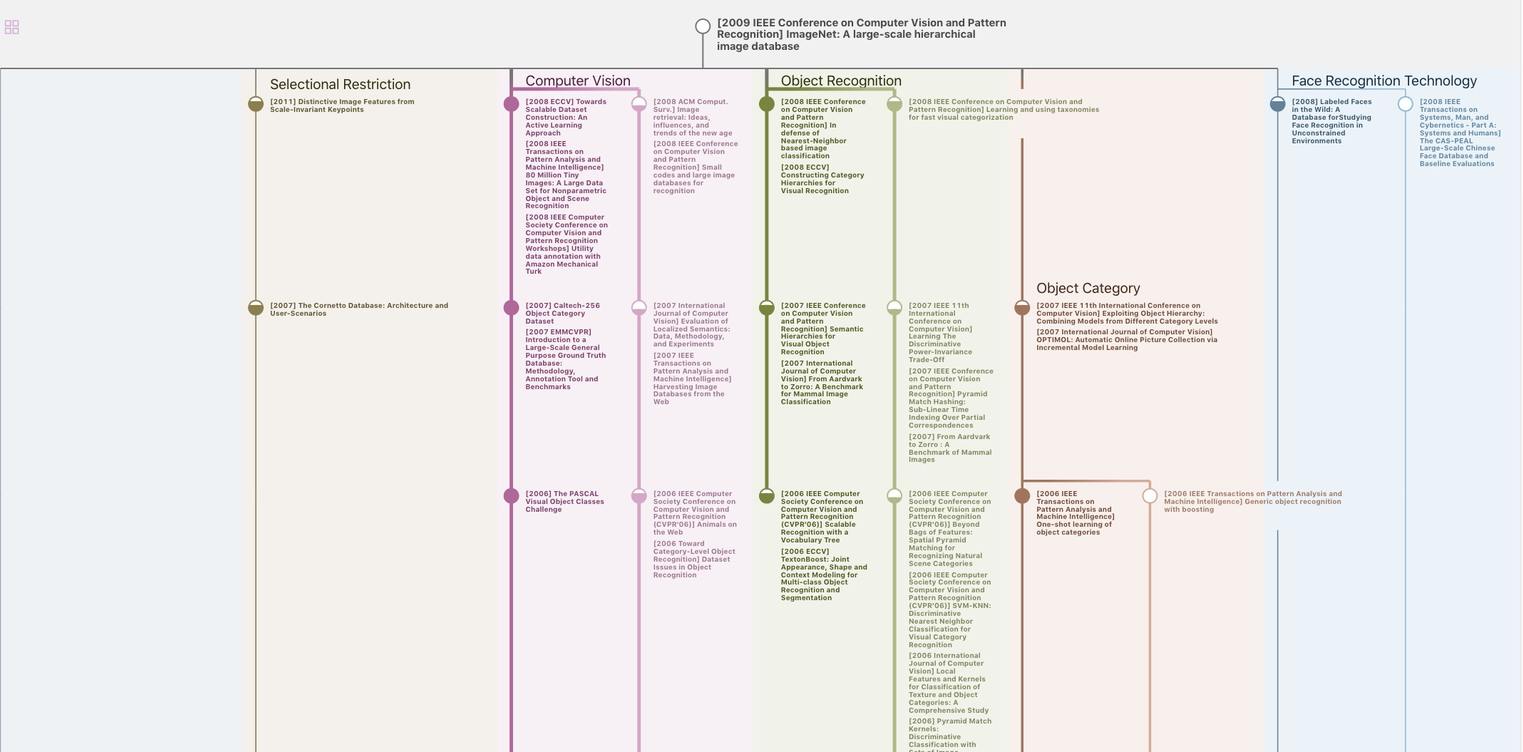
生成溯源树,研究论文发展脉络
Chat Paper
正在生成论文摘要