Transfer Learning Applied to Stellar Light Curve Classification
arXiv (Cornell University)(2023)
摘要
Variability carries physical patterns and astronomical information of objects, and stellar light curve variations are essential to understand the stellar formation and evolution processes. The studies of variations in stellar photometry have the potential to expand the list of known stars, protostars, binary stars, and compact objects, which could shed more light on stages of stellar lifecycles. The progress in machine-learning techniques and applications has developed modern algorithms to detect and condense features from big data, which enables us to classify stellar light curves efficiently and effectively. We explore several deep-learning methods on variable star classifications. The sample of light curves is constructed with $\delta$ Scuti, $\gamma$ Doradus, RR Lyrae, eclipsing binaries, and hybrid variables from \textit{Kepler} observations. Several algorithms are applied to transform the light curves into images, continuous wavelet transform (CWT), Gramian angular fields, and recurrent plots. We also explore the representation ability of these algorithms. The processed images are fed to several deep-learning methods for image recognition, including VGG-19, GoogLeNet, Inception-v3, ResNet, SqueezeNet, and Xception architectures. The best transformation method is CWT, resulting in an average accuracy of 95.6\%. VGG-19 shows the highest average accuracy of 93.25\% among all architectures, while it shows the highest accuracy of 97.2\% under CWT transformation method. The prediction can reach $\sim1000$ light curves per second by using NVIDIA RTX 3090. Our results indicate that the combination of big data and deep learning opens a new path to classify light curves automatically.
更多查看译文
关键词
stellar light curve classification,learning
AI 理解论文
溯源树
样例
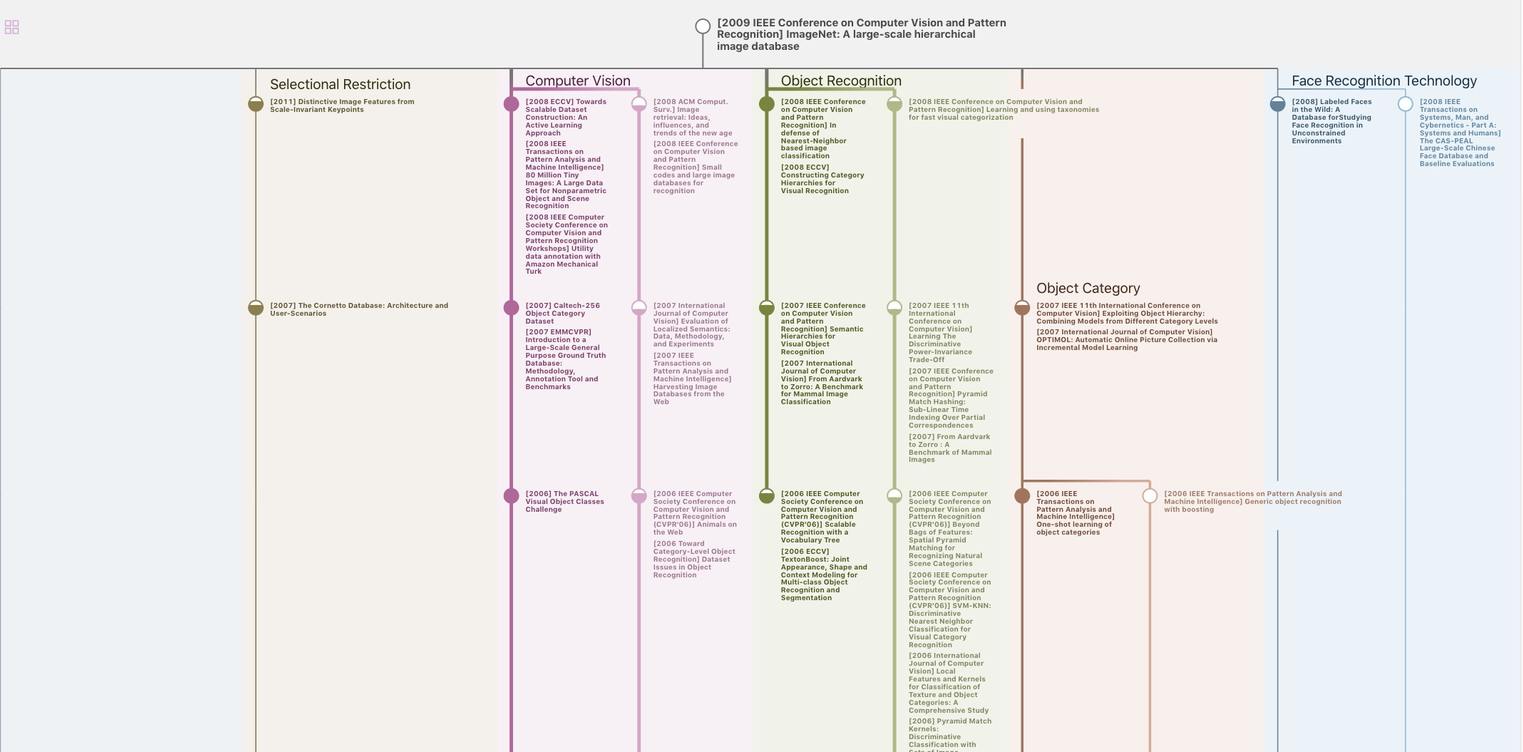
生成溯源树,研究论文发展脉络
Chat Paper
正在生成论文摘要