Extended wavelet sparse convolutional neural network (EWSCNN) for super resolution
SADHANA-ACADEMY PROCEEDINGS IN ENGINEERING SCIENCES(2023)
摘要
Multiresolution property of wavelet transform at first level decomposition is used for single image super-resolution (SISR) in this paper. Earlier, wavelet based SISR methods used generative approaches, but in this work, discriminative approach is used. With the help of deep CNN, we attempt to learn a complex end-to-end nonlinear mapping between low-resolution (LR) and high-resolution (HR) patches in order to estimate the LR patch's missing details. The input LR patch comprises of four sub-bands of LR image wavelet coefficients and the output HR patches are the residuals of four sub-bands of HR image wavelet coefficients. We reduce the low frequency information reconstruction problem by utilizing residues of wavelet sub bands. A key advantage of residual learning is speeding up the training and providing better reconstruction accuracy. We name the deep CNN proposed for super resolution in this work as Extended Sparse Wavelet Convolutional Neural Network for Super Resolution (EWSCNN-SR). The proposed EWSCNN-SR net provides better performance when compared to the other state-of-the-art SISR methods.
更多查看译文
关键词
Discriminative approach,Extended Sparse Wavelet CNN,reconstruction artifacts,wavelet sub-bands,sparsity
AI 理解论文
溯源树
样例
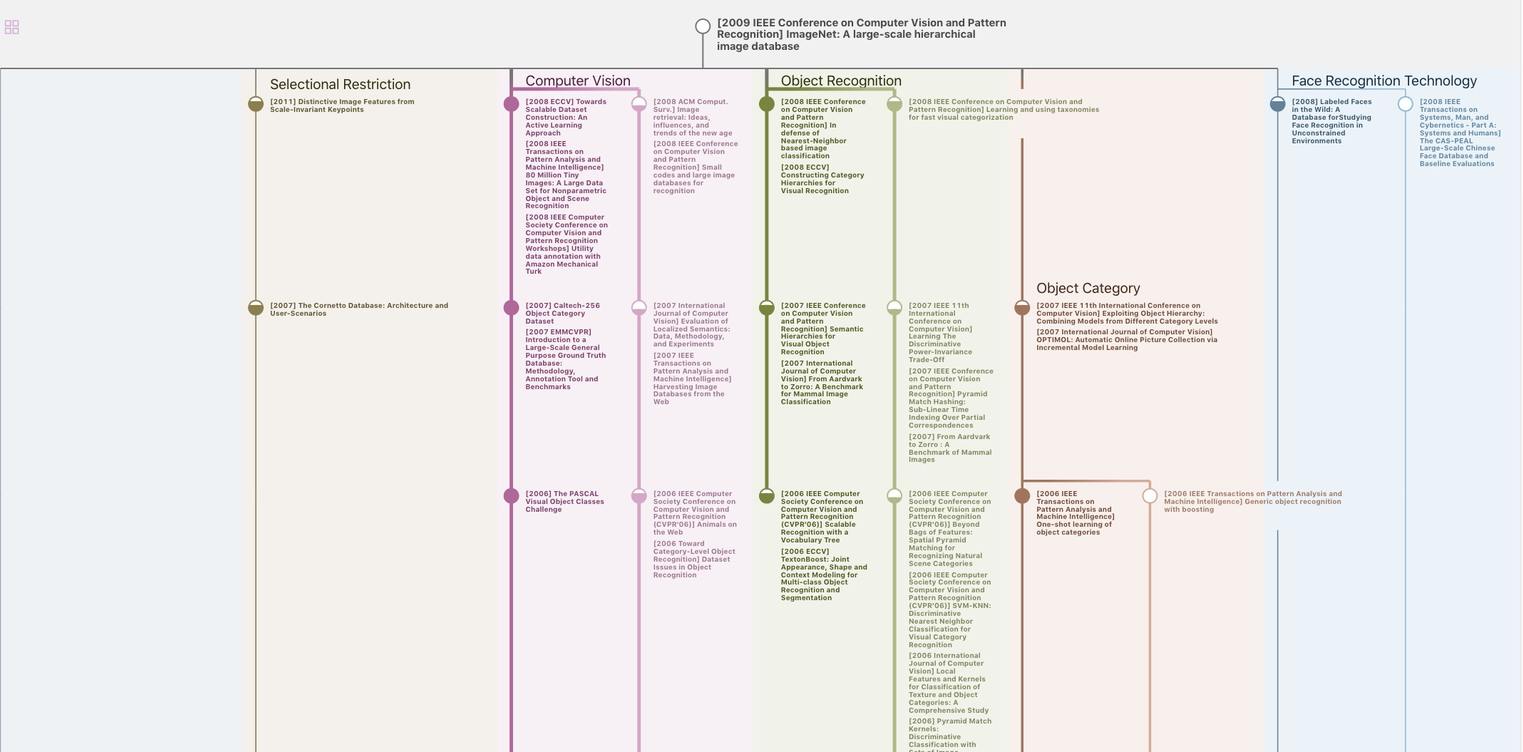
生成溯源树,研究论文发展脉络
Chat Paper
正在生成论文摘要