A formal approach to score normalization for meta-search
HLT '02: Proceedings of the second international conference on Human Language Technology Research(2002)
摘要
Meta-search, or the combination of the outputs of different search engines in response to a query, has been shown to improve performance. Since the scores produced by different search engines are not comparable, researchers have often decomposed the meta-search problem into a score normalization step followed by a combination step. Combination has been studied by many researchers. While appropriate normalization can affect performance, most of the normalization schemes suggested are ad hoc in nature. In this paper, we propose a formal approach to normalizing scores for meta-search by taking the distributions of the scores into account. Recently, it has been shown that for search engines the score distributions for a given query may be modeled using an exponential distribution for the set of non-relevant documents and a normal distribution for the set of relevant documents. Here, it is shown that by equalizing the distributions of scores of the top non-relevant documents the best meta-search performance reported in the literature is obtained. Since relevance information is not available apri-ori, we discuss two different ways of obtaining a good approximation to the distribution of scores of non-relevant documents. One is obtained by looking at the distribution of scores of all documents. The second is obtained by fitting a mixture model of an exponential and a Gaussian to the scores of all documents and using the resulting exponential distribution as an estimate of the non-relevant distribution. We show with experiments on TREC-3, TREC-4 and TREC-9 data that the best combination results are obtained by averaging the parameters obtained from these approximations. These techniques work on a variety of different search engines including vector space search engines like SMART and probabilistic search engines like INQUERY. The problem of normalization is important in many other areas including information filtering, topic detection and tracking, multilingual search and distributed retrieval. Thus, the techniques proposed here are likely to be applicable to many of these tasks.
更多查看译文
关键词
different search engine,exponential distribution,non-relevant document,multilingual search,probabilistic search engine,search engine,vector space search engine,non-relevant distribution,normal distribution,score distribution,formal approach,score normalization
AI 理解论文
溯源树
样例
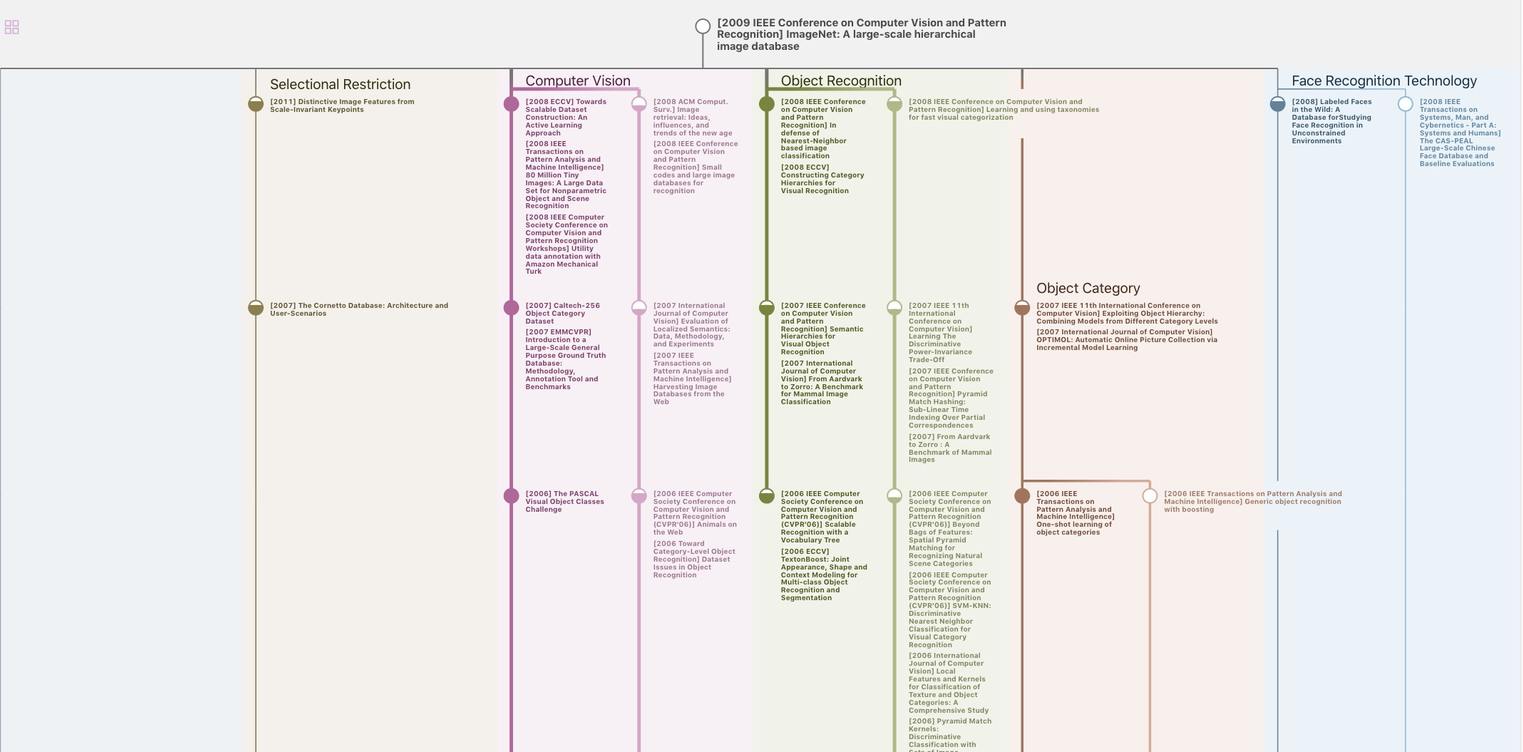
生成溯源树,研究论文发展脉络
Chat Paper
正在生成论文摘要