Automatic key term extraction from spoken course lectures using branching entropy and prosodic/semantic features
SLT(2010)
Abstract
This paper proposes a set of approaches to automatically extract key terms from spoken course lectures including audio signals, ASR transcriptions and slides. We divide the key terms into two types: key phrases and keywords and develop different approaches to extract them in order. We extract key phrases using right/left branching entropy and extract keywords by learning from three sets of features: prosodic features, lexical features and semantic features from Probabilistic Latent Semantic Analysis (PLSA). The learning approaches include an unsupervised method (K-means exemplar) and two supervised ones (AdaBoost and neural network). Very encouraging preliminary results were obtained with a corpus of course lectures, and it is found that all approaches and all sets of features proposed here are useful.
MoreTranslated text
Key words
neural network,prosody,k-means,audio signals,speech recognition,learning (artificial intelligence),automatic key term extraction,asr transcriptions,keyword extraction,course lectures,semantic features,key phrase extraction,unsupervised method,adaboost,probabilistic latent semantic analysis,pat tree,audio signal processing,spoken course lectures,entropy,machine learning,lexical features,prosodic features,probabilistic latent semantic analysis (plsa),neural nets,probability,branching entropy,feature extraction,k means,markov processes,learning artificial intelligence,hidden markov models,semantics,artificial neural networks
AI Read Science
Must-Reading Tree
Example
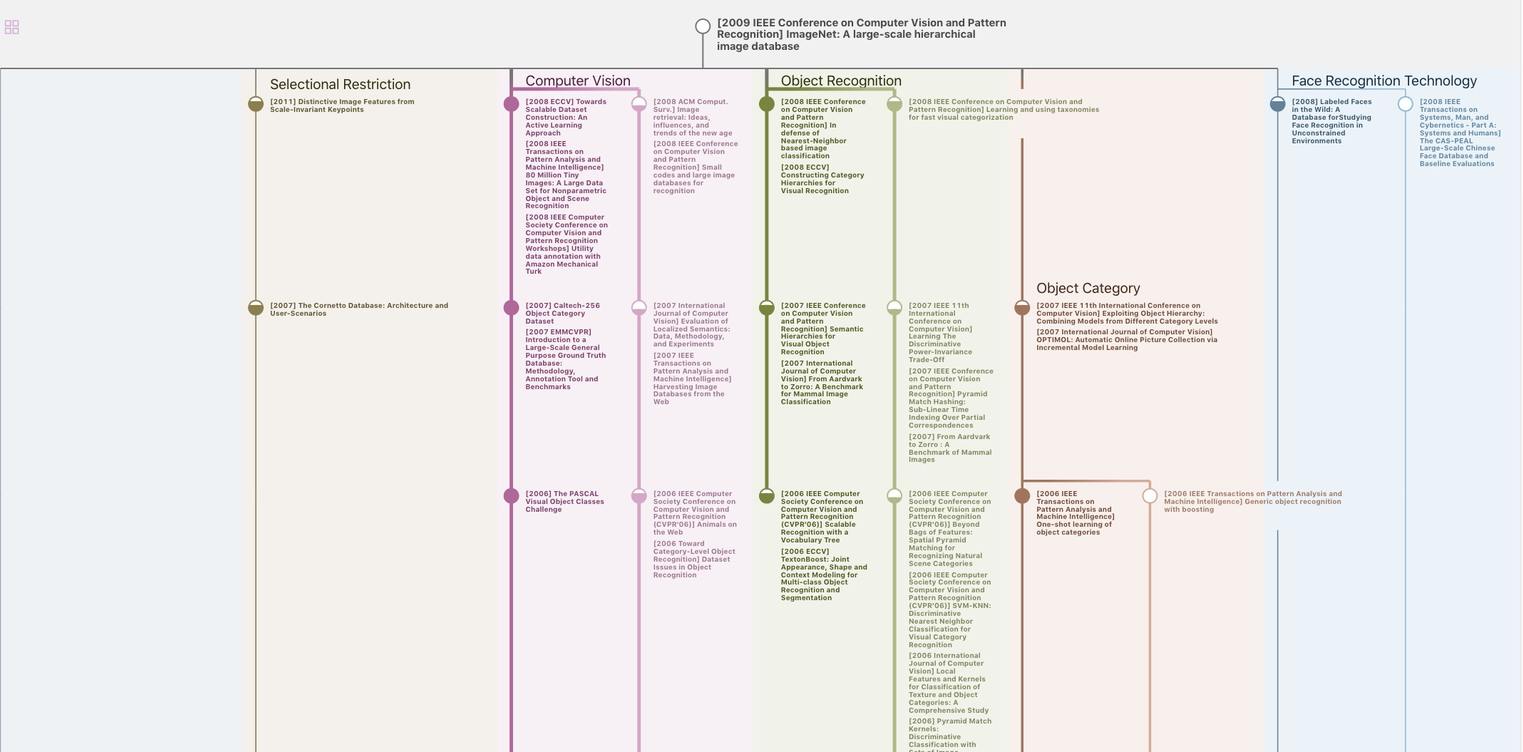
Generate MRT to find the research sequence of this paper
Chat Paper
Summary is being generated by the instructions you defined