A Dynamic Approach to Statistical Debugging: Building Program Specific Models with Neural Networks
msra(2007)
Abstract
Computer software is constantly increasing in complexity; this requires more de- veloper time, effort, and knowledge in order to correct bugs inevitably occurring in software production. Eventually, increases in complexity and size will make manually correcting programmatic errors impractical. Thus, there is a need for automated software-debugging tools that can reduce the time and effort required by the developer. The performance of previously developed debugging techniques can be greatly improved by combining them with machine-learning. Our research focuses on the application of neural networks within the domain of statistical de- bugging. Specifically, we develop methods to mine statistical debugging data that can then be used to train neural networks; these generated multi-layered neural networks can then be used to identify suspicious programmatic entities. Our devel- oped networks are generated on a per program basis in order to leverage specific programmatic properties. In our empirical evaluation we compare our proposed ap- proach with a state-of-the-art automated debugging technique. The results of the evaluation indicate that, for the cases considered, our approach is more effective than the considered technique.
MoreTranslated text
Key words
neural network,machine learning
AI Read Science
Must-Reading Tree
Example
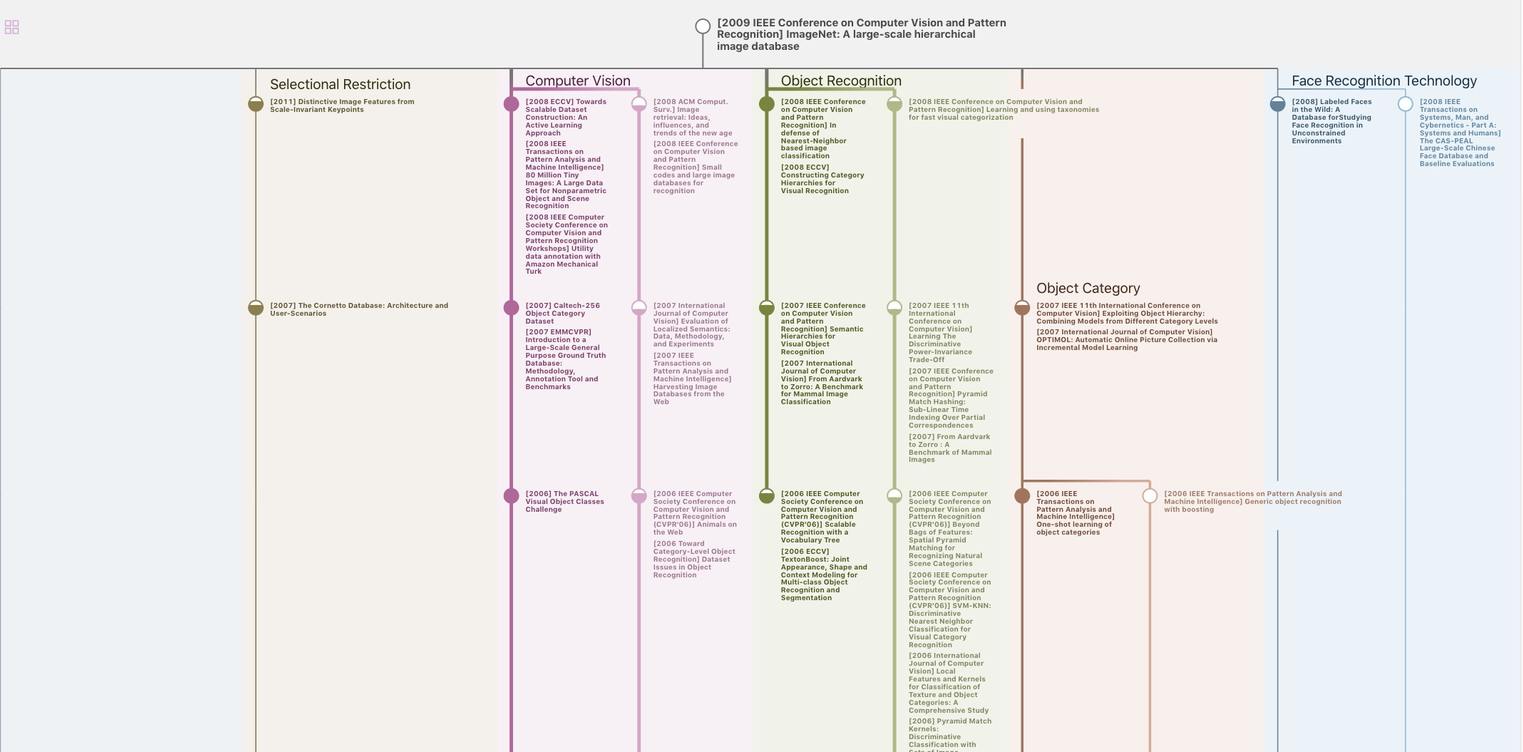
Generate MRT to find the research sequence of this paper
Chat Paper
Summary is being generated by the instructions you defined