A Generalization of SAT and #SAT for Robust Policy Evaluation.
IJCAI '13: Proceedings of the Twenty-Third international joint conference on Artificial Intelligence(2013)
摘要
Both SAT and #SAT can represent difficult problems in seemingly dissimilar areas such as planning, verification, and probabilistic inference. Here, we examine an expressive new language, #∃SAT, that generalizes both of these languages. #∃SAT problems require counting the number of satisfiable formulas in a concisely-describable set of existentially-quantified, propositional formulas. We characterize the expressiveness and worst-case difficulty of #∃SAT by proving it is complete for the complexity class # P NP [1] , and relating this class to more familiar complexity classes. We also experiment with three new general-purpose #∃SAT solvers on a battery of problem distributions including a simple logistics domain. Our experiments show that, despite the formidable worst-case complexity of # P NP [1] , many of the instances can be solved efficiently by noticing and exploiting a particular type of frequent structure.
更多查看译文
关键词
SAT problem,SAT solvers,complexity class,familiar complexity class,formidable worst-case complexity,expressive new language,worst-case difficulty,difficult problem,dissimilar area,frequent structure,robust policy evaluation
AI 理解论文
溯源树
样例
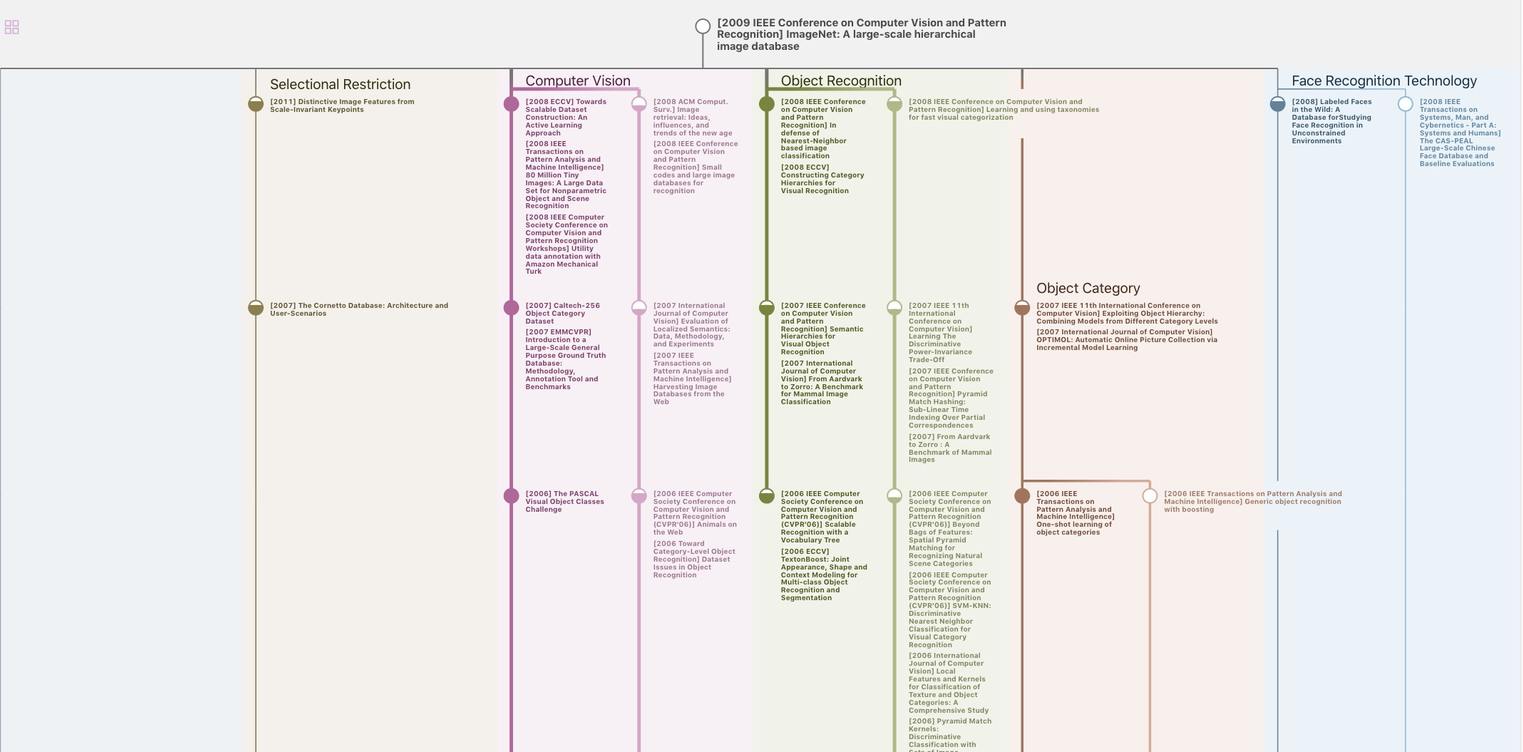
生成溯源树,研究论文发展脉络
Chat Paper
正在生成论文摘要