Two-stage framework for visualization of clustered high dimensional data
IEEE VAST(2009)
摘要
In this paper, we discuss dimension reduction methods for 2D visualization of high dimensional clustered data. We propose a two-stage framework for visualizing such data based on dimension reduction methods. In the first stage, we obtain the reduced dimensional data by applying a supervised dimension reduction method such as linear discriminant analysis which preserves the original cluster structure in terms of its criteria. The resulting optimal reduced dimension depends on the optimization criteria and is often larger than 2. In the second stage, the dimension is further reduced to 2 for visualization purposes by another dimension reduction method such as principal component analysis. The role of the second- stage is to minimize the loss of information due to reducing the dimension all the way to 2. Using this framework, we propose several two-stage methods, and present their theoretical characteristics as well as experimental comparisons on both artificial and real-world text data sets.
更多查看译文
关键词
principal component analysis,orthogonal centroid method,dimension reduction,generalized singular value decomposition index terms: h.5.2 information interfaces and pre- sentation: user interfaces—theory and methods,2d pro- jection,clustered data,regularization,linear discriminant analysis,indexing terms,data mining,user interface,optimization,vectors,upper bound,data visualization,data visualisation,high dimensional data,visual analytics
AI 理解论文
溯源树
样例
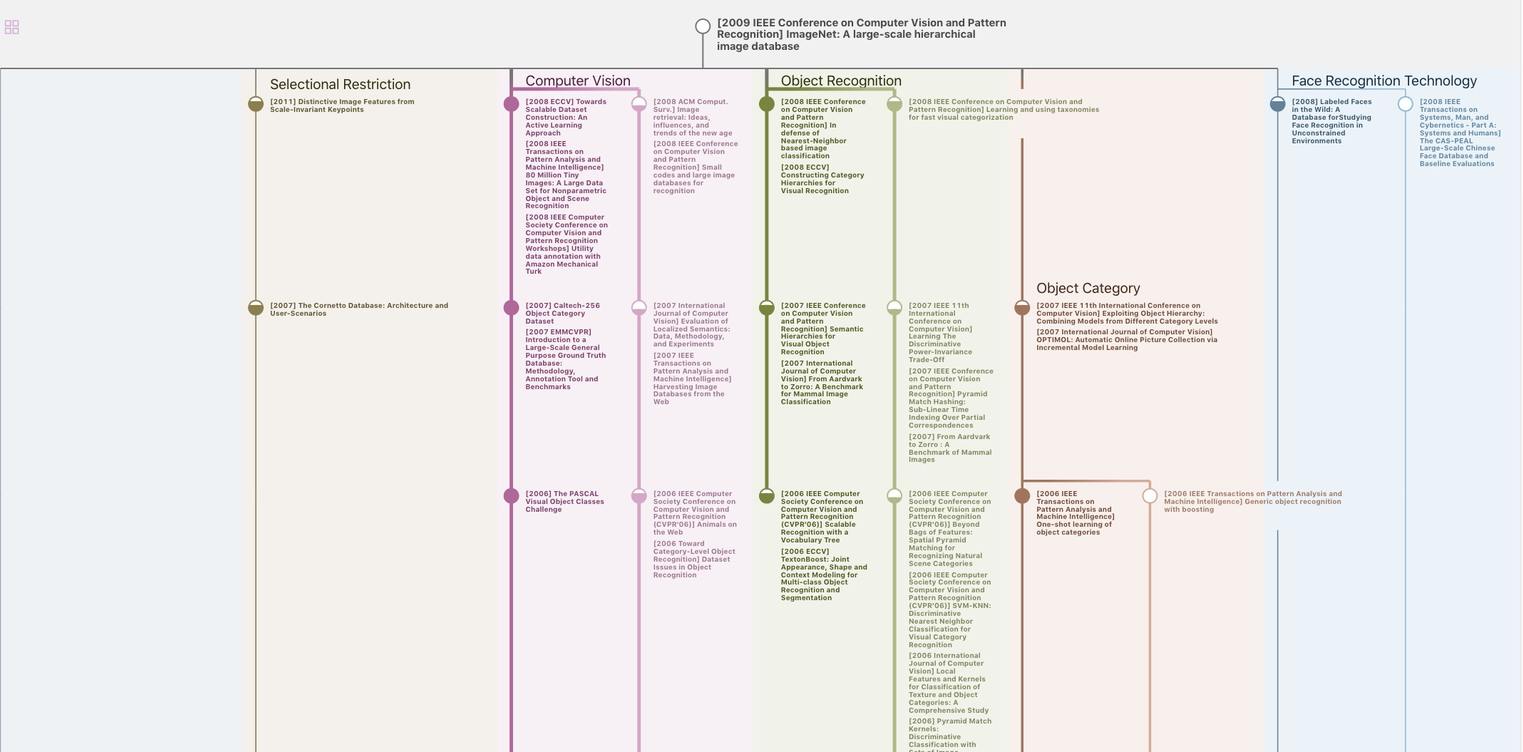
生成溯源树,研究论文发展脉络
Chat Paper
正在生成论文摘要