Analog Hardware Implementation Issues In Deterministic Boltzmann Machines
IEEE TRANSACTIONS ON CIRCUITS AND SYSTEMS II-ANALOG AND DIGITAL SIGNAL PROCESSING(1998)
摘要
The deterministic Boltzmann machine (DBM) is a neural network architecture that has a simple, local learning rule, making it an ideal candidate for implementation in massively parallel digital or analog VLSI hardware, The ability of the learning rule to compensate for hardware deficiencies simplifies a hardware implementation,This paper explores the effects of various nonideal analog hardware characteristics and explains which of these effects are automatically compensated for by the learning algorithm, and which must be carefully avoided during hardware design, It is found that moderate levels of most nonideal characteristics are tolerated well, However, in. the case of continuous learning of capacitive weights, the DBM cannot tolerate weight storage capacitor charge decay and offsets in the learning circuitry because they may cause the synaptic weight values to drift, resulting in oscillation, These effects can be alleviated by incorporating learning thresholds into the adaptation circuitry, at the expense of increased residual errors.
更多查看译文
关键词
analog VLSI circuits, artificial neural networks, Boltzmann machines
AI 理解论文
溯源树
样例
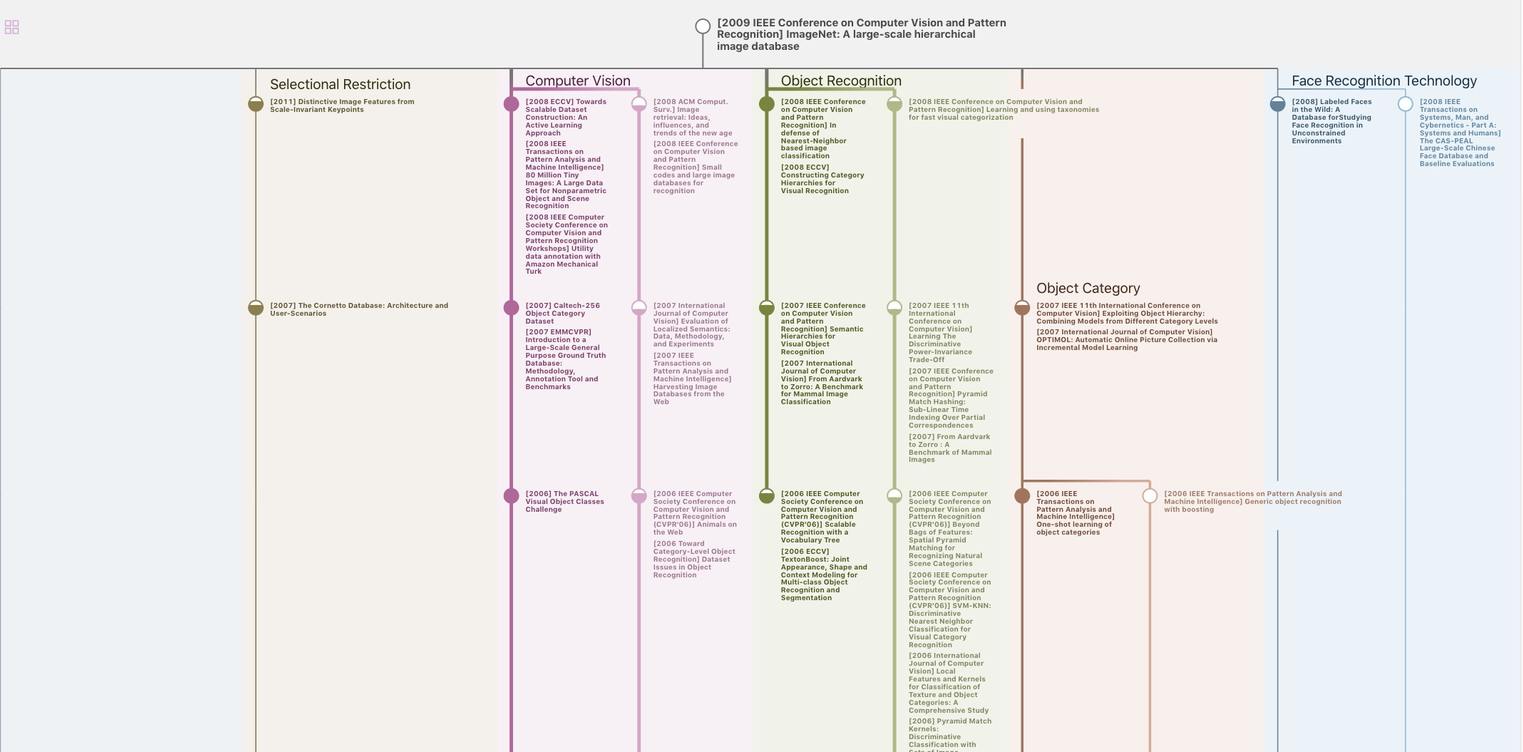
生成溯源树,研究论文发展脉络
Chat Paper
正在生成论文摘要