Transfer learning for behavioral targeting.
WWW(2010)
摘要
ABSTRACTRecently, Behavioral Targeting (BT) is attracting much attention from both industry and academia due to its rapid growth in online advertising market. Though a basic assumption of BT, which is, the users who share similar Web browsing behaviors will have similar preference over ads, has been empirically verified, we argue that the users' ad click preference and Web browsing behavior are not reflecting the same user intent though they are correlated. In this paper, we propose to formulate BT as a transfer learning problem. We treat the users' preference over ads and Web browsing behaviors as two different user behavioral domains and propose to utilize transfer learning strategy across these two user behavioral domains to segment users for BT ads delivery. We show that some classical BT solutions could be formulated in transfer learning view. As an example, we propose to leverage translated learning, which is a recent proposed transfer learning algorithm, to benefit the BT ads delivery. Experimental results on real ad click data show that, BT user segmentation by the approach of transfer learning can outperform the classical user segmentation strategies for larger than 20% in terms of smoothed ad Click Through Rate(CTR).
更多查看译文
AI 理解论文
溯源树
样例
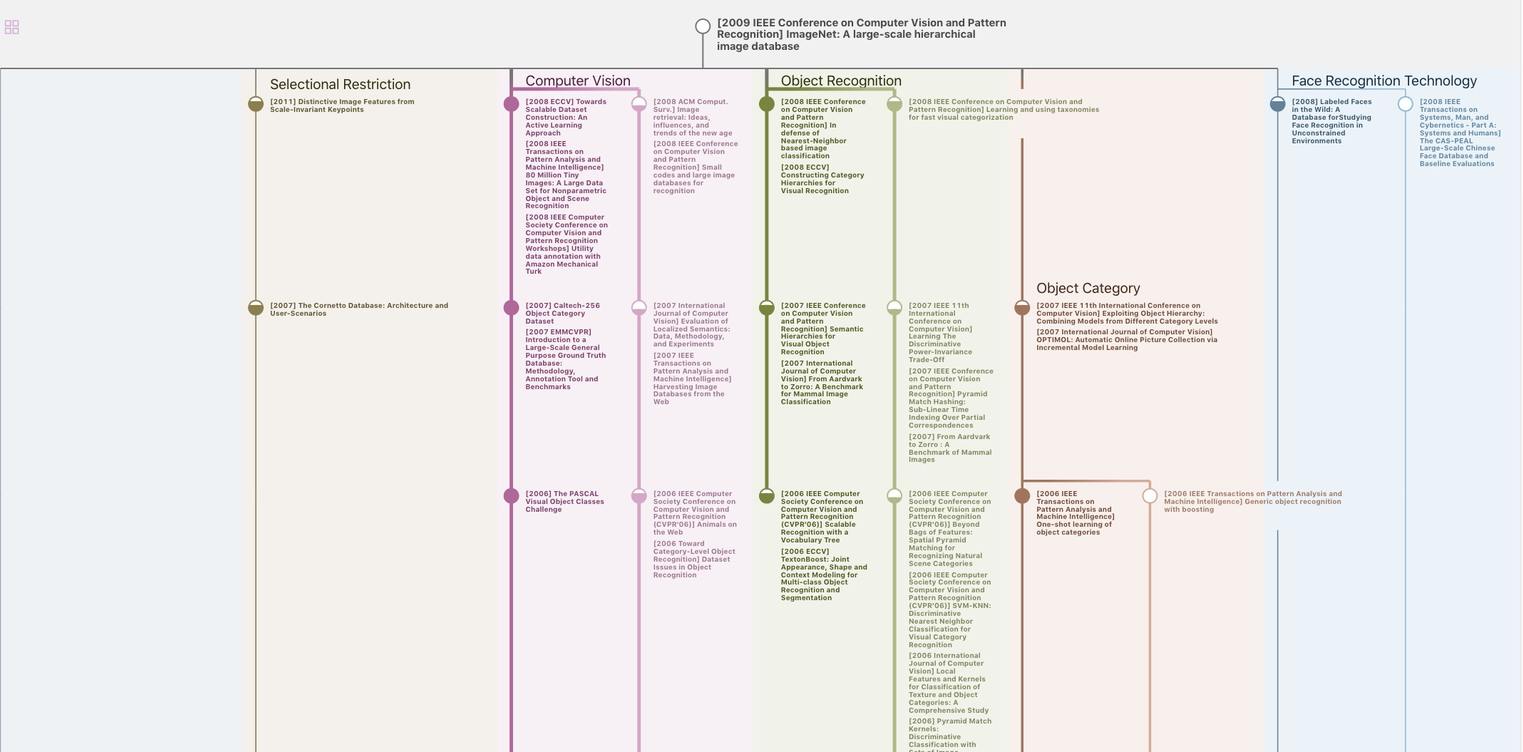
生成溯源树,研究论文发展脉络
Chat Paper
正在生成论文摘要