Searching through composite time series
msra(2007)
摘要
Recently there has been much interest in detecting anoma- lies in both categorical and real valued time series data. Many classical statistical methods deal with univariate data, and less has been done about multivariate data. The drastic decrease of data storage costs, availability of cheap sensors along with automation of systems have resulted in prolifera- tion of time series data. In most cases the data is multivari- ate in nature, and the eect of an anomaly can potentially be observed across more than one of these series. A traditional method to apply univariate methods in these cases would be to reduce the dimension using some dimensionality re- duction technique (eg PCA). But, an anomaly detected in a weighted linear combination of the data might not be mean- ingful to the end user. A majority of such techniques suers from the lack of user interpretability of the results. This motivates our approach of search through simple arithmetic combinations of time series. We compare the performance of our algorithm with related methods such as Vector Au- toregression on semi-synthetic health data.
更多查看译文
AI 理解论文
溯源树
样例
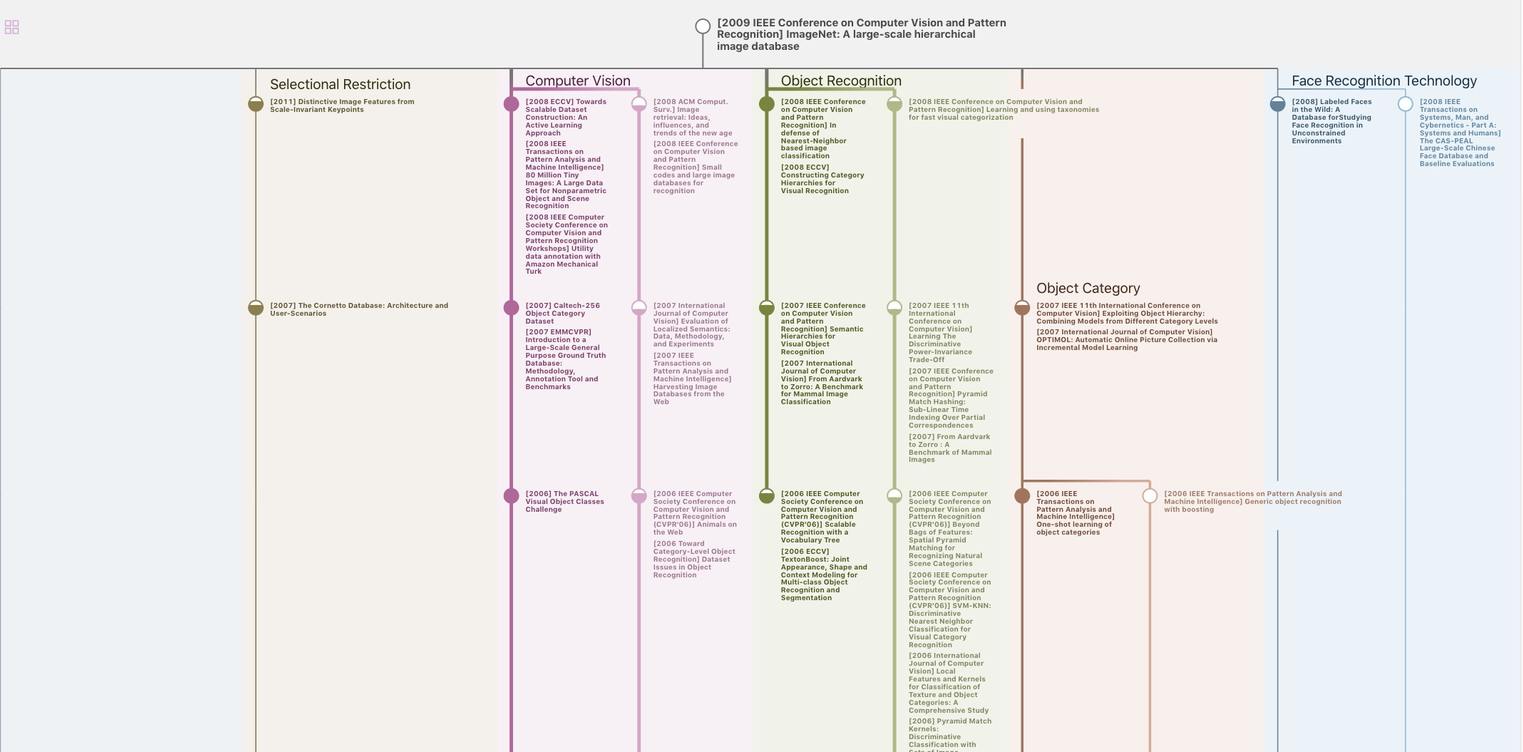
生成溯源树,研究论文发展脉络
Chat Paper
正在生成论文摘要