Sparse Unsupervised Dimensionality Reduction Algorithms
ECML PKDD'10: Proceedings of the 2010 European conference on Machine learning and knowledge discovery in databases: Part I(2010)
摘要
Principal component analysis (PCA) and its dual-principal coordinate analysis (PCO)-are widely applied to unsupervised dimensionality reduction. In this paper, we show that PCA and PCO can be carried out under regression frameworks. Thus, it is convenient to incorporate sparse techniques into the regression frameworks. In particular, we propose a sparse PCA model and a sparse PCO model. The former is to find sparse principal components, while the latter directly calculates sparse principal coordinates in a low-dimensional space. Our models can be solved by simple and efficient iterative procedures. Finally, we discuss the relationship of our models with other existing sparse PCA methods and illustrate empirical comparisons for these sparse unsupervised dimensionality reduction methods. The experimental results are encouraging.
更多查看译文
关键词
regression framework,existing sparse PCA method,sparse PCA model,sparse PCO model,sparse principal,sparse principal component,sparse technique,sparse unsupervised dimensionality reduction,principal component analysis,unsupervised dimensionality reduction
AI 理解论文
溯源树
样例
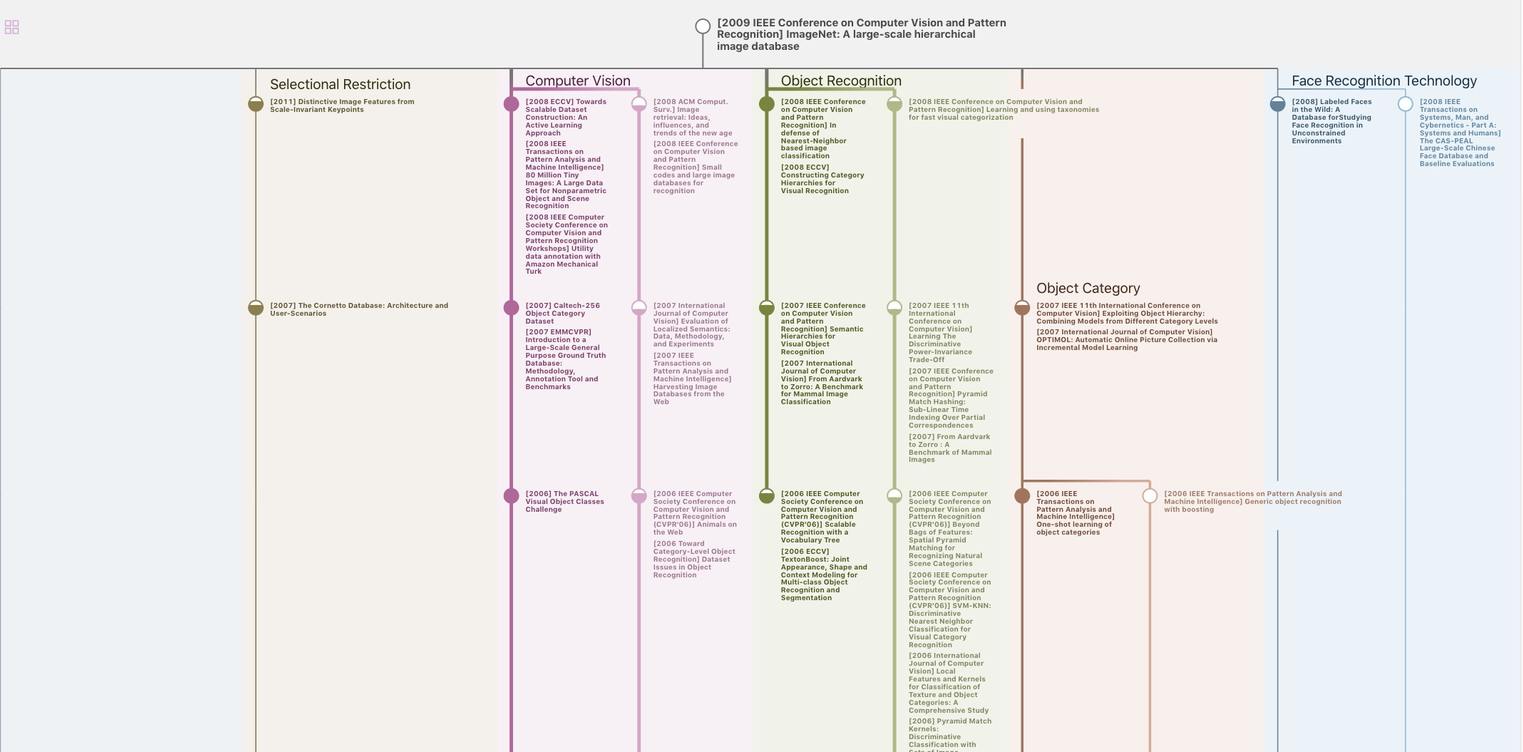
生成溯源树,研究论文发展脉络
Chat Paper
正在生成论文摘要