Supervised clustering with structural svms
Supervised clustering with structural svms(2009)
摘要
Supervised clustering is the problem of training clustering methods to produce desirable clusterings. Given sets of items and complete clusterings over these sets, a supervised clustering algorithm learns how to cluster future sets of items in a similar fashion, typically by changing the underlying similarity measure between item pairs. This work presents a general approach for training clustering methods such as correlation clustering and k-means/spectral clustering able to optimize to task-specific performance criteria using structural SVMs. We empirically and theoretically analyze our supervised clustering approach on a variety of datasets and clustering methods. This analysis also leads to general insights about structural SVMs beyond supervised clustering. Specifically, since clustering is a NP-hard task and the corresponding training problem likewise must make use of approximate inference during training of the parameters, we present a detailed theoretical and empirical analysis of the general use of approximations in structural SVM training.
更多查看译文
关键词
supervised clustering approach,Supervised clustering,structural SVMs,correlation clustering,clustering method,general approach,corresponding training problem,structural SVM training,general insight,structural svms,supervised clustering algorithm
AI 理解论文
溯源树
样例
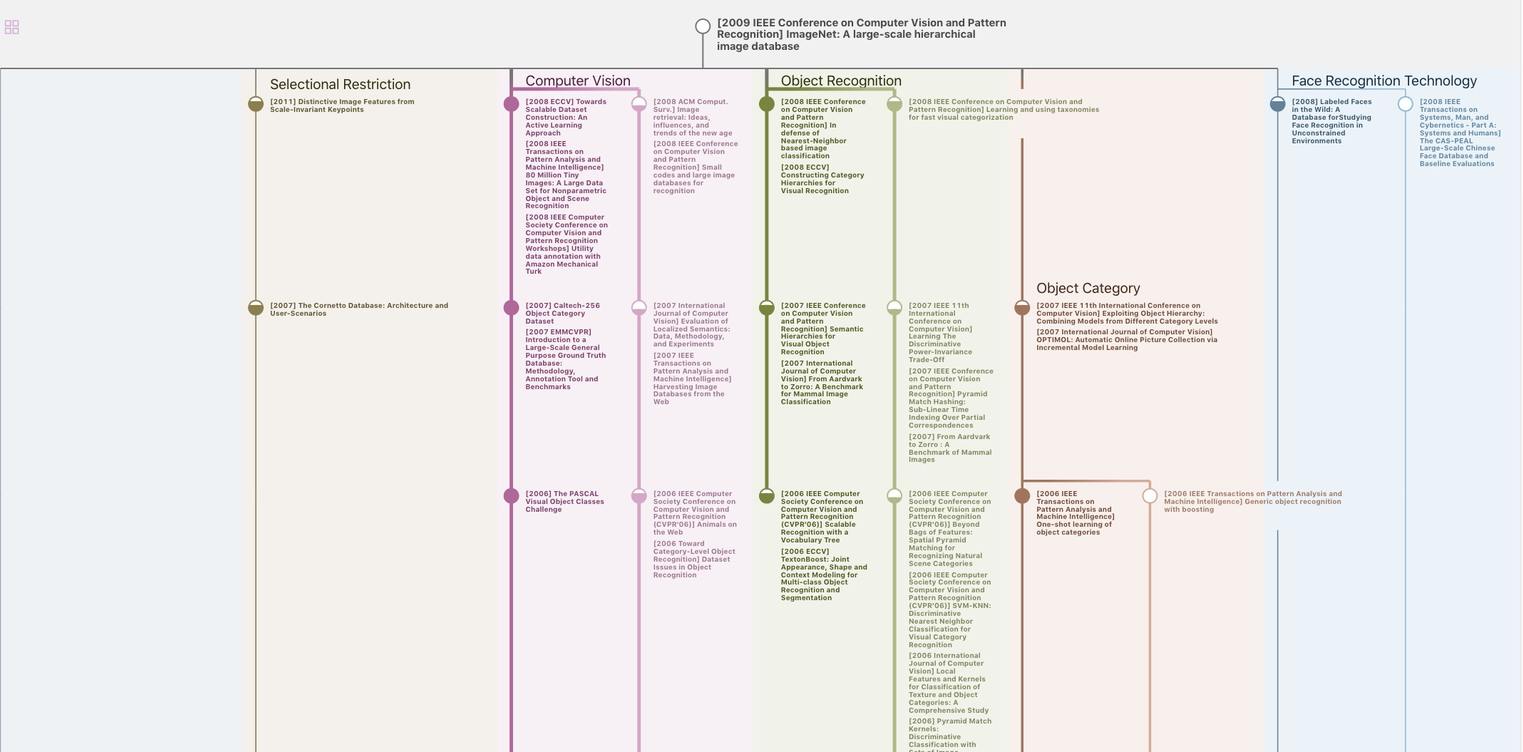
生成溯源树,研究论文发展脉络
Chat Paper
正在生成论文摘要