Sampling Methods for Unsupervised Learning
NIPS(2004)
摘要
We present an algorithm to overcome the local maxima problem in es- timating the parameters of mixture models. It combines existing ap- proaches from both EM and a robust tting algorithm, RANSAC, to give a data-driven stochastic learning scheme. Minimal subsets of data points, sufcient to constrain the parameters of the model, are drawn from pro- posal densities to discover new regions of high likelihood. The proposal densities are learnt using EM and bias the sampling toward promising solutions. The algorithm is computationally efcient, as well as effective at escaping from local maxima. We compare it with alternative methods, including EM and RANSAC, on both challenging synthetic data and the computer vision problem of alpha-matting.
更多查看译文
关键词
sampling methods,computer vision,unsupervised learning,synthetic data,mixture model
AI 理解论文
溯源树
样例
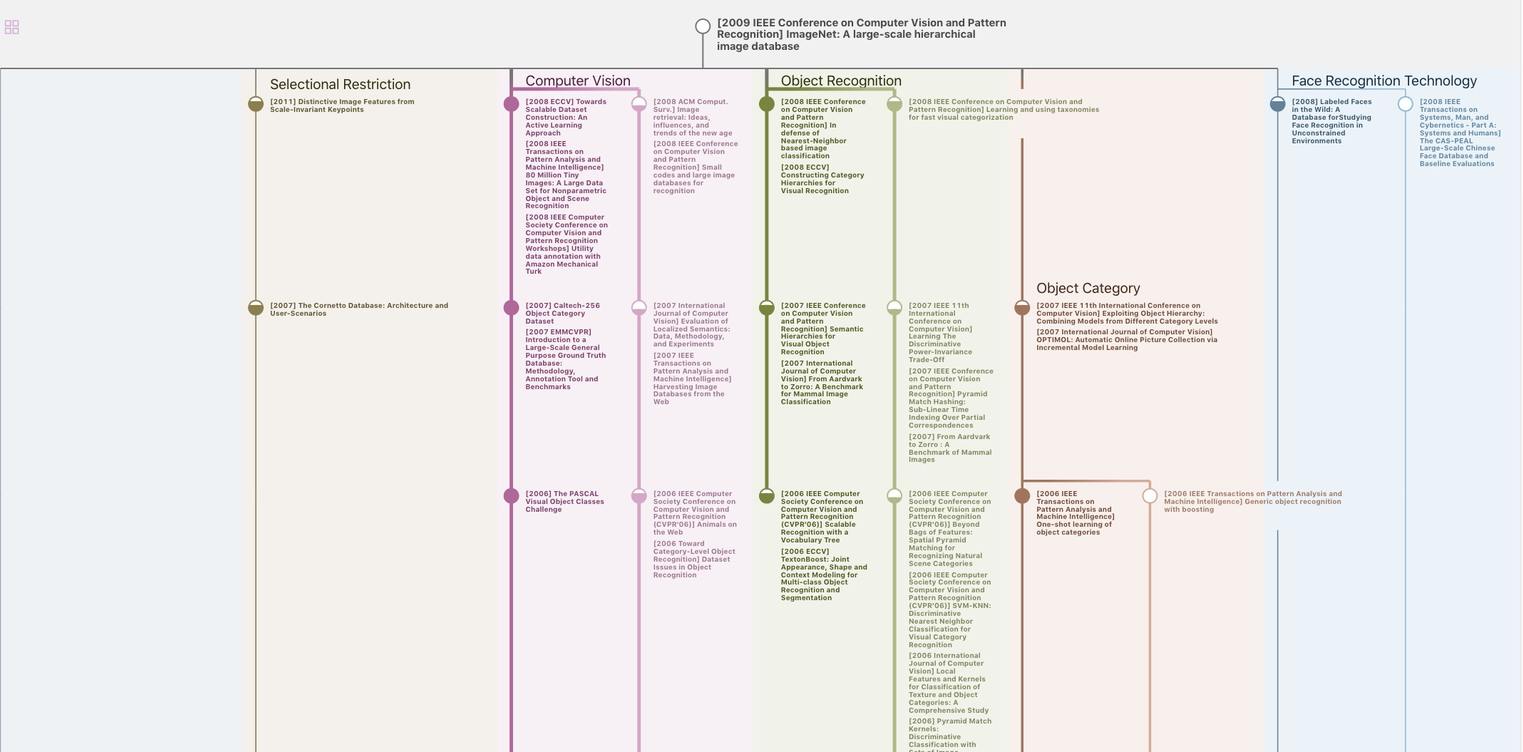
生成溯源树,研究论文发展脉络
Chat Paper
正在生成论文摘要