Partial orientation and local structural learning of causal networks for prediction
WCCI Causation and Prediction Challenge(2008)
摘要
For a prediction problem of a given target feature in a large causal network under ex- ternal interventions, we propose in this paper two partial orientation and local structural learning (POLSL) approaches, Local-Graph and PCD-by-PCD (where PCD denotes Par- ents, Children and some Descendants). The POLSL approaches are used to discover the local structure of the target and to orient edges connected to the target without discov- ering a global causal network. Thus they can greatly reduce computational complexity of structural learning and improve power of statistical tests. This approach is stimulated by the challenge problems proposed in IEEE World Congress on Computational Intelligence (WCCI2008) competition workshop. For the cases with and without external interventions, we select difierent feature sets to build prediction models. We apply the L1 penalized lo- gistic regression model to the prediction. For the case with noise and calibrant features in microarray data, we propose a two-stage fllter to correct global and local patterns of noise.
更多查看译文
关键词
partial orientation.,local structural learning,causal network,statistical test,microarray data,computational complexity,computational intelligence,regression model,prediction model
AI 理解论文
溯源树
样例
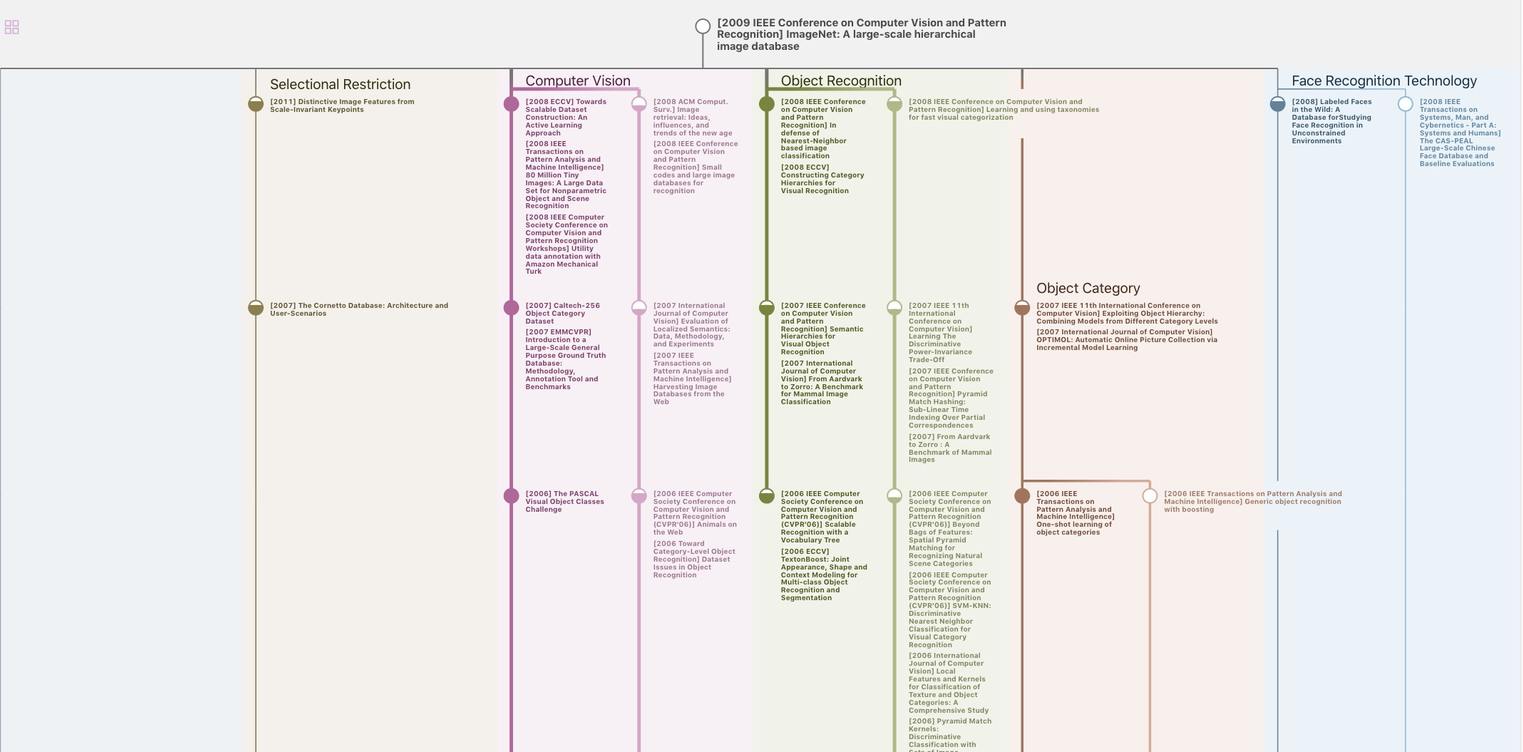
生成溯源树,研究论文发展脉络
Chat Paper
正在生成论文摘要