A Long-term Memory Approach for Dynamic Multiobjective Evolutionary Algorithms.
ECTA 2011/FCTA 2011: PROCEEDINGS OF THE INTERNATIONAL CONFERENCE ON EVOLUTIONARY COMPUTATION THEORY AND APPLICATIONS AND INTERNATIONAL CONFERENCE ON FUZZY COMPUTATION THEORY AND APPLICATIONS(2016)
摘要
A dynamic optimization problem (DOP) may involve two or more functions to be optimized simultaneously, as well as constraints and parameters which can be changed over time, it is essential to have a response approach to react when a change is detected. In the past, several memory-based approaches have been proposed in order to solve single-objective dynamic problems. Such approaches use a long-term memory to store the best known solution found so far before a change in the environment occurs, such that the solutions stored can be used as seeds in subsequent environments. However, when we deal with a Dynamic Multiobjective Problems with a Pareto-based evolutionary approach, it is natural to expect several traded-off solutions at each environment. Hence, it would be prohibitive to incorporate a memory-based methodology into it. In this paper, we propose a viable algorithm to incorporate a long-term memory into evolutionary multiobjective optimization approaches. Results indicate that the proposed approach is competitive with respect to two previously proposed dynamic multiobjective evolutionary approaches.
更多查看译文
关键词
Evolutionary algorithms,Dynamic multiobjective optimization
AI 理解论文
溯源树
样例
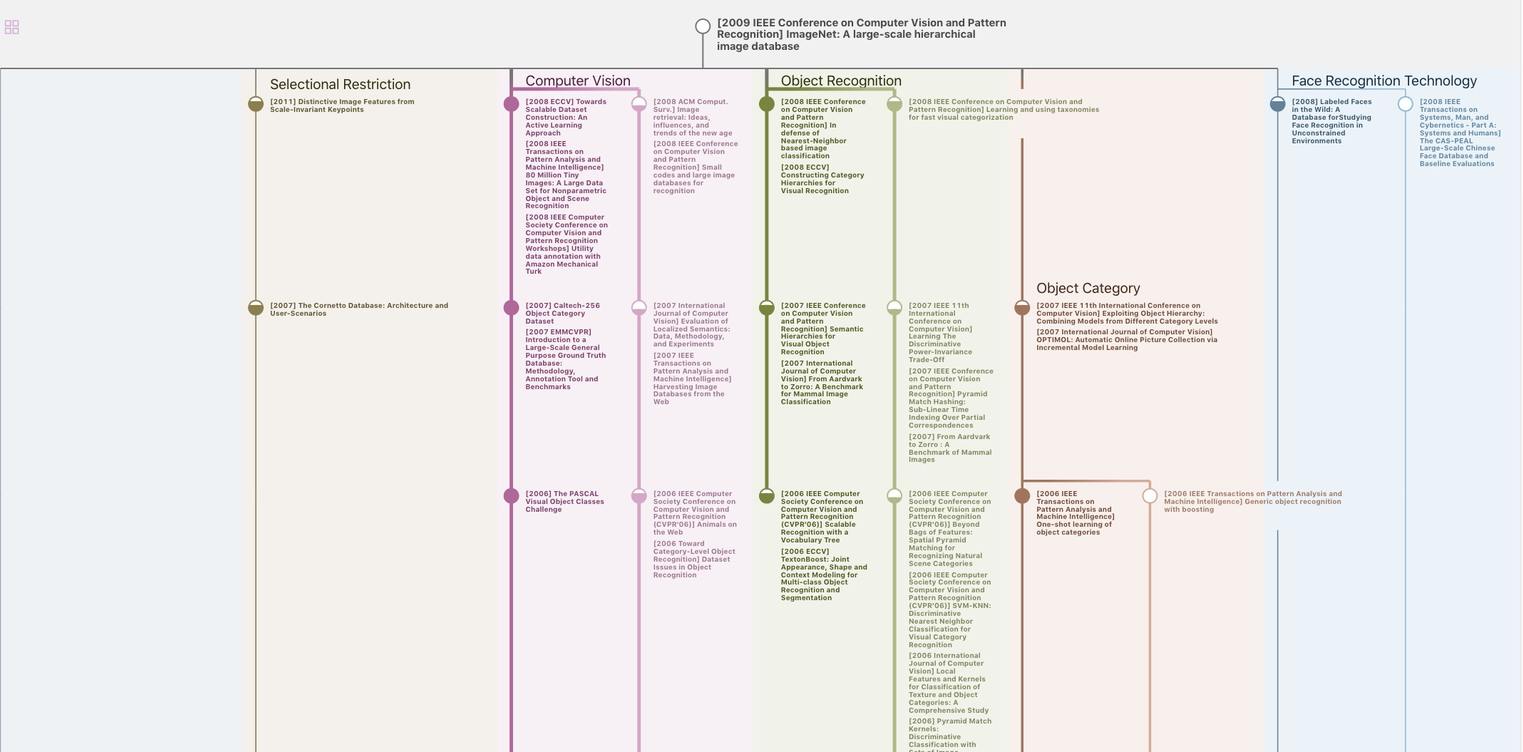
生成溯源树,研究论文发展脉络
Chat Paper
正在生成论文摘要