Contextual Hashing for Large-Scale Image Search
IEEE Transactions on Image Processing(2014)
摘要
With the explosive growth of the multimedia data on the Web, content-based image search has attracted considerable attentions in the multimedia and the computer vision community. The most popular approach is based on the bag-of-visual-words model with invariant local features. Since the spatial context information among local features is critical for visual content identification, many methods exploit the geometric clues of local features, including the location, the scale, and the orientation, for explicitly post-geometric verification. However, usually only a few initially top-ranked results are geometrically verified, considering the high computational cost in full geometric verification. In this paper, we propose to represent the spatial context of local features into binary codes, and implicitly achieve geometric verification by efficient comparison of the binary codes. Besides, we explore the multimode property of local features to further boost the retrieval performance. Experiments on holidays, Paris, and Oxford building benchmark data sets demonstrate the effectiveness of the proposed algorithm.
更多查看译文
关键词
benchmark data sets,multimedia computing,oxford,image coding,location,scale,geometric verification,spatial context modeling,high computational cost,computational geometry,visual content identification,hashing,web,bovw,bag-of-visual-words model,internet,spatial context information,feature extraction,content-based image search,image retrieval,invariant local features,file organisation,geometric clues,computer vision,orientation,large-scale image search,contextual hashing,binary codes,image search,multimedia data,paris,content-based retrieval,computer vision community,post-geometric verification,vectors,hamming distance,indexing,visualization
AI 理解论文
溯源树
样例
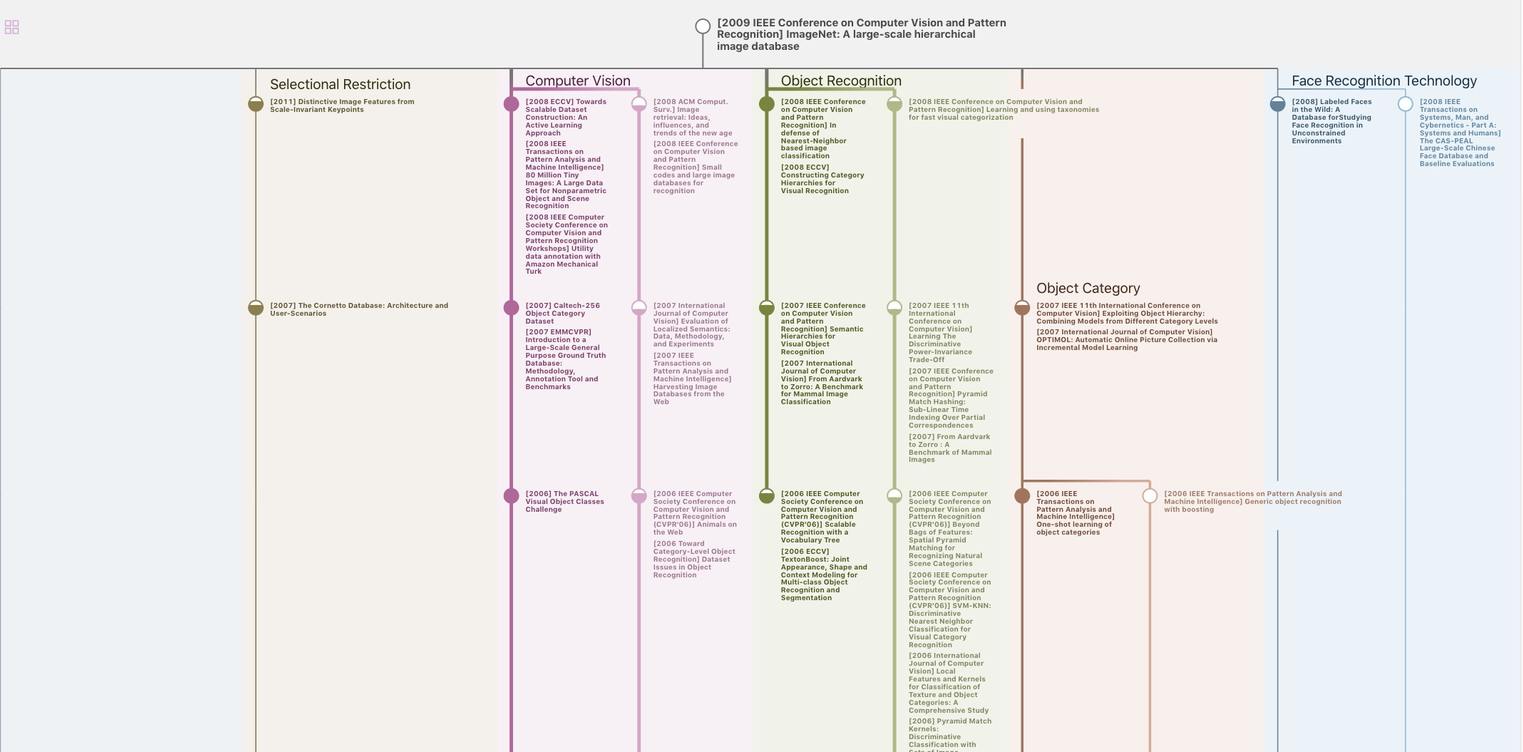
生成溯源树,研究论文发展脉络
Chat Paper
正在生成论文摘要