Forecasting using Decomposition and Combinations of Experts
ICAI '05: PROCEEDINGS OF THE 2005 INTERNATIONAL CONFERENCE ON ARTIFICIAL INTELLIGENCE, VOLS 1 AND 2(2005)
摘要
We study the effect of decomposing a series into multiple components and performing forecasts on each component separately. The focus here is on sales data - most of the series considered display both seasonality and trend. Hence the original series is decomposed into trend, seasonality and an irregular component. Multiple forecasting,experts' are used to forecast each component series. These range from different feedforward neural network topologies to Holt-Winter, ARIMA (of various orders) and double exponential smoothing. We compare the forecast errors with and without decomposition. We study the result of combining using the mean/median of all expert forecasts. Since our space of composite experts runs into the thousands, we experiment with more limited cardinalities using greedy elimination and best expert pair.
更多查看译文
关键词
time series forecasting,neural networks,genetic algorithms,decomposition,combining techniques
AI 理解论文
溯源树
样例
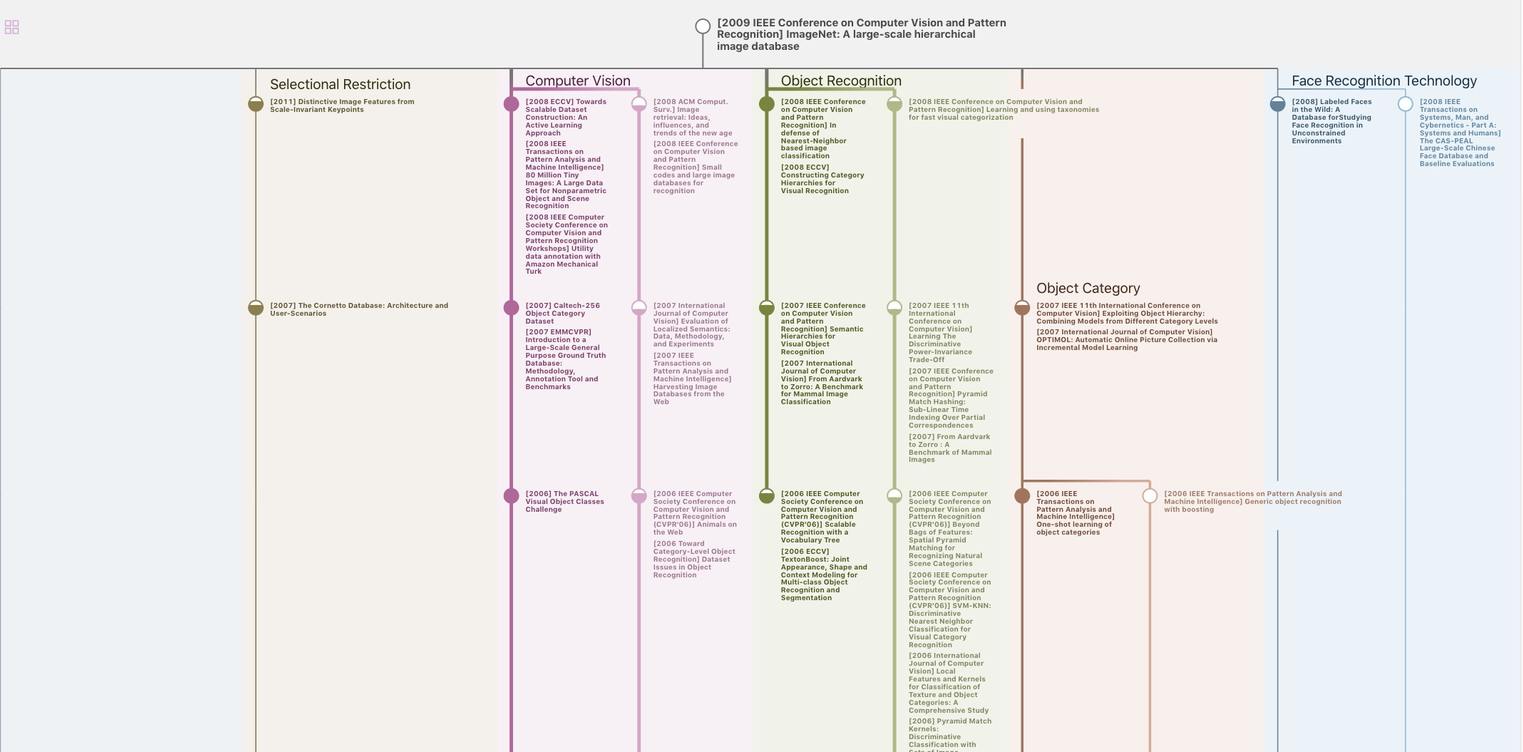
生成溯源树,研究论文发展脉络
Chat Paper
正在生成论文摘要