Designing Relevant Features For Continuous Data Sets Using Ica
INTERNATIONAL JOURNAL OF COMPUTATIONAL INTELLIGENCE AND APPLICATIONS(2008)
摘要
Isolating relevant information and reducing the dimensionality of the original data set are key areas of interest in pattern recognition and machine learning. In this paper, a novel approach to reducing dimensionality of the feature space by employing independent component analysis (ICA) is introduced. While ICA is primarily a feature extraction technique, it is used here as a feature selection/construction technique in a generic way. The new technique, called feature selection based on independent component analysis (FS ICA), efficiently builds a reduced set of features without loss in accuracy and also has a fast incremental version. When used as a first step in supervised learning, FS ICA outperforms comparable methods in efficiency without loss of classification accuracy. For large data sets as in medical image segmentation of high-resolution computer tomography images, FS ICA reduces dimensionality of the data set substantially and results in efficient and accurate classification.
更多查看译文
关键词
Feature subset selection, classification, HRCT, independent component analysis
AI 理解论文
溯源树
样例
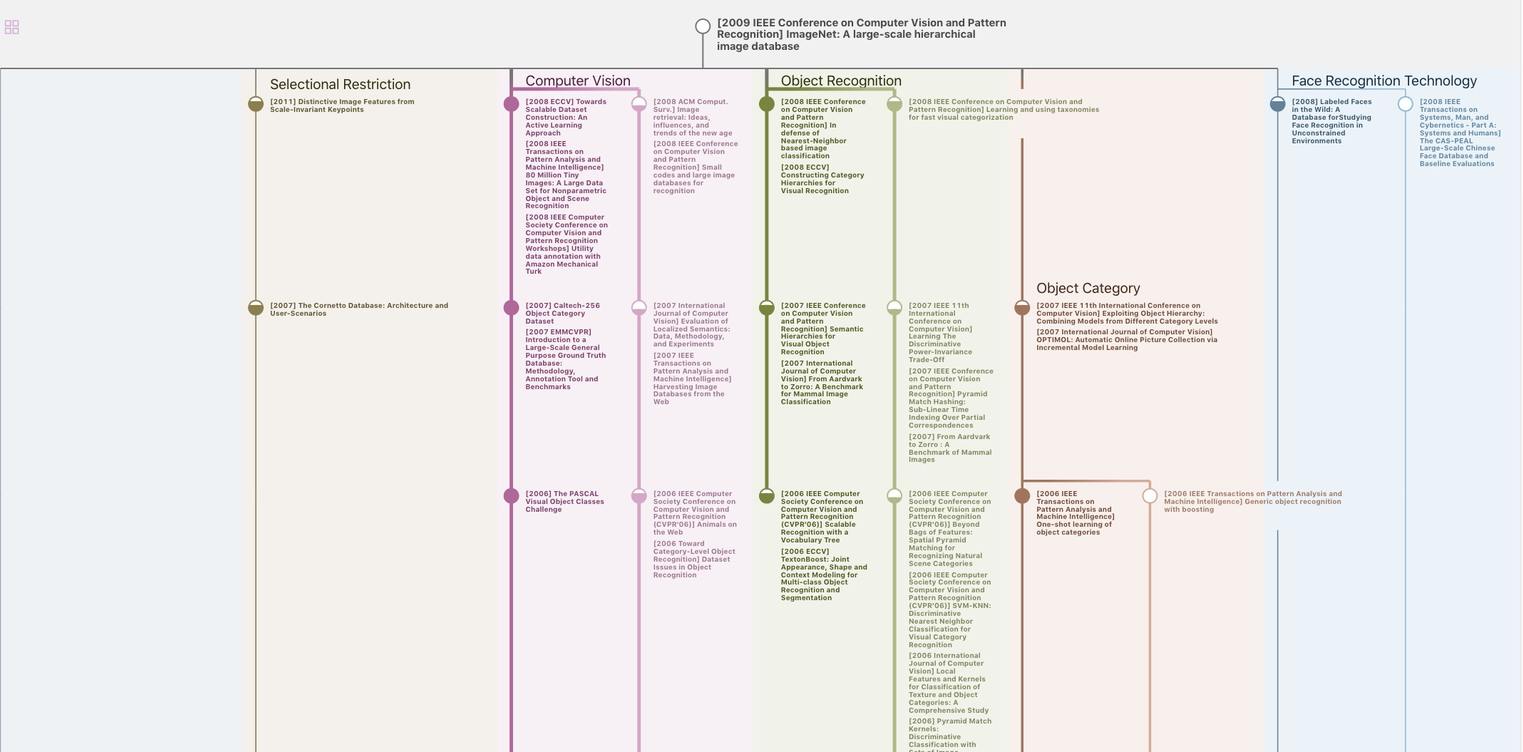
生成溯源树,研究论文发展脉络
Chat Paper
正在生成论文摘要