FedSpeed: Larger Local Interval, Less Communication Round, and Higher Generalization Accuracy
ICLR 2023(2023)
摘要
Federated learning (FL) is an emerging distributed machine learning framework which jointly trains a global model via a large number of local devices with data privacy protections. Its performance suffers from the non-vanishing biases introduced by the local inconsistent optimal and the rugged client-drifts by the local over-fitting. In this paper, we propose a novel and practical method, FedSpeed, to alleviate the negative impacts posed by these problems. Concretely, FedSpeed applies the prox-correction term on the current local updates to efficiently reduce the biases introduced by the prox-term, a necessary regularizer to maintain the strong local consistency. Furthermore, FedSpeed merges the vanilla stochastic gradient with a perturbation computed from an extra gradient ascent step in the neighborhood, thereby alleviating the issue of local over-fitting. Our theoretical analysis indicates that the convergence rate is related to both the communication rounds $T$ and local intervals $K$ with a tighter upper bound $\mathcal{O}(\frac{1}{T})$ if $K=\mathcal{O}(T)$. Moreover, we conduct extensive experiments on the real-world dataset to demonstrate the efficiency of our proposed FedSpeed, which converges significantly faster and achieves the state-of-the-art (SOTA) performance on the general FL experimental settings than several baselines including FedAvg, FedProx, FedCM, FedAdam, SCAFFOLD, FedDyn, FedADMM, etc.
更多查看译文
关键词
federated learning
AI 理解论文
溯源树
样例
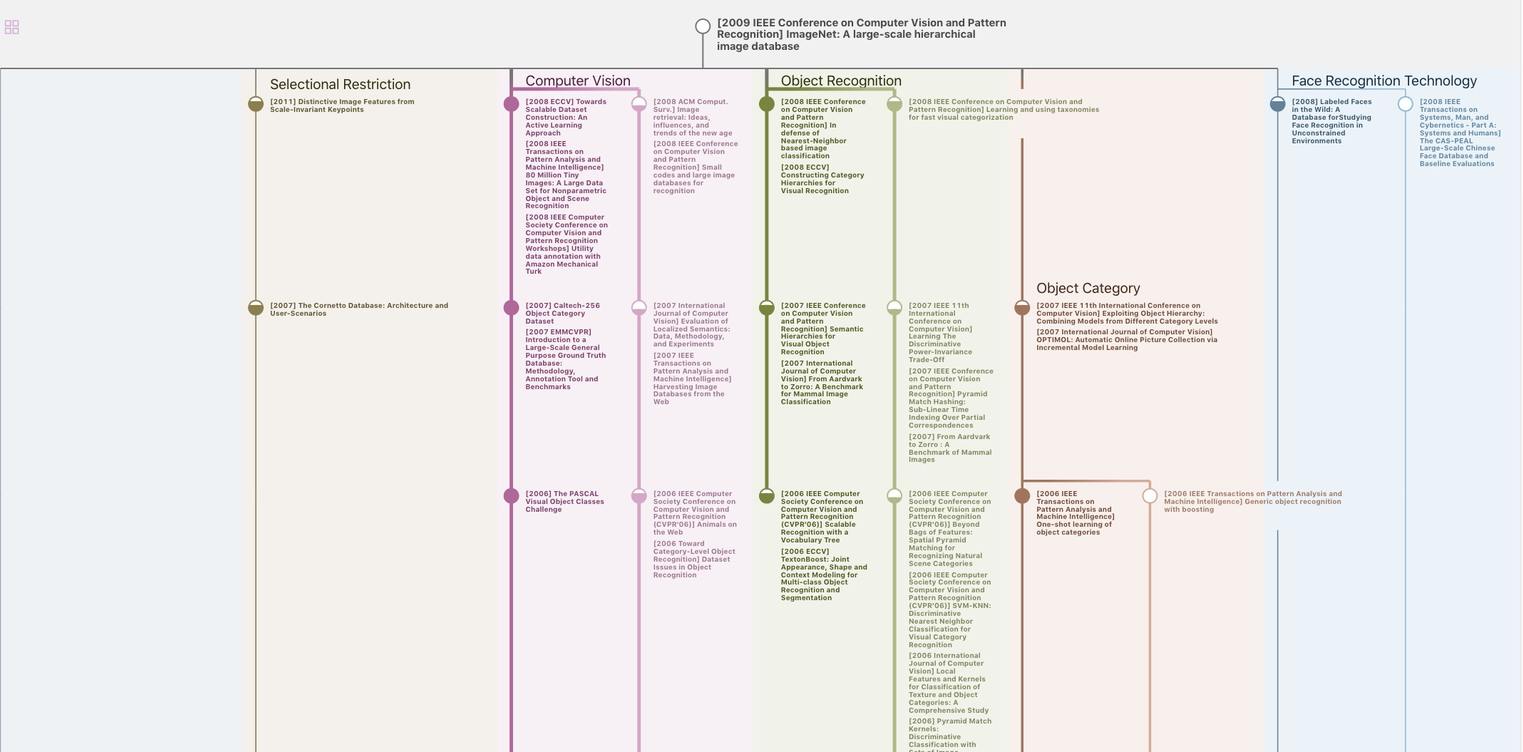
生成溯源树,研究论文发展脉络
Chat Paper
正在生成论文摘要