Bootstrapping through discrete convolutional methods
APPLIED STOCHASTIC MODELS IN BUSINESS AND INDUSTRY(2024)
摘要
Bootstrapping was designed to randomly resample data from a fixed sample using Monte Carlo techniques. However, the original sample itself defines a discrete distribution. Convolutional methods are well suited for discrete distributions, and we show the advantages of utilizing these techniques for bootstrapping. The discrete convolutional approach can provide exact numerical solutions for bootstrap quantities, or at least mathematical error bounds. In contrast, Monte Carlo bootstrap methods can only provide confidence intervals which converge slowly. Additionally, for some problems the computation time of the convolutional approach can be dramatically less than that of Monte Carlo resampling. This article provides several examples of bootstrapping using the proposed convolutional technique and compares the results to those of the Monte Carlo bootstrap, and to those of the competing saddlepoint method.
更多查看译文
关键词
convolution,degradation data,discrete Fourier transform,saddlepoint method
AI 理解论文
溯源树
样例
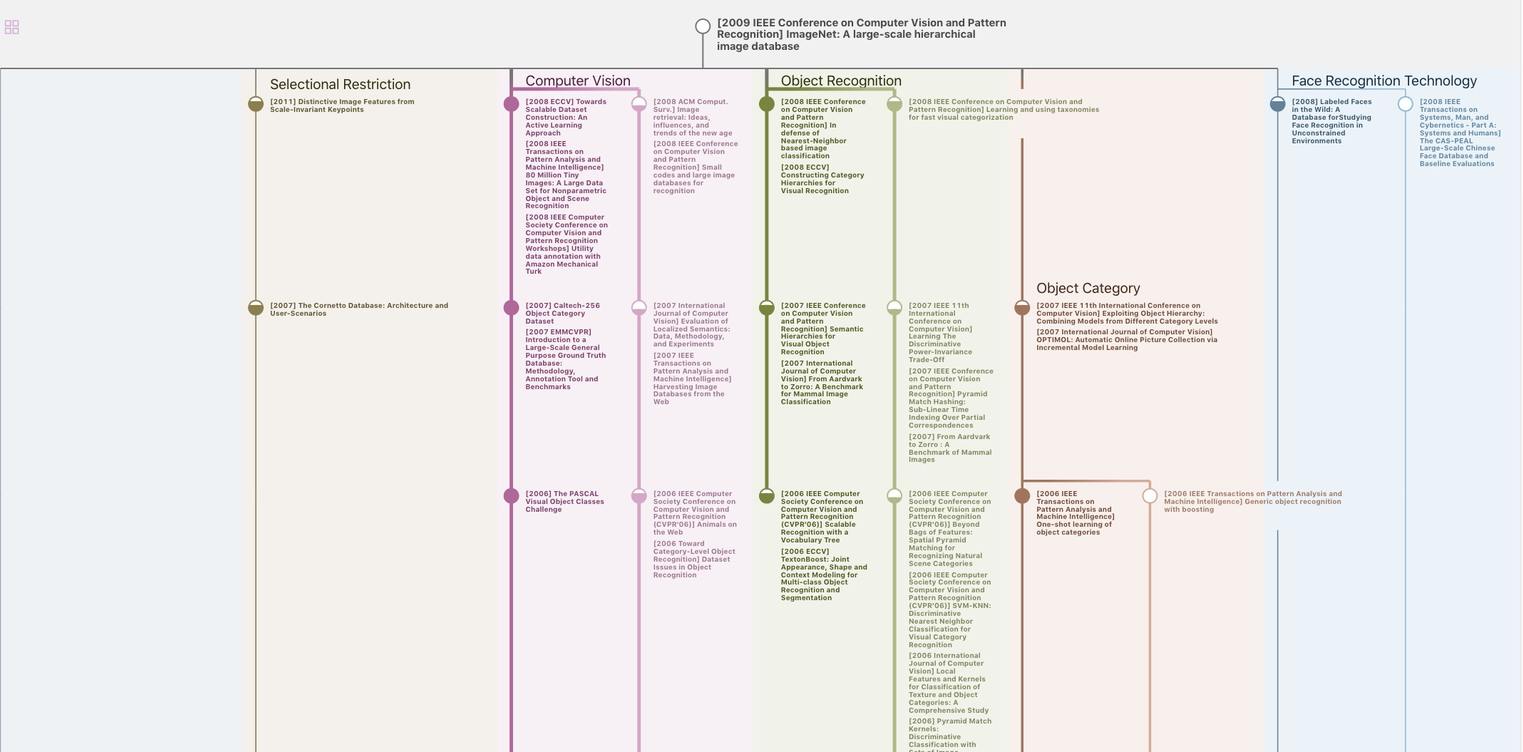
生成溯源树,研究论文发展脉络
Chat Paper
正在生成论文摘要