Identification of source components in multivariate time series by state space modelling
msra(2013)
摘要
In this paper we study the application of classical methods for dynamical modelling of time series to the task of decomposing multivariate time series into approximately independent source components, a task that has traditionally been addressed by Factor Analysis (FA) and more recently by Independent Component Analysis (ICA). Based on maximum-likelihood fitting of linear state space models we develop a new framework for this task, for which many of the limitations of standard ICA algorithms can be relieved. Through comparison of likelihood, or, more precisely, of the Akaike Information Criterion, it is demonstrated that dynamical modelling provides considerably better description of given data than FA and non-dynamical ICA. The comparison is applied to both simulated and real-world time series, the latter being given by an electrocardiogram and an electroencephalogram.
更多查看译文
关键词
whitening,independent component analysis,multivariate autoregressive modelling,time series analysis,kalman filtering,innovation approach,state space model,kalman filter,akaike information criterion,factor analysis,maximum likelihood,time series,state space
AI 理解论文
溯源树
样例
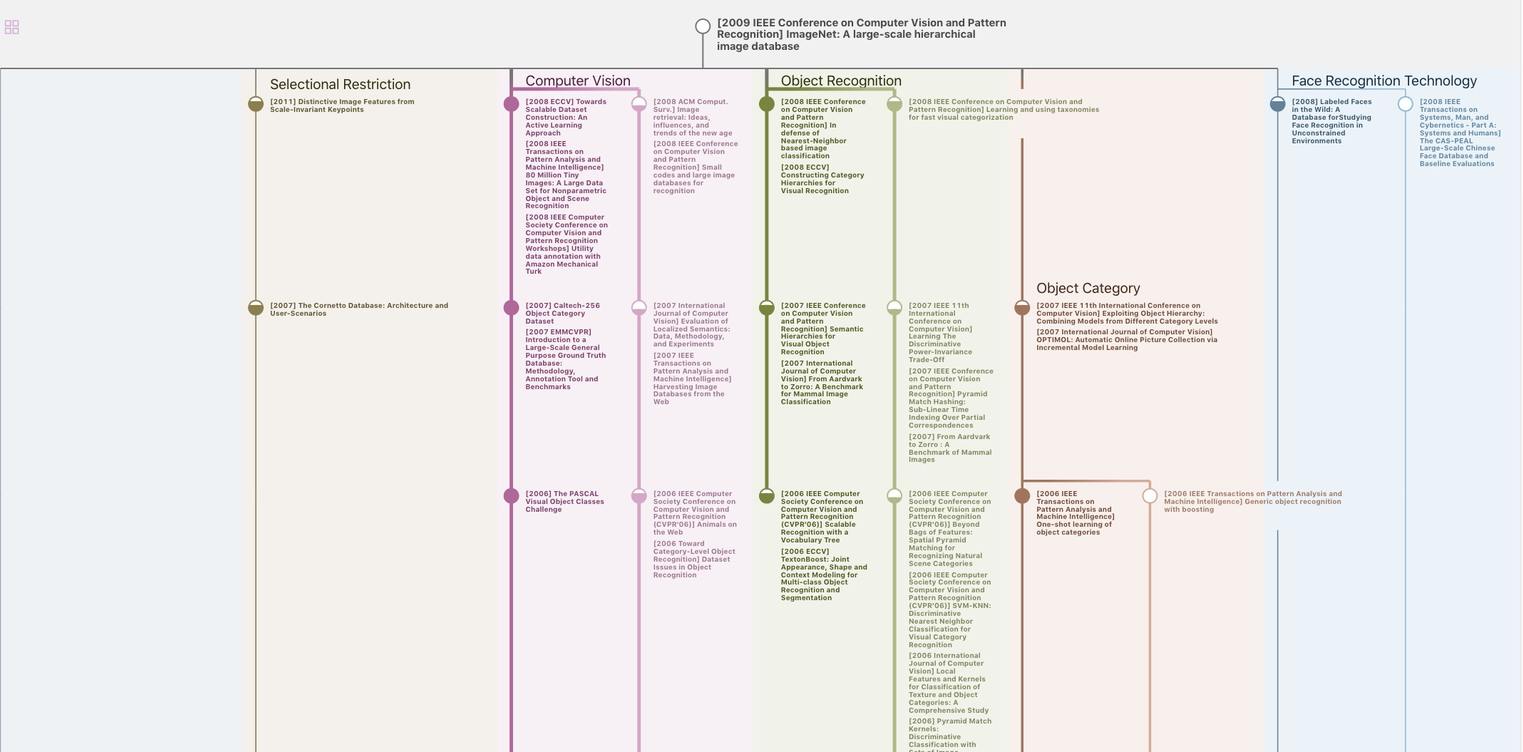
生成溯源树,研究论文发展脉络
Chat Paper
正在生成论文摘要