Parameter Tuning Boosts Performance Of Variation Operators In Multiobjective Optimization
PPSN'10: Proceedings of the 11th international conference on Parallel problem solving from nature: Part I(2010)
摘要
Typically, the variation operators deployed in evolutionary multiobjective optimization algorithms (EMOA) are either simulated binary crossover with polynomial mutation or differential evolution operators. This empirical study aims at the development of a sound method how to assess which of these variation operators perform best in the multiobjective context. In case of the S-metric selection EMOA our main findings are: (1) The performance of the tuned operators improved significantly compared to the default parameterizations. (2) The performance of the two tuned variation operators is very similar. (3) The optimized parameter configurations for the considered problems are very different.
更多查看译文
关键词
parameter tuning,performance assessment,benchmarking,inultiobjective variation operators,sequential parameter optimization
AI 理解论文
溯源树
样例
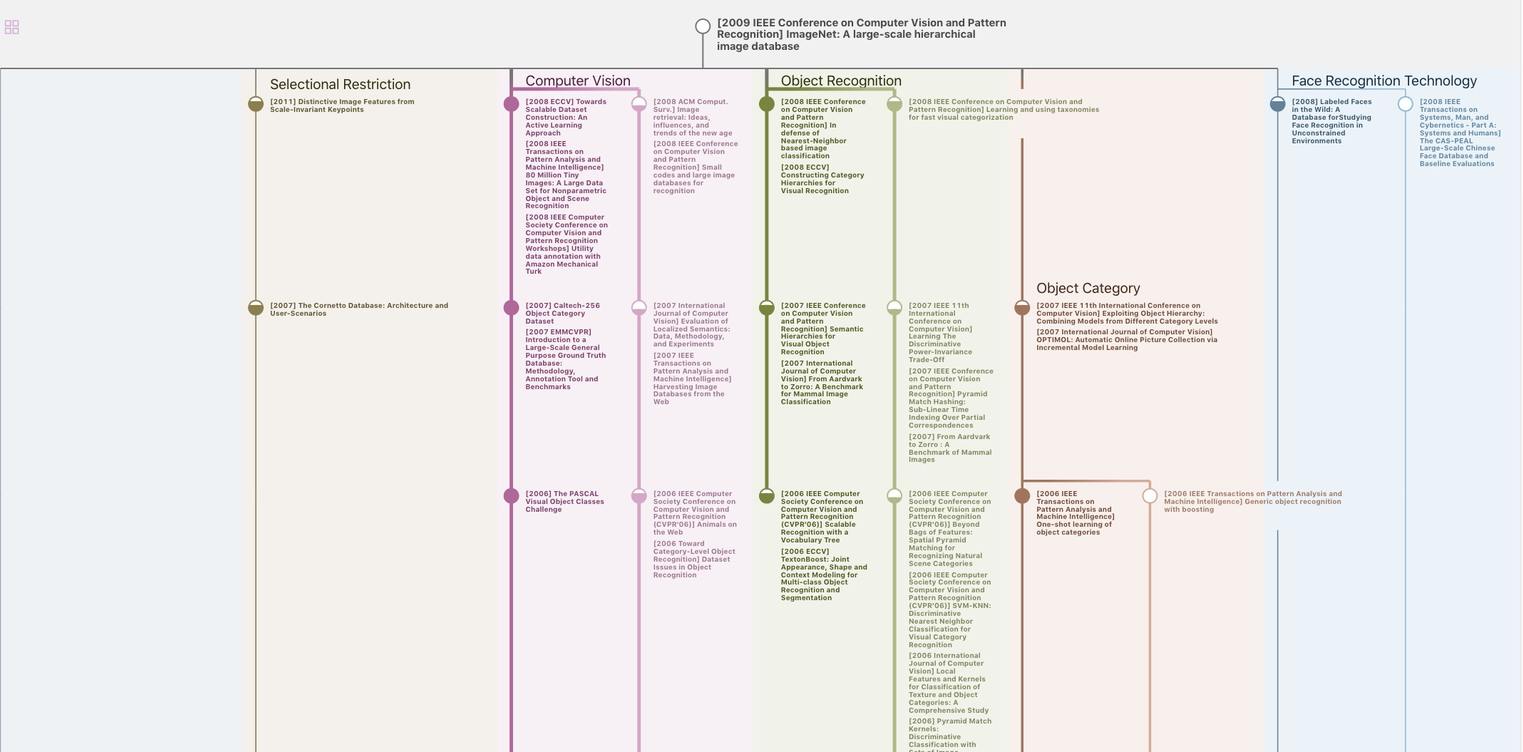
生成溯源树,研究论文发展脉络
Chat Paper
正在生成论文摘要