A Comparison Between Spiking And Differentiable Recurrent Neural Networks On Spoken Digit Recognition
PROCEEDINGS OF THE SECOND IASTED INTERNATIONAL CONFERENCE ON NEURAL NETWORKS AND COMPUTATIONAL INTELLIGENCE(2004)
摘要
In this paper we demonstrate that Long Short-Term Memory (LSTM) is a differentiable recurrent neural net (RNN) capable of robustly categorizing timewarped speech data. We measure its performance on a spoken digit identification task, where the data was spike-encoded in such a way that classifying the utterances became a difficult challenge in non-linear timewarping. We find that LSTM gives greatly superior results to an SNN found in the literature, and conclude that the architecture has a place in domains that require the learning of large timewarped datasets, such as automatic speech recognition.
更多查看译文
关键词
speech recognition,LSTM,RNN,SNN,timewarping
AI 理解论文
溯源树
样例
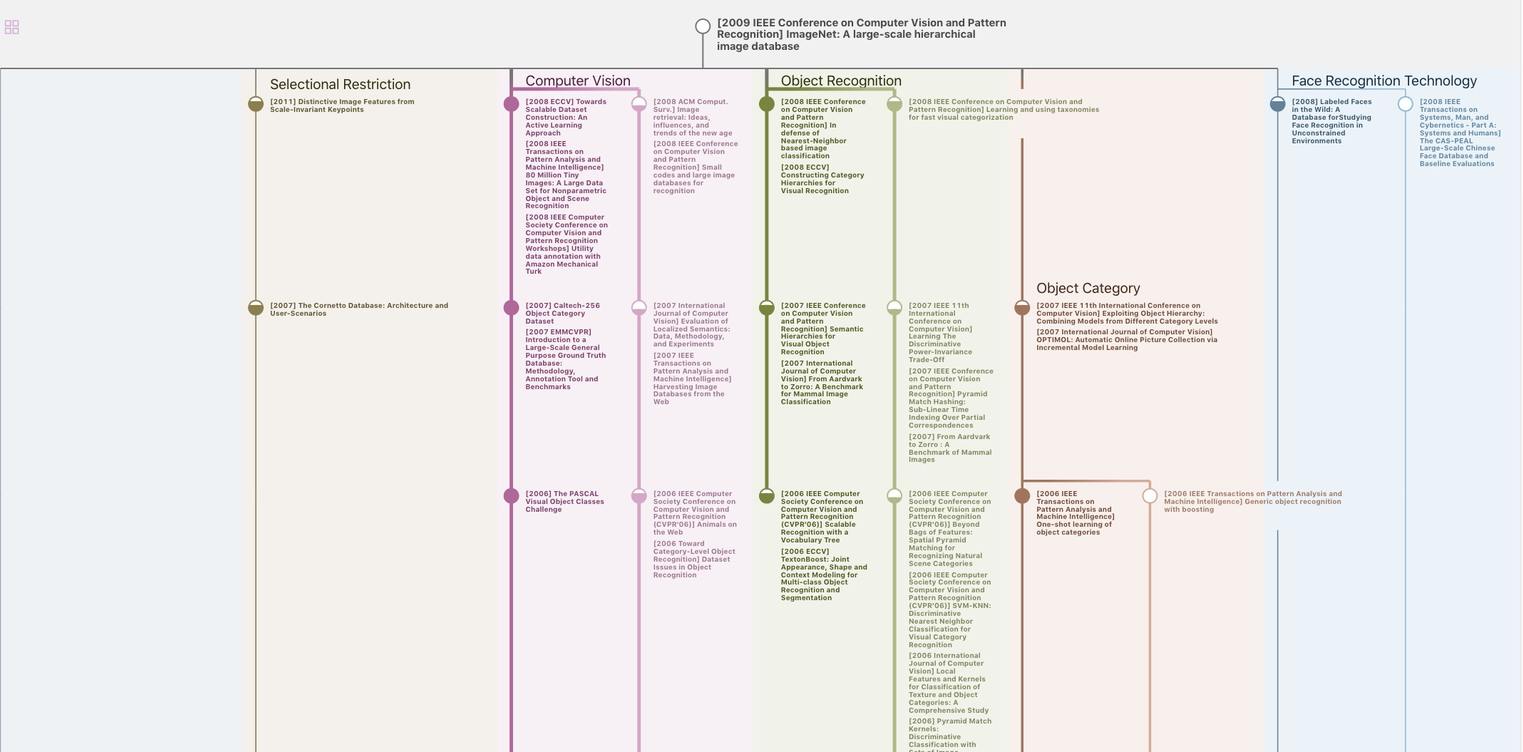
生成溯源树,研究论文发展脉络
Chat Paper
正在生成论文摘要