SU-GG-J-137: Application of Unsupervised Spectral Clustering in 3D for PET Tumor Segmentation: A Digital Phantom Study
MEDICAL PHYSICS(2010)
Abstract
Purpose:Spectralclustering refers to a class of techniques which rely on the eigenstructure of a similarity matrix to partition pixels into disjoint clusters. This study intended to investigate the feasibility of using the unsupervised spectralclustering method for PETtumor segmentation. Method and Materials: In this study a digital PET phantom was adopted as the source to generate PETimages with heterogeneous and/or irregular shaped tumors. The simulation was carried out by feeding the Zubal phantom as anatomical input to the SimSET (the Simulation System for Emission Tomography)software, which employs Monte Carlo techniques to model the underlying physical processes and imaging system to produce simulated emission tomography data. There were a total of 30 lung tumors with a variety of heterogeneous activities and/or irregular shapes simulated. The unsupervised spectralclustering algorithm was then applied to segment the simulated images. Segmentation accuracy was assessed with two metrics: volume detection error (VDE) and volume overlap measure (VOM). Results: The spectralclustering technique partitions the image volume into an optimal number of clusters according to the pair‐wise similarity measure between voxels. Upon segmentation, one can then select the segmented tumor regions for subsequent processing and analysis. Applying the spectralclustering technique on all the 30 simulated PETimages shows that VDE ranges from −5.5% to 6.2%, and VOM ranges from 0.94 to 1.Conclusion: This study shows the potential of the unsupervised 3D spectralclustering method for PETtumor segmentation. Segmentation performance in terms of VDE and VOM on stimulated PETimages shows this method may have the potential for accurate and consistent segmentation of tumors. Compared with thresholding‐based PETtumor delineation techniques, it segments the PETimage without requiring any threshold‐volume relationship. Studies to further evaluate this method on patient data are planned.
MoreTranslated text
Key words
spectral clustering
AI Read Science
Must-Reading Tree
Example
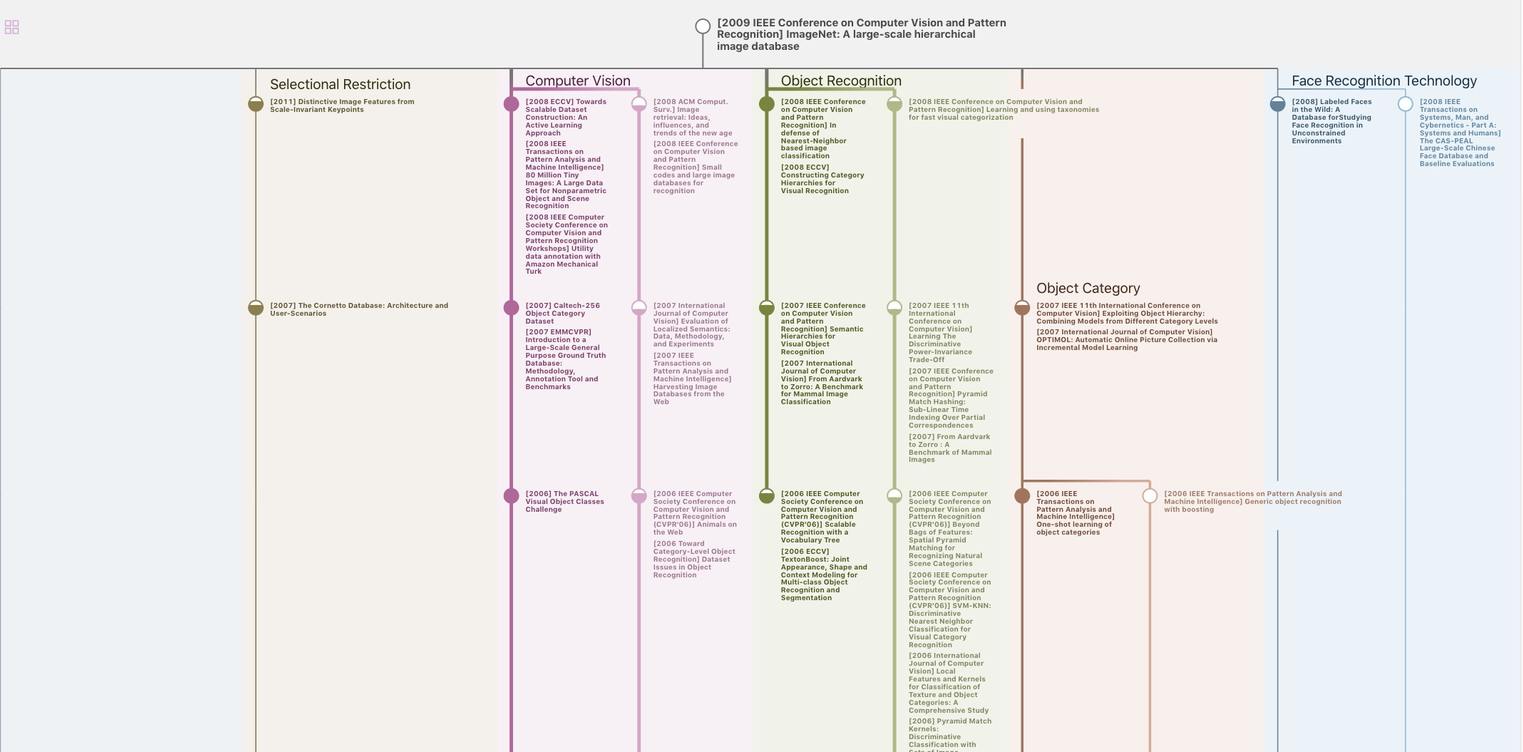
Generate MRT to find the research sequence of this paper
Chat Paper
Summary is being generated by the instructions you defined