Discriminating between Long Memory and Volatility Shifts
Austrian Journal of Statistics(2016)
摘要
We develop a practical implementation of the test proposed in Berkes, Horvath, Kokoszka, and Shao (2006) designed to distinguish be- tween a change-point model and a long memory model. Our implementa- tion is calibrated to distinguish between a shift in volatility of returns and long memory in squared returns. It uses a kernel estimator of the long-run variance of squared returns with the maximal lag selected by a data driven procedure which depends on the sample size, the location of the estimated change point and the direction of the apparent volatility shift (increase versus decrease). In a simulations study, we also consider other long-run variance estimators, including the VARHAC estimator, but we find that they lead to tests with inferior performance. Applied to returns on indexes and individual stocks, our test indicates that even for the same asset, a change-point model may be preferable for a certain period of time, whereas there is evidence of long memory in another period of time. Generally there is stronger evidence for long memory in the eight years ending June 2006 than in the eight years starting January 1992. This pattern is most pronounced for US stock indexes and shares in the US financial sector. Zusammenfassung: Wir entwickeln die praktische Umsetzung des in Berkes et al. (2006) vorgeschlagen Tests zur Unterscheidung zwischen einem Change- Point und einem Long Memory Modell. Unsere Implementierung ist darauf abgestimmt, zwischen einer Verschiebung in der Volatilitat von Returns und Long Memory in quadrierten Returns zu unterscheiden und verwendet einen Kernsch¨ atzer der Long-Run Varianz der quadrierten Returns. Dabei wird der maximale Lag durch eine datengesteuerte Prozedur gew¨ ahlt, die vom
更多查看译文
关键词
volatility of returns.,change-point,long memory,garch
AI 理解论文
溯源树
样例
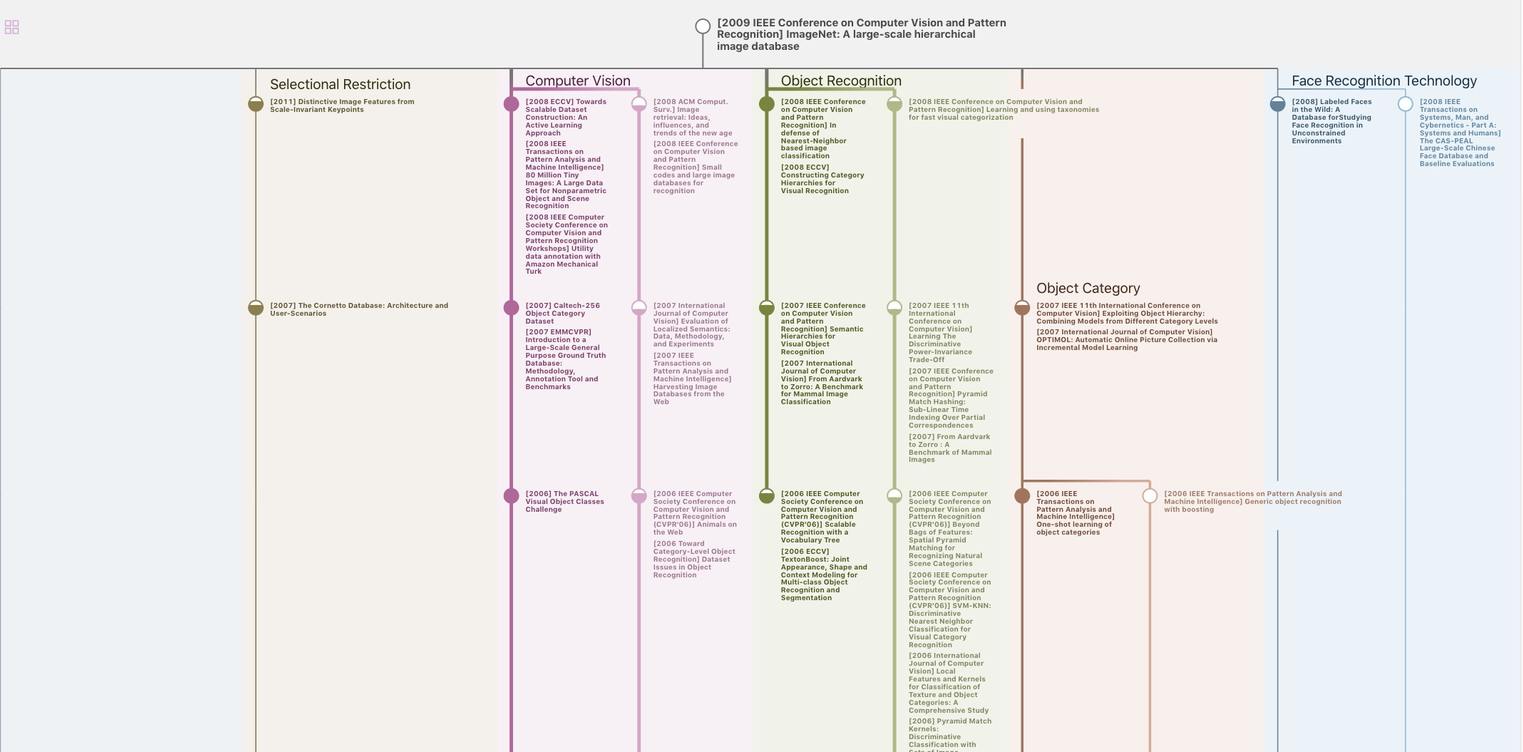
生成溯源树,研究论文发展脉络
Chat Paper
正在生成论文摘要