Automatic detection of omissions in medication lists.
JOURNAL OF THE AMERICAN MEDICAL INFORMATICS ASSOCIATION(2011)
摘要
Objective Evidence suggests that the medication lists of patients are often incomplete and could negatively affect patient outcomes. In this article, the authors propose the application of collaborative filtering methods to the medication reconciliation task. Given a current medication list for a patient, the authors employ collaborative filtering approaches to predict drugs the patient could be taking but are missing from their observed list. Design The collaborative filtering approach presented in this paper emerges from the insight that an omission in a medication list is analogous to an item a consumer might purchase from a product list. Online retailers use collaborative filtering to recommend relevant products using retrospective purchase data. In this article, the authors argue that patient information in electronic medical records, combined with artificial intelligence methods, can enhance medication reconciliation. The authors formulate the detection of omissions in medication lists as a collaborative filtering problem. Detection of omissions is accomplished using several machine-learning approaches. The effectiveness of these approaches is evaluated using medication data from three long-term care centers. The authors also propose several decision-theoretic extensions to the methodology for incorporating medical knowledge into recommendations. Results Results show that collaborative filtering identifies the missing drug in the top-10 list about 40-50% of the time and the therapeutic class of the missing drug 50%-65% of the time at the three clinics in this study. Conclusion Results suggest that collaborative filtering can be a valuable tool for reconciling medication lists, complementing currently recommended process-driven approaches. However, a one-size-fits-all approach is not optimal, and consideration should be given to context (eg, types of patients and drug regimens) and consequence leg, the impact of omission on outcomes).
更多查看译文
关键词
collaborative filtering,negative affect,machine learning
AI 理解论文
溯源树
样例
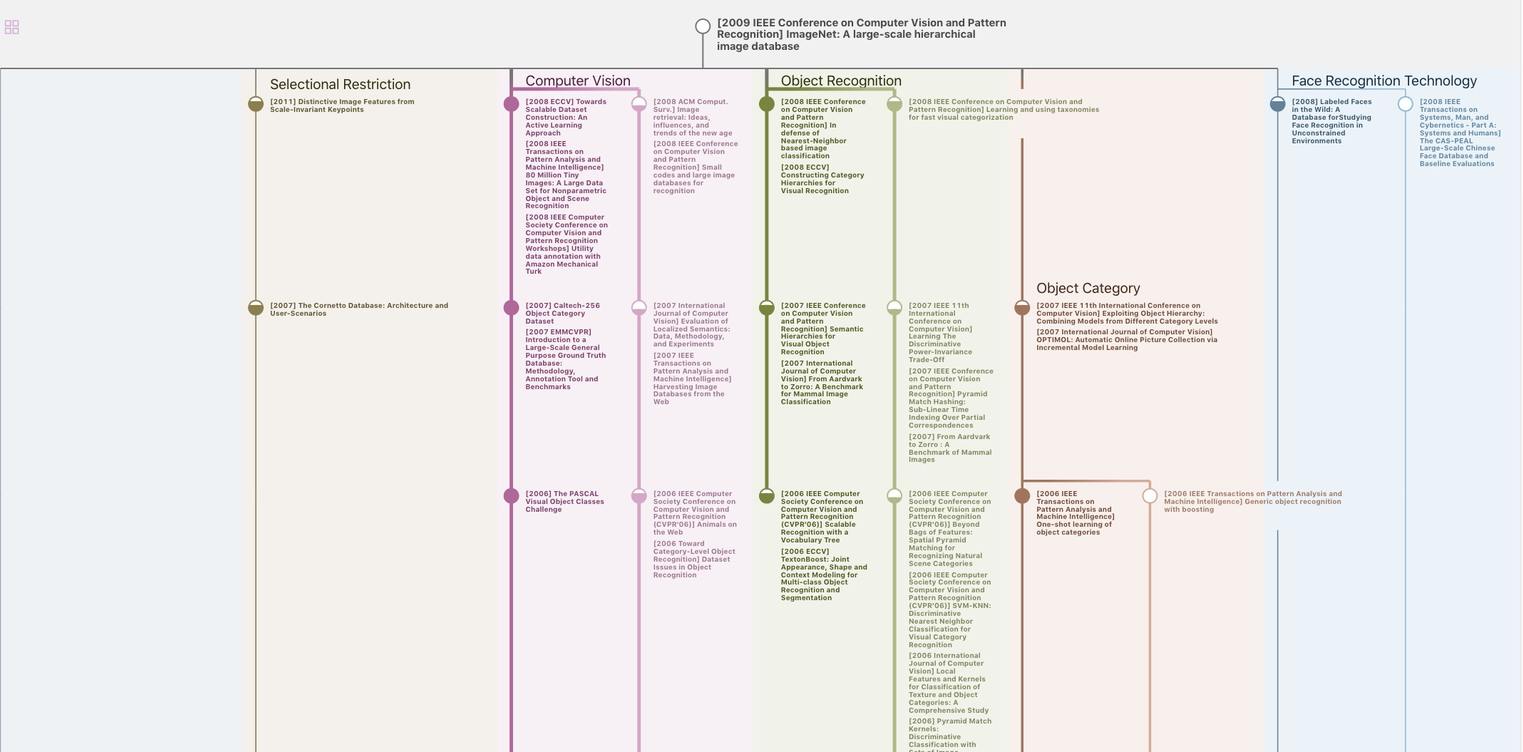
生成溯源树,研究论文发展脉络
Chat Paper
正在生成论文摘要