Cross-indexing of binary SIFT codes for large-scale image search.
IEEE Transactions on Image Processing(2014)
摘要
In recent years, there has been growing interest in mapping visual features into compact binary codes for applications on large-scale image collections. Encoding high-dimensional data as compact binary codes reduces the memory cost for storage. Besides, it benefits the computational efficiency since the computation of similarity can be efficiently measured by Hamming distance. In this paper, we propose a novel flexible scale invariant feature transform (SIFT) binarization (FSB) algorithm for large-scale image search. The FSB algorithm explores the magnitude patterns of SIFT descriptor. It is unsupervised and the generated binary codes are demonstrated to be dispreserving. Besides, we propose a new searching strategy to find target features based on the cross-indexing in the binary SIFT space and original SIFT space. We evaluate our approach on two publicly released data sets. The experiments on large-scale partial duplicate image retrieval system demonstrate the effectiveness and efficiency of the proposed algorithm.
更多查看译文
关键词
large scale,scale invariant feature transform,binary sift codes,sift binarization,indexing,searching strategy,transform coding,dispreserving binary codes,sift descriptor magnitude patterns,image retrieval,cross-indexing,large-scale image search,binary codes,unsupervised algorithm,image search,flexible sift binarization algorithm,cross indexing,large-scale partial duplicate image retrieval system,hamming distance,vectors,feature extraction,visualization
AI 理解论文
溯源树
样例
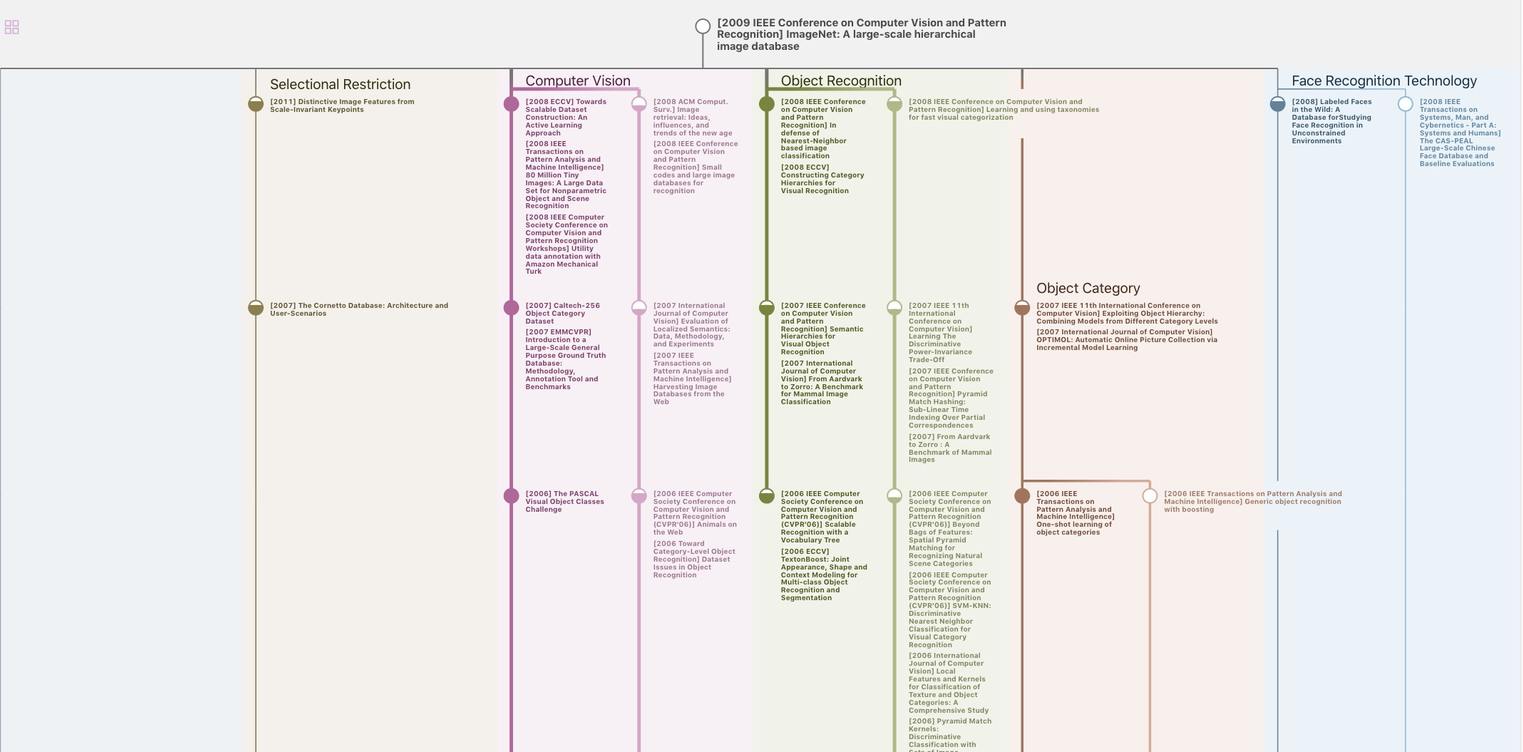
生成溯源树,研究论文发展脉络
Chat Paper
正在生成论文摘要