Predicting changes in hypertension control using electronic health records from a chronic disease management program.
JOURNAL OF THE AMERICAN MEDICAL INFORMATICS ASSOCIATION(2014)
摘要
Objective Common chronic diseases such as hypertension are costly and difficult to manage. Our ultimate goal is to use data from electronic health records to predict the risk and timing of deterioration in hypertension control. Towards this goal, this work predicts the transition points at which hypertension is brought into, as well as pushed out of, control. Method In a cohort of 1294 patients with hypertension enrolled in a chronic disease management program at the Vanderbilt University Medical Center, patients are modeled as an array of features derived from the clinical domain over time, which are distilled into a core set using an information gain criteria regarding their predictive performance. A model for transition point prediction was then computed using a random forest classifier. Results The most predictive features for transitions in hypertension control status included hypertension assessment patterns, comorbid diagnoses, procedures and medication history. The final random forest model achieved a c-statistic of 0.836 (95% CI 0.830 to 0.842) and an accuracy of 0.773 (95% CI 0.766 to 0.780). Conclusions This study achieved accurate prediction of transition points of hypertension control status, an important first step in the long-term goal of developing personalized hypertension management plans.
更多查看译文
关键词
hypertension control,predictive modeling,visualization
AI 理解论文
溯源树
样例
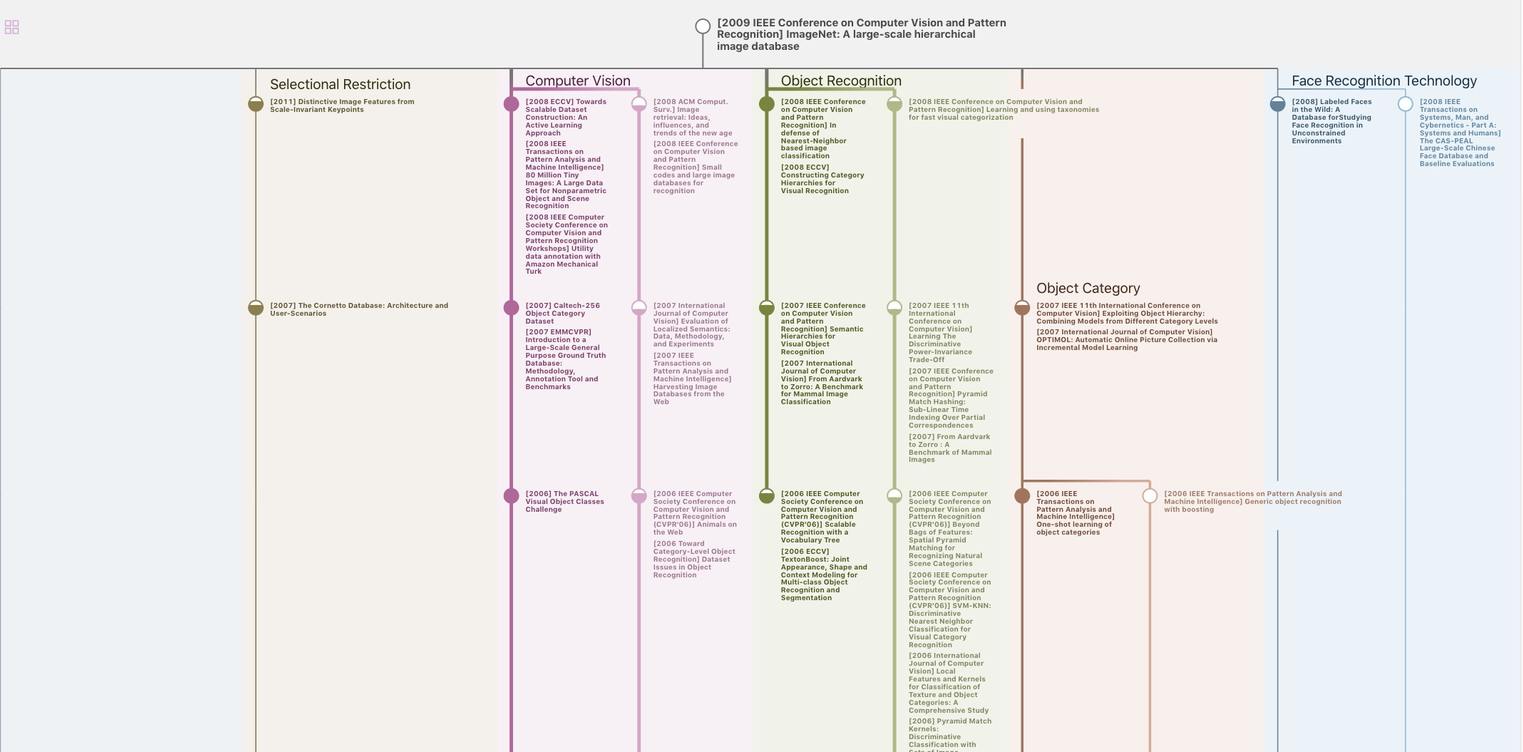
生成溯源树,研究论文发展脉络
Chat Paper
正在生成论文摘要